Image Analytics Tools: Their Role in Modern Research
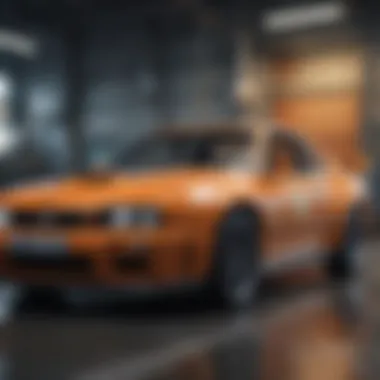
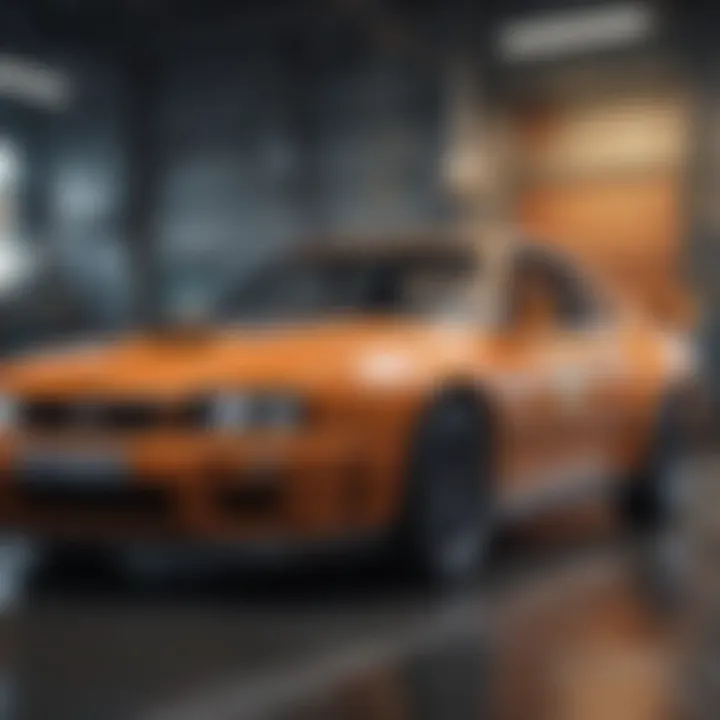
Intro
In the contemporary landscape of research, image analytics tools have emerged as a powerful ally for scientists, researchers, and academics. As technology advances, the ability to analyze visual data has become crucial. These tools allow for deeper insights and more informed decisions based on images across various disciplines. This introductory section will provide a foundational understanding of what image analytics entails and why its significance cannot be overstated.
The use of images in research is not new, but the methods for analyzing these images have evolved dramatically. Early practices were limited by the lack of sophisticated tools, often relying on manual interpretation. Today, a variety of image analytics tools facilitate high-level processing and analysis, integrating machine learning and artificial intelligence to identify patterns, anomalies, and valuable data points within visual materials.
As the quantity of data produced from images grows, the need for more efficient and accurate analysis becomes essential. Fields such as medicine, biology, environmental science, and social sciences greatly benefit from advancements in image analytics. An understanding of these tools enables researchers to enhance their methodologies and lead to breakthroughs that were previously unattainable.
By diving into the methodologies, applications, and the current challenges faced in this domain, the following sections aim to illuminate the transformative potential of image analytics in modern research.
Understanding Image Analytics Tools
Understanding image analytics tools is crucial for comprehending their impact on modern research. These tools enhance the capability of researchers to analyze and interpret visual data, which is increasingly important in various fields, including healthcare, environmental science, and marketing. The significance of image analytics lies in its ability to convert raw images into actionable insights. This process enables better decision-making and supports innovation.
Definition and Scope
Image analytics tools refer to software and methodologies designed to evaluate visual data. These tools utilize advanced algorithms to process images, extract features, and identify patterns. The scope of image analytics encompasses a wide range of functionalities, from basic image processing to sophisticated analysis leveraging machine learning and deep learning.
The definition can be fleshed out further. For instance, image analytics may include tasks such as image segmentation, object detection, and classification. By understanding the scope, researchers can select appropriate tools based on their specific needs and the complexities of the data they are handling.
Historical Development
The journey of image analytics tools can be traced back to early image processing techniques developed in the 1960s. Initial efforts focused on enhancing image quality and addressing noise in photographs. Over the decades, rapid advancements in computing power and algorithmic sophistication fueled the growth of more complex image analysis methods.
