Understanding Digital Twin Technology and Its Impact
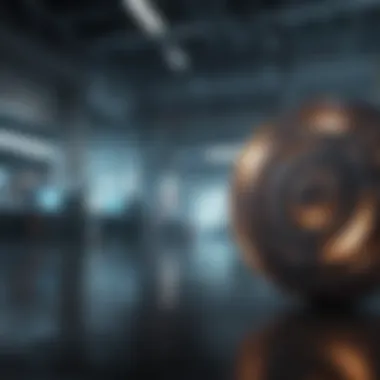
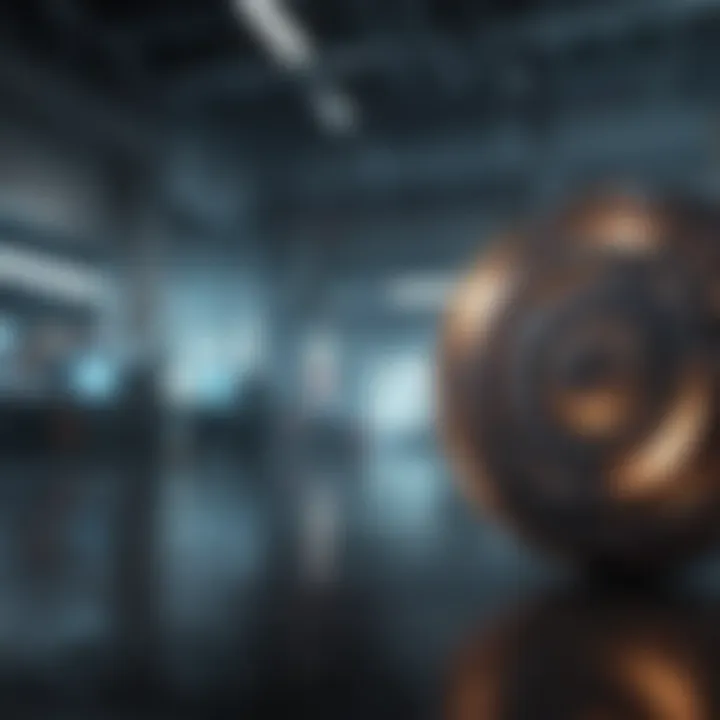
Intro
Digital twin technology has emerged as a pivotal concept in the realms of engineering, simulation, and system optimization. It refers to the creation of a virtual replica of physical systems, which can be utilized for modeling, analyzing, and enhancing real-world operations. The continued evolution of digital twin technology holds immense potential across various sectors, including manufacturing, healthcare, and urban planning. The significance of understanding this domain lies in its application towards improving efficiency, reducing costs, and fostering innovation.
Overview of Research Topic
Brief Background and Context
The idea of a digital twin dates back over a decade, gaining traction primarily from advancements in the Internet of Things (IoT) and data analytics technologies. As organizations increasingly embrace digital transformation, the integration of physical systems with their virtual counterparts becomes vital. The digital twin acts as a conduit for data, enabling real-time insights that inform decision-making processes.
Importance in Current Scientific Landscape
In today’s rapidly evolving technological environment, digital twin technology is critical for research and practical applications. The capacity to simulate different scenarios provides researchers and practitioners with invaluable insights. For instance, in manufacturing, digital twins can optimize production lines, enhancing operational performance and asset management. In healthcare, they empower personalized medicine and improve patient outcomes. Understanding this technology is essential for students, researchers, and industry professionals aiming to remain competitive in their fields.
Methodology
Research Design and Approach
To grasp the intricacies of digital twin technology, a qualitative research approach is adopted. This includes extensive literature reviews, case studies, and interviews with industry experts. This design helps to uncover diverse perspectives and comprehensive insights regarding functionality and applications.
Data Collection Techniques
Collecting data involves various techniques such as:
- Surveys: Gathering information from industry participants about their experiences with digital twins.
- Case Studies: Analyzing successful implementations across different sectors to understand best practices.
- Expert Interviews: Engaging with thought leaders to gain deeper insights into challenges and future developments.
The digital twin is not just a model; it is a comprehensive system that learns and evolves over time.
Definition of Digital Twin
Digital twin technology is gaining significant attention in various sectors for its ability to create a digital counterpart to physical entities. Understanding the definition of a digital twin allows professionals to appreciate its importance in enhancing operational efficiency and innovation. A digital twin is not just a static model; it is an active digital replica of physical objects, processes, or systems. This replication incorporates real-time data and analytics, which leads to better decision-making.
The digital twin concept facilitates the simulation of real-world conditions in a virtual environment, enabling organizations to test scenarios without risks. This aspect becomes especially crucial in industries like manufacturing or healthcare, where implementing changes can be costly and intricate.
Origins and Evolution
The idea of a digital twin has its roots in the field of product lifecycle management. The term "digital twin" first emerged in the manufacturing industry around 2002, presented by Michael Grieves in a talk about product lifecycle management. Since then, the concept has evolved significantly, driven by advancements in information technology and data analytics.
Initially, digital twins were primarily used for engineering purposes, focusing on design and manufacturing processes. Over the years, the application of this technology has expanded into sectors such as aerospace, automotive, and even urban planning, indicating a broader relevance beyond traditional boundaries. The evolution has been largely supported by improvements in sensor technology, which enable accurate data capture and real-time feedback, transforming the digital twin from a conceptual model into practical tools that inform strategic decisions.
Core Concept Explained
At its core, a digital twin functions by integrating virtual and physical realities. This partnership is established through sensors embedded in physical systems which collect data on performance and operations. The digital twin uses this information to simulate what is happening in the real world.
Factors like machine learning and analytics play a crucial role. They allow digital twins to analyze vast amounts of information, providing insights that can lead to predictive maintenance and improved resource management. The model grows sophisticated over time as it learns from new data inputs. Notably, this real-time aspect allows organizations to not only visualize their operations but also forecast potential issues and opportunities, ultimately leading to enhanced performance and cost savings.
In summary, understanding the definition and core concepts of digital twin technology is essential for leveraging it effectively in any industry. Its origins trace back to product management, while its current applications extend widely across various sectors. This understanding provides a foundation for comprehending its complex components and broader implications in today's digital landscape.
Components of a Digital Twin
The concept of a digital twin hinges not just on its definition but also on the components that form its underlying structure. Understanding these components is crucial, as they collectively enable the functionalities that define a digital twin. The amalgamation of physical entities, virtual models, and robust data integration collectively enhance the capabilities of a digital twin, driving efficiency and innovation across various sectors.
Physical Entity
The physical entity is the tangible counterpart in the digital twin framework. This can be anything from a complex machine in an industrial setting to a building or even a city. The integrity and accuracy of the digital twin heavily depend on the features and operational capacities of this physical entity. If the real-world object changes—be it through wear, upgrades, or damage—the digital representation must also reflect these modifications. Updates are essential; otherwise, the digital model becomes obsolete, offering misleading data. This connection is often facilitated through a multitude of sensors that continuously monitor and relay information about the physical entity’s performance and state.
Virtual Model
The virtual model serves as the core of the digital twin. It is a sophisticated simulation that replicates the physical entity's behavior, characteristics, and interactions. This model is designed using computational algorithms and mathematical frameworks that mirror the real-world functionalities. The accuracy of the virtual model is essential for reliable predictions and insights. It allows users to analyze "what-if" scenarios, facilitating better decision-making processes. Such dynamic simulations can reveal important patterns that can optimize operations and preemptively identify potential issues. This adaptability makes the virtual model a powerful tool in development, testing, and optimization in various fields like engineering and healthcare.