By the 1980s and 1990s, the introduction of computer vision laid a foundational framework for more advanced analytics. Today, deep learning frameworks have revolutionized the field, providing tools that can analyze images with unprecedented accuracy and speed. The evolution from simple processing to complex analytical capabilities highlights how image analytics have become integral to modern research.
Core Technologies Behind Image Analytics
The field of image analytics thrives on several core technologies that serve as its backbone. Understanding these technologies is crucial, as they shape the effectiveness and application of image analytics tools in diverse research environments. Each core technology brings unique benefits and considerations that contribute to the progress of scientific inquiry, making them indispensable for any modern research initiative.
Computer Vision
Computer vision is a vital component of image analytics. This technology enables systems to interpret and process visual data from the world. By mimicking human sight capabilities, computer vision allows researchers to automate image processing tasks efficiently. Its importance lies in the ability to enhance accuracy in data extraction, recognition, and classification.
- Key benefits include:
- Automation of visual tasks: Reduces the need for manual intervention, leading to increased throughput.
- Precision: Algorithms can identify patterns and anomalies that may go unnoticed by the human eye.
- Real-time analysis: Facilitates immediate data assessment crucial for timely decision-making.
Computer vision finds applications in various fields, particularly in healthcare, where it assists in interpreting medical images for diagnosis and treatment planning.
Machine Learning
Machine learning plays an essential role in image analytics through its capabilities to learn from and adapt to new data. It allows systems to improve automatically from experience without being explicitly programmed. By analyzing large datasets of images, machine learning algorithms can discern complex patterns and provide meaningful insights.
- Important points include:
- Continuous improvement: The more data a system processes, the better it becomes at making predictions.
- Classification capabilities: Machine learning enhances the ability to classify images into different categories, which is useful in sectors like retail and security.
- Personalization: Algorithms can adapt outputs based on user preferences, providing tailored insights.
The integration of machine learning in image analytics tools elevates their functionality, enabling them to evolve and deliver increasingly precise results.
Deep Learning Frameworks
Deep learning frameworks have emerged as a transformative force in image analytics. These frameworks, built on artificial neural networks, contribute significantly to the functionality of modern image analysis tools. Deep learning excels at processing vast quantities of data, making it particularly suited for image recognition tasks that require high levels of accuracy.
- Notable aspects include:
- Layered architecture: Allows the model to learn from data in a hierarchical manner, extracting features at different levels of complexity.
- Increased accuracy: Deep learning models often outperform traditional methods in tasks such as object detection and image segmentation.
- Scalability: Capable of handling larger datasets, deep learning scales efficiently with increased data availability.
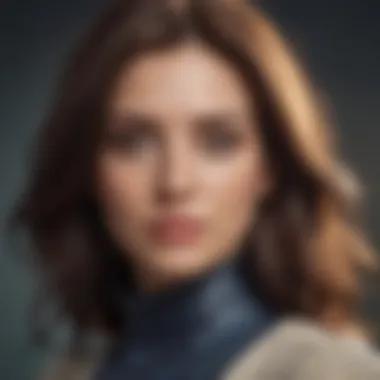
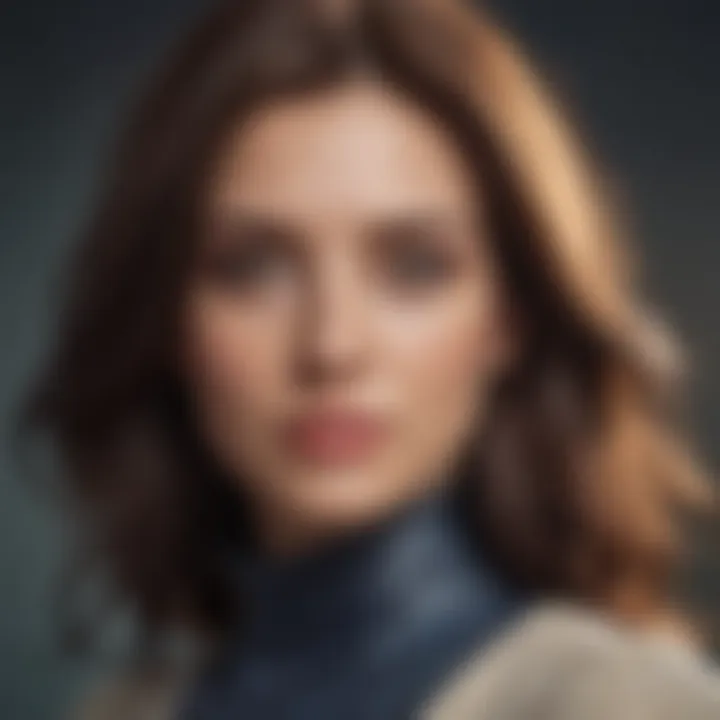
Deep learning frameworks represent the forefront of image analytics technology, creating unparalleled opportunities for advancements in research and industry applications.