Data Integration
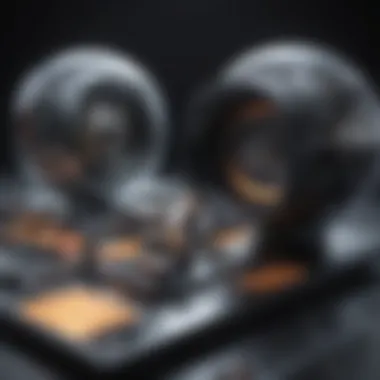
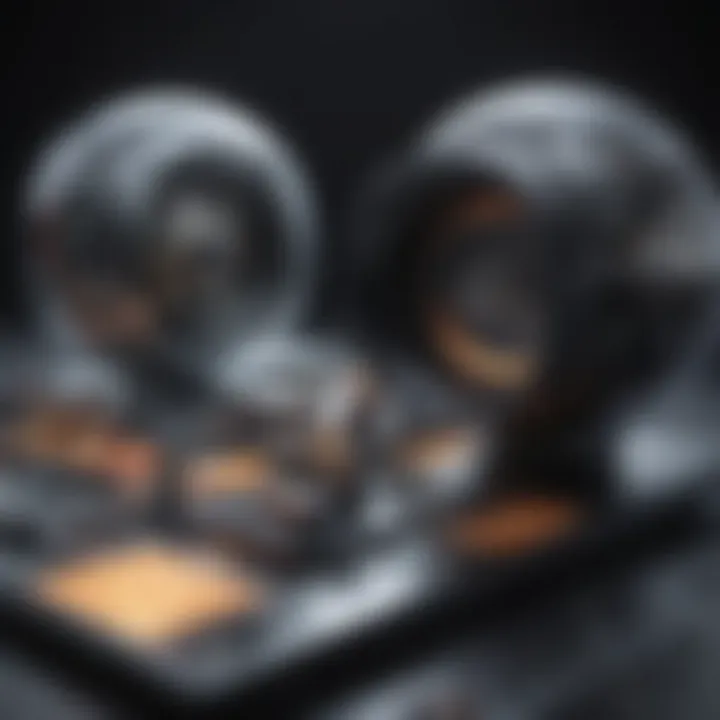
Data integration forms the backbone of effective digital twin technology. It encompasses the aggregation of data from various sources including sensors installed on the physical entity, external databases, and other real-time inputs. The ability to collect, process, and analyze large sets of data allows digital twins to function optimally. The real-time processing of this integrated data enables continuous monitoring and accurate representation of the physical counterpart, enhancing responsiveness and adaptability. Furthermore, data integration can sometimes raise challenges related to interoperability of systems and data privacy, but overcoming these issues opens new avenues for more advanced analytics and insights.
Effective data integration is vital for not only the performance of the digital twin but also its broader applications across industries.
In summary, the components of a digital twin—a physical entity, a virtual model, and data integration—are interdependent elements that together create a comprehensive representation of an object or system. Their synergy allows for real-time insights and optimizations, making digital twins invaluable across many sectors.
Technological Framework
Digital twin technology relies on a robust technological framework that enables the integration and functioning of various components. This framework comprises several critical elements, each contributing to the effective creation and utilization of digital twins. A strong technological framework is essential for optimizing the application of digital twin technology across diverse industries.
IoT and Sensor Technology
The Internet of Things (IoT) serves as a crucial foundation for digital twin technology. With a network of interconnected devices, IoT facilitates the real-time collection and transmission of data from physical entities to their digital counterparts. Sensors are at the heart of this process, capturing data from various sources such as machinery, vehicles, and environmental conditions.
By employing IoT and sensor technology, organizations can gain insights into operational performance and condition monitoring. This data flow enables accurate replication of physical assets in a virtual environment. Moreover, having real-time feedback allows for timely decision-making, enhancing operational efficiency.
Artificial Intelligence
Artificial Intelligence (AI) enhances digital twin technology by enabling advanced analytics and predictive modeling. With AI, digital twins can analyze historical data and forecast future performance or failures. This is particularly valuable for predictive maintenance, where potential issues are identified before they lead to costly downtimes.