"Deep learning frameworks enable machines to achieve tasks that require human-like understanding of images, vastly improving the efficiency of data analysis."
In summary, the core technologies behind image analytics, specifically computer vision, machine learning, and deep learning frameworks, offer substantial advantages to researchers. Each technology provides unique capabilities that enhance data interpretation and facilitate innovation in various sectors. Understanding and leveraging these technologies is key to maximizing the impact of image analytics tools in modern research.
Application Areas of Image Analytics
Image analytics tools are transformative across various sectors, significantly impacting research methodologies and outcomes. Understanding the application areas helps to elucidate their versatility and effectiveness. Fields like biomedical research, environmental studies, manufacturing, and marketing benefit from enhanced data visualization and analysis capabilities. Each application area presents unique advantages, making it essential to explore their specific contributions to contemporary research and practice.
Biomedical Research
In biomedical research, image analytics tools are crucial for diagnosing diseases and monitoring patient health. High-resolution imaging techniques, such as magnetic resonance imaging (MRI) and computed tomography (CT), allow scientists to obtain detailed images of internal structures. Image analysis assists in identifying anomalies that may indicate health issues. Researchers can automate image interpretation, thus improving accuracy and reducing time for diagnosis. Additionally, these tools facilitate quantitative analysis, enabling detailed measurements of tumor size or organ dimensions, which are vital in treatment planning.
Environmental Studies
Environmental studies leverage image analytics tools to monitor ecosystems and assess human impact. Satellite imagery and aerial photography provide vast amounts of data, helping researchers track changes in land use, deforestation, and urbanization. Utilizing image analysis enables the identification of specific trends over time. For instance, monitoring water bodies for pollution levels or detecting heat islands in urban areas becomes more efficient. The insights derived from these analyses support more informed policy-making and resource management.
Manufacturing and Production
Within manufacturing, image analytics plays a significant role in quality control and process optimization. Automated inspection systems utilize high-resolution cameras to detect defects on assembly lines. By applying machine learning techniques to image data, manufacturers can predict failures before they occur, saving costs and time. The ability to analyze production visuals helps in maintaining consistent product quality, which is essential in competitive markets. Additionally, image analytics can aid in monitoring equipment performance, enhancing operational efficiency.
Marketing and Consumer Behavior
In marketing, image analytics tools provide a nuanced understanding of consumer behavior. By analyzing data from social media and digital marketing campaigns, brands can assess public sentiment and preferences. Visual content analytics enables marketers to determine which images or videos attract more engagement. Understanding visual cues in consumer interaction leads to more targeted campaigns, increasing conversion rates. Moreover, retailers use image recognition technologies to analyze shopping patterns, helping them optimize product placement and enhance customer experiences.
Image analytics tools not only improve process efficiency but also offer substantial insights across multiple sectors.
In summary, image analytics tools are integral to biomedical research, environmental studies, manufacturing, and marketing. These areas benefit from enhanced data interpretation and decision-making capabilities. Exploring these applications reveals the significance of image analytics in modern research and its pervasive influence on advancing various fields.
Methods of Image Analysis
In the realm of image analytics, methods of image analysis hold a paramount significance. They form the backbone of how raw image data is interpreted, processed, and converted into actionable insights. Understanding these methods is crucial for professionals who rely on image analytics tools in various disciplines. The methods encompass a range of techniques, each contributing uniquely to the analysis process, ensuring that the information derived from images is both meaningful and practical.
Image Processing Techniques
Image processing techniques involve the manipulation and enhancement of images to make them suitable for analysis. These techniques can help in improving image quality by adjusting brightness, contrast, and sharpness. For example, the use of filters can remove noise from images, thereby revealing clearer features. Additionally, image processing often includes operations such as scaling, cropping, and resizing ensure relevant areas are focused while extraneous elements are minimized.