The integration of AI algorithms enables automated learning and improvement of the digital twin's accuracy. By continuously learning from new data, AI makes the virtual twin more reliable and more reflective of the actual system. Thus, organizations can make informed decisions based on accurate simulations.
Cloud Computing
Cloud computing plays a significant role in enabling digital twin technology. Through cloud platforms, organizations can store vast amounts of data generated by sensors and IoT devices. These platforms provide the computational power necessary for processing this data and running complex simulations.
Furthermore, cloud computing allows for scalability in managing digital twins. As businesses grow or technology evolves, they can easily adjust their digital twin configurations and processing capabilities in the cloud without significant infrastructure investment. This flexible environment fosters innovation and maximizes the potential of digital twin applications.
"A proper technological framework is the backbone of efficient digital twin implementation, driving performance and enabling innovation across sectors."
In summary, the technological framework behind digital twin technology is built on the integration of IoT, AI, and cloud computing. These elements work in concert to provide real-time data, advanced analytics, and scalable solutions, allowing organizations to optimize their operations and embrace the full potential of digital twins.
Applications of Digital Twin Technology
Applications of digital twin technology illustrate its transformative potential across various sectors. By creating a virtual replica of a physical entity, organizations can simulate behaviors, monitor operations, and optimize processes. The analysis of these applications reveals crucial benefits and considerations that enhance efficiency and strategic planning.
Manufacturing and Engineering
In the manufacturing and engineering sectors, digital twins are pivotal for enhancing production processes. These technologies allow companies to create real-time simulations of their manufacturing systems. By monitoring the performance using sensor data, manufacturers can gain insights into equipment efficiency, production rates, and potential bottlenecks.
Benefits include:
- Predictive analysis: Utilizing machine learning algorithms to predict equipment failures enables timely maintenance.
- Design validation: Engineers can test design changes in a virtual environment before implementing them physically, saving resources.
- Process optimization: Adjustments can be made quickly in the virtual model, ensuring the physical process runs more smoothly.
Healthcare
The healthcare industry also benefits significantly from digital twin technology. By constructing digital representations of patients, clinicians can personalize treatment plans based on the unique characteristics of an individual's anatomy and medical history.
Key aspects are:
- Real-time monitoring: Patient data can be gathered continuously, allowing healthcare professionals to respond to changes in a patient's condition swiftly.
- Surgical planning: Surgeons are able to simulate procedures on the digital twin before actual operations, minimizing risks.
- Drug development: Pharmaceutical companies can model the impacts of new drugs on virtual patients, expediting clinical trials.
Smart Cities
Digital twin technology plays a significant role in the development of smart cities. By creating a virtual model of a city, planners and operators can test different urban strategies or changes in real-time.
Considerations include:
- Urban infrastructure management: By analyzing traffic flows and resource distribution, cities can improve service delivery and reduce waste.
- Disaster management: Simulating scenarios such as floods or earthquakes helps enhance city resilience and response strategies.
- Energy efficiency: Municipalities can assess energy usage patterns and optimize responses to reduce costs and improve sustainability.