Key image processing techniques include:
- Histogram Equalization: This process enhances the contrast of images, allowing for better visibility of details.
- Edge Detection: Techniques such as the Sobel or Canny edge detectors highlight boundaries and transitions within an image, important for object recognition.
- Morphological Operations: These techniques assist in processing the geometrical structure of images, useful in fields like biomedical imaging.
These methods are fundamentally important as they enable more accurate analysis, leading to enhanced decision-making processes across various fields of research.
Pattern Recognition Algorithms
Pattern recognition algorithms play a vital role in identifying patterns and features within images. They classify data based on the input images, which allows researchers to unveil trends or anomalies that they may otherwise overlook. This branch of image analysis leverages various mathematical models and machine learning techniques to distinguish between different objects within an image. For instance, convolutional neural networks (CNNs) are widely used for their efficacy in recognizing complex patterns.
Common approaches in pattern recognition include:
- Supervised Learning: Labels are assigned to data, allowing the system to learn from known examples.
- Unsupervised Learning: The system identifies inherent structures or patterns without pre-existing labels.
- Semi-Supervised Learning: This combines elements of both methods, leveraging a small amount of labeled data with a larger amount of unlabeled data.
Pattern recognition algorithms are essential for extracting key information from images. They help transform raw data into structured and insightful knowledge, facilitating diverse applications such as facial recognition, medical diagnosis, and security systems.
Feature Extraction Methods
Feature extraction methods are crucial in reducing the dimensionality of data while retaining its essential characteristics. This process involves selecting and transforming image attributes into a format that algorithms can efficiently analyze. Features such as color, texture, shape, and spatial relationships are commonly extracted to create a robust representation of the image.
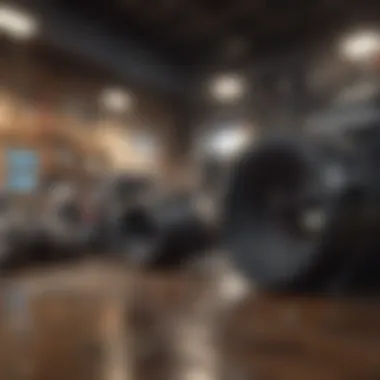
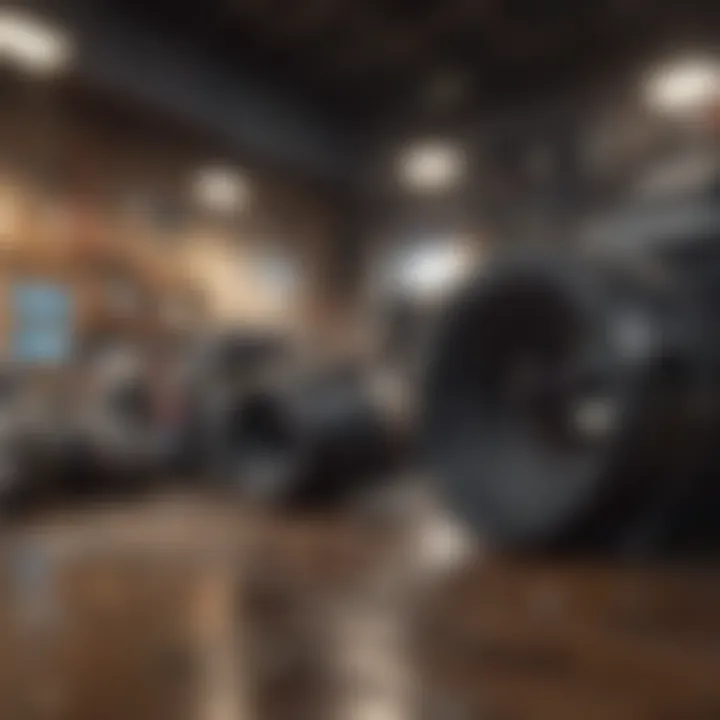
Some common feature extraction techniques include:
- Texture Analysis: Using methods like Local Binary Patterns (LBP) or Gabor filters to quantify the texture of surfaces within an image.
- Color Histograms: Analyzing the distribution of colors within an image, which can help in identifying objects or classifying images based on visual similarities.