Transportation and Logistics
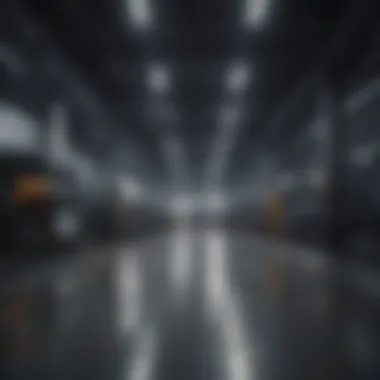
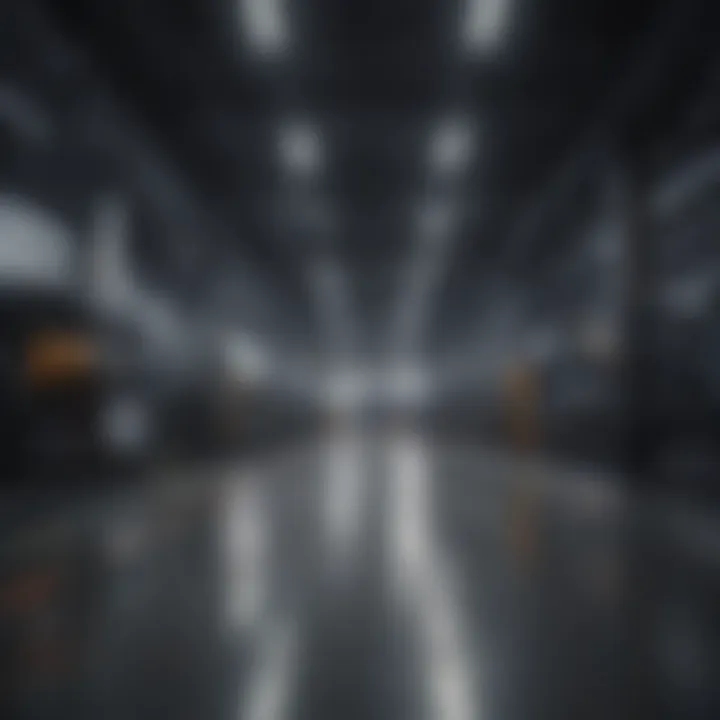
Lastly, the transportation and logistics sectors leverage digital twin technology to enhance operations and efficiency. Virtual models of vehicles, fleets, or entire logistics networks enable detailed analysis and monitoring.
Important factors are:
- Route optimization: By modeling different logistics pathways, companies can identify the most efficient routes and reduce delivery times.
- Fleet management: Real-time tracking of vehicles using digital twins helps managers optimize vehicle usage and maintenance scheduling.
- Supply chain resilience: Organizations can simulate disruptions in the supply chain and develop contingency plans to mitigate risks.
"Digital twins provide a unique opportunity to improve real-world performance by leveraging virtual environments for testing and analysis."
Benefits of Implementing Digital Twins
Digital twin technology offers numerous advantages across various industries. The significance of these benefits cannot be overstated. As organizations increasingly adopt digital twins, they unlock opportunities for enhanced operational efficiency, real-time monitoring, and informed decision-making. Here, we will explore the specific benefits that help elucidate the power of digital twin technology.
Enhanced Monitoring and Diagnostics
One of the primary advantages of digital twins is the ability to significantly improve monitoring and diagnostics. By creating a virtual replica of a physical object or system, organizations can gather data in real time. This data provides insights into the condition and performance of assets. Using advanced analytics, companies can detect anomalies early. For instance, if a machine's temperature rises beyond a certain threshold, the digital twin can trigger alerts. Companies like Siemens have employed such strategies to ensure that machinery operates within optimal parameters.
"Real-time monitoring enables firms to move from reactive to proactive maintenance, enhancing the reliability of their operations."
Predictive Maintenance
Predictive maintenance is closely tied to enhanced monitoring. Understanding how a system behaves allows organizations to anticipate failures before they occur. With the help of machine learning algorithms, digital twins can analyze historical data and identify patterns. This foresight enables maintenance teams to address potential issues prior to equipment failures. By minimizing unplanned downtime, businesses can save on repair costs and prolong the lifespan of their equipment. Businesses in the aviation industry, such as Boeing, utilize predictive maintenance to ensure flight safety and operational efficiency.
Performance Optimization
Another key benefit is performance optimization. Digital twins allow organizations to simulate changes in processes or equipment configurations without disrupting real-time operations. By experimenting with design alterations in the virtual space, companies can identify the best solutions. This capability helps in fine-tuning operations for better productivity. For example, manufacturers can run what-if scenarios to optimize production lines. This approach not only enhances performance but can also lead to improved product quality.
Cost Efficiency
Finally, implementing digital twins can contribute to considerable cost savings. Organizations can significantly reduce operational costs by making informed decisions based on the insights derived from digital twin analyses. Streamlining processes leads to lower resource consumption, whether that be energy, time, or materials. Additionally, the reduced need for physical prototypes during the design phase further saves costs. As a result, the investment in digital twin technology is often offset by the savings generated from improved efficiency and reduced maintenance expenses.