- Shape Descriptors: Employing techniques like contour and region-based descriptors to represent object shapes effectively.
Feature extraction is vital as it leads to more efficient processing and higher accuracy in classification tasks. It distills complex data down to relevant features, ensuring that the image analytics tools operate at their highest potential.
Benefits of Using Image Analytics Tools
Image analytics tools have become essential in modern research, as they provide various benefits that enhance the ability to interpret large volumes of imagery data. The importance of these benefits cannot be understated, especially as researchers strive for accuracy and efficiency in their work. Understanding these advantages is integral for students, educators, and professionals looking to leverage image analytics effectively.
Enhanced Data Insights
One of the primary advantages of image analytics tools is the ability to derive enhanced insights from visual data. These tools transform raw imagery into valuable information, enabling researchers to uncover patterns and trends that may not be immediately visible to the human eye. For example, in the field of biomedical research, image analytics can identify anomalies in medical imaging data, leading to early detection of diseases.
Moreover, the incorporation of artificial intelligence in these tools allows for a more profound analysis. Machine learning algorithms can learn from vast datasets, ensuring that the insights produced are both relevant and actionable. This ability to analyze complex data sets facilitates a more informed understanding of the resource being studied.
"Through the application of image analytics, researchers are not just viewing images but interpreting them with context and precision. This leads to deeper understanding and innovative insights."
Improved Decision Making
Another significant benefit of image analytics tools is their contribution to improved decision-making processes. By providing data-driven insights, these tools empower researchers to make more informed choices based on visual evidence.
For instance, in agricultural monitoring, image analytics can help farmers assess crop health more accurately. This capability enables them to make strategic decisions regarding fertilizer application, pest control, and irrigation practices. Additionally, by presenting data in a user-friendly format, these tools facilitate clearer communication among stakeholders involved in a project, leading to more cohesive strategy formulation.
The reliance on objective data rather than subjective observations minimizes the risk of bias in decision making. In today's fast-paced research environment, such clarity is crucial for successful outcomes.
Time and Cost Efficiency
Lastly, image analytics tools significantly enhance time and cost efficiency within research frameworks. Automating image processing and analysis reduces the need for manual intervention, which can often be cumbersome and prone to errors. In sectors such as manufacturing, where precision is key, image analytics tools can quickly identify defects in production lines, potentially saving companies considerable amounts of money.
Furthermore, the accuracy achieved through image analysis leads to reduced wastage and better resource management. By processing and analyzing images rapidly, researchers can focus their efforts on high-value tasks, skipping laborious processes that do not add much to the outcome.
In summary, the benefits of using image analytics tools extend beyond improved research outcomes. These tools transform the way researchers draw conclusions, make decisions, and manage resources, establishing a foundation for innovation and advancement in multiple fields.
Challenges in Image Analytics
The field of image analytics presents notable challenges that must be addressed for successful implementation. Understanding these challenges is crucial for optimizing image analytics tools. Key elements include data quality issues, integration with existing systems, and scalability concerns. Each aspect critically impacts the effectiveness of image analytics in various sectors, highlighting the need for caution and thorough planning.
Data Quality Issues
Data quality is a fundamental concern in image analytics. Poor quality images can significantly affect the outcomes of analysis. Factors such as image resolution, noise, and artifacts can lead to misinterpretations. For example, biomedical imaging requires high precision. A distorted image may lead to incorrect diagnoses. To counter these issues, organizations must invest in high-quality imaging equipment and robust preprocessing techniques.
Unstandardized data formats are another challenge. Integrating images from different sources may result in inconsistencies which complicate data analysis. Employing strict quality control measures throughout the image acquisition process can alleviate these issues to some extent.
Integration with Existing Systems
Another major challenge lies in integrating image analytics tools with existing systems. Many organizations operate on legacy systems that may not support the latest technologies. This situation can hinder the seamless flow of data needed for accurate analysis. The integration process can be time-consuming and costly. Compatibility between new image analytics tools and legacy software is often limited. Organizations must perform a careful evaluation of current systems to mitigate disruptions.
The use of APIs can facilitate better integration, allowing for more fluid interaction between disparate systems. Ensuring that all systems can communicate effectively is paramount for leveraging the full potential of image analytics tools.
Scalability Concerns
Scalability is also a critical issue in image analytics. As the volume of data grows, systems need to scale accordingly. The process can be complex and requires careful planning. If analytics tools do not scale well, they may become a bottleneck in the research workflow.
Organizations must design their image analytics framework with future growth in mind. This can include cloud-based solutions that offer flexible storage and processing power. The ability to manage increasing data loads without loss of performance is essential in maintaining the usefulness of image analytics in research and industry.
Case Studies in Image Analytics
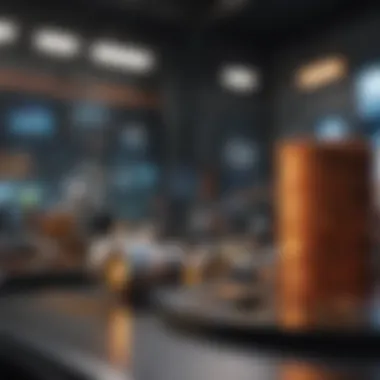
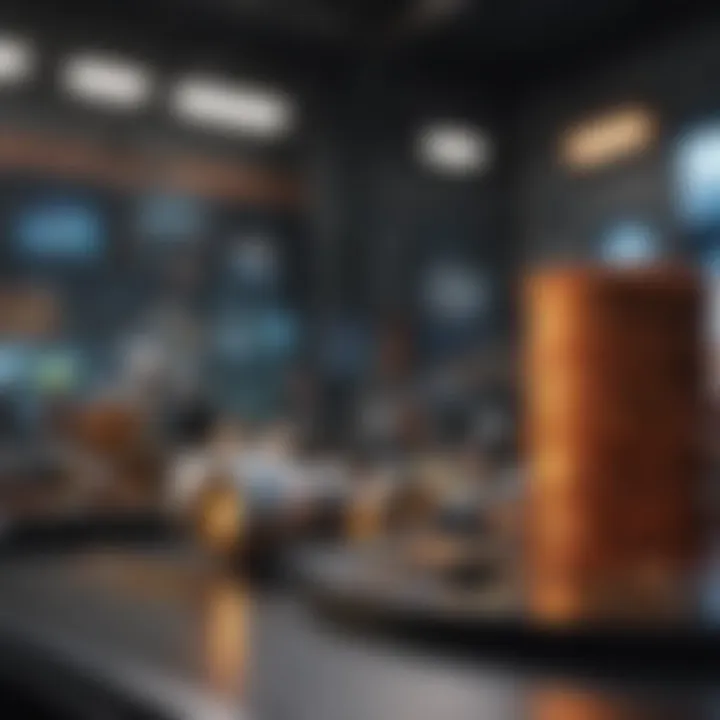
Case studies in image analytics demonstrate the practical applications and effectiveness of these tools in various sectors. Analyzing specific scenarios elucidates not only the benefits but also the ongoing challenges that practitioners face. By focusing on real-world insights, this section underscores the significance of image analytics in solving complex problems and improving outcomes in diverse fields.
Healthcare Applications
In healthcare, image analytics tools play a pivotal role. They enhance diagnostic processes by allowing for more precise interpretation of medical images. Tools such as MRI and CT scanners can now benefit from advanced algorithms that assist in identifying anomalies. For instance, studies have shown that machine learning can help in detecting early signs of diseases like cancer more accurately than traditional methods.
The integration of image analytics enhances patient outcomes significantly. It also facilitates personalized medicine by allowing tailored treatment plans based on detailed imaging data. Furthermore, tools like the ImageJ software enable researchers to quantitatively analyze medical images, streamlining the research aspect of healthcare.
Agricultural Monitoring
Agricultural monitoring is another field where image analytics is gaining momentum. Drones equipped with imaging technology provide farmers with insights into crop health. By employing multispectral and hyperspectral imaging, farmers can assess vital data such as soil moisture levels and plant vitality. This data-driven approach facilitates informed decision-making about irrigation and fertilization.
Real-time analytics enhances yield prediction and pest management strategies. Tools like Sentera and DroneDeploy allow for immediate feedback on crop status. This not only maximizes productivity but also contributes to sustainability practices within farming.
Urban Planning Initiatives
Urban planning is being transformed through image analytics as well. Planners leverage satellite imagery and aerial footage to scrutinize urban growth patterns. This information enables better infrastructure development and resource allocation. For instance, GIS (Geographic Information Systems) integrates image analytics to visualize urban environments effectively.