In summary, the benefits of implementing digital twins are profound and multifaceted. Enhanced monitoring and diagnostics facilitate proactive approaches, while predictive maintenance reduces unexpected failures. Performance optimization enables efficient operations, and cost efficiency contributes to healthier bottom lines. These factors make digital twin technology not only innovative but essential for modern businesses.
Challenges in Digital Twin Implementation
Digital twin implementation offers numerous benefits, yet it comes with specific challenges that organizations must confront. Understanding these challenges is crucial, as they can hinder the successful deployment and operation of digital twin solutions. Addressing these issues can enhance the efficiency and effectiveness of digital twin applications, making their advantages more accessible and sustainable for various industries.
Data Security and Privacy Concerns
Data security is a significant concern when implementing digital twin technology. As digital twins rely on massive amounts of real-time data, the potential for sensitive information to be compromised increases. Organizations must prioritize data protection measures to safeguard critical information from cyber threats.
The integration of Internet of Things (IoT) devices increases the volume of data flowing into digital twin systems. This often includes personal data that can lead to privacy violations if not handled properly. Companies must comply with regulations such as GDPR, ensuring that user information remains confidential and is collected and stored ethically. Developing robust encryption methods, implementing access controls, and conducting regular security audits are steps organizations can take to enhance data security and address privacy concerns.
Scalability Issues
Scalability presents a challenge in the digital twin framework. As businesses grow and operations expand, they require a digital twin to adapt to increased complexity. Ensuring that the digital twin can scale to accommodate additional data sources and systems is vital.
Organizations may face difficulties in scaling their digital twin solutions effectively. Performance bottlenecks can arise if the initial architecture cannot handle additional loads. Hence, preemptive planning during the development phase becomes essential to ensure that scalability is built into the digital twin from the outset. This may include choosing cloud solutions that offer flexible scaling capabilities, optimizing algorithms, and leveraging distributed computing environments to manage an expanding data set.
Integration with Existing Systems
Integrating a digital twin with existing systems can also be a complex task. Many organizations utilize legacy systems and older technologies that may not seamlessly communicate with modern digital twin solutions. This lack of compatibility can lead to operational inefficiencies.
An effective integration strategy is necessary to bridge the gap between legacy and new systems. Organizations should consider developing middleware solutions that facilitate communication between various systems. Conducting thorough assessments of both existing and planned systems helps identify potential compatibility issues early in the implementation process. Additionally, fostering collaboration between IT and operational departments can lead to more successful integration outcomes.
"Tackling the challenges in digital twin implementation demands a strategic approach that not only addresses technical hurdles but also incorporates security and privacy safeguards to build trust in these technologies."
In summary, implementing digital twins presents obstacles relating to data security, scalability, and system integration. Addressing these challenges is crucial for organizations aiming to leverage digital twin technology effectively. Only by overcoming these hurdles can they fully unlock the transformative potential of digital twins.
Future Prospects of Digital Twin Technology
Digital twin technology holds immense potential for transforming various sectors. Its future prospects are shaped by several key factors, including advancements in artificial intelligence, the emergence of new industries, and evolving regulatory frameworks. Understanding these elements is essential because it provides insights into how digital twins can further optimize processes, enhance decision-making, and improve outcomes across different applications.
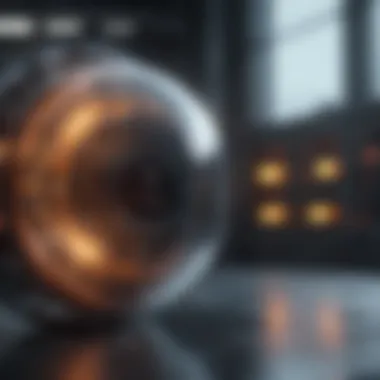