Case studies show that cities utilizing image analytics tools can assess traffic patterns and optimize public transportation systems. They also provide valuable insights into environmental impacts, such as urban heat islands. This capability allows urban planners to make data-informed decisions, thereby fostering smart city initiatives.
"Image analytics has become indispensable in contemporary research, providing clarity and direction in complex environments."
By examining these case studies, it becomes evident how vital image analytics tools are across different sectors. Each case brings its own challenges and lessons learned, allowing for continuous improvement and innovation in these technologies.
Future Directions in Image Analytics
As we advance into an era driven by data, the future of image analytics stands as a pillar for innovation in numerous sectors. This segment emphasizes the transformative potential of emerging technologies and the pivotal role they will play in determining how researchers interact with images and data. By focusing on advancements in artificial intelligence, new trends in data utilization, and ethical considerations, we can understand how to leverage these tools effectively.
Advancements in AI Technologies
The integration of AI technologies into image analytics is rapidly evolving. Innovations in algorithms and computational methods are enhancing the capability of image analysis tools. Machine learning models now demonstrate the ability to learn from vast datasets, improving accuracy in tasks such as segmentation, classification, and object detection.
Among notable advancements is the development of convolutional neural networks (CNNs) that specialize in extracting features from images, making processes faster and more efficient. Transfer learning, which allows models to apply knowledge gained from a specific task to another domain, is also on the rise. This requires less data for training while still providing reliable results. In industries such as healthcare, these advancements mean quicker diagnosis and better patient outcomes through enhanced image interpretation.
Emerging Trends in Data Utilization
Recent trends show a shift towards more sophisticated ways of utilizing data from image analytics. Big data is becoming integral, driving the need for tools that can analyze vast quantities of images efficiently. As cloud computing technologies advance, researchers can access powerful resources for storage and analysis.
Besides, the use of real-time data processing is gaining traction. This allows for immediate feedback and decision-making, especially crucial in high-stakes environments like emergency healthcare and security surveillance. Moreover, data visualization techniques are evolving, transforming complex data sets into comprehensible formats. This makes it easier for non-technical stakeholders to interpret findings, bridging gaps between technical and non-technical fields.
Ethical Considerations in Image Analysis
While the future holds promise, ethical considerations cannot be overlooked. As image analytics tools become more pervasive, concerns regarding privacy and data security are paramount. Researchers must grapple with the responsibilities tied to the use of sensitive images, especially in fields such as healthcare and law enforcement. Safeguards must be established to protect anonymity and ensure that data is used responsibly.
Additionally, biases in AI algorithms are an area of concern. If the training data lacks diversity, the resultant model may reinforce stereotypes or make inaccurate predictions. Continuous evaluation and adjustment of datasets are necessary to mitigate these biases. Engaging interdisciplinary teams can help address these ethical dilemmas, leading to a more balanced and fair data analysis process.
"The continued evolution of image analytics will redefine benchmarks in research, provided that ethical considerations are interwoven with technological advancements."
The End
The conclusion of this article emphasizes the significance of image analytics tools in modern research. These tools are not just instruments for analysis; they represent a transformative leap in how researchers interpret visual data across various fields. Recognizing the multifaceted capabilities of image analytics can help drive innovation and productivity in research initiatives.
Summation of Key Insights
Image analytics tools enhance the ability to extract meaningful insights from vast amounts of visual data. Their applications range from biomedical research to environmental studies, showcasing a broad spectrum of utility. Key insights include the enhancement of data quality, facilitating more informed decision-making. By utilizing advanced technologies such as machine learning and deep learning frameworks, researchers can identify patterns and features that were previously difficult to discern. In summary:
- Diverse Applications: Applicable across multiple fields, including healthcare and agriculture.
- Technological Innovations: Continuous advancements in AI are enhancing the capabilities of image analytics.
- Quality and Efficiency: Improved data insights lead to better decision making and operational efficiency.
"The future of image analytics is intricately tied to advancements in artificial intelligence and the ethical implications of data use."
Final Thoughts on Future Impact
The future impact of image analytics in research is poised to be profound. As these tools evolve, they will enable more sophisticated analysis of visual data, offering researchers deeper insights than ever before. Ethical considerations surrounding data use will become increasingly important as these technologies are more integrated into everyday research practices. Hence, it is crucial for professionals in the field to stay informed about both technological advancements and ethical guidelines.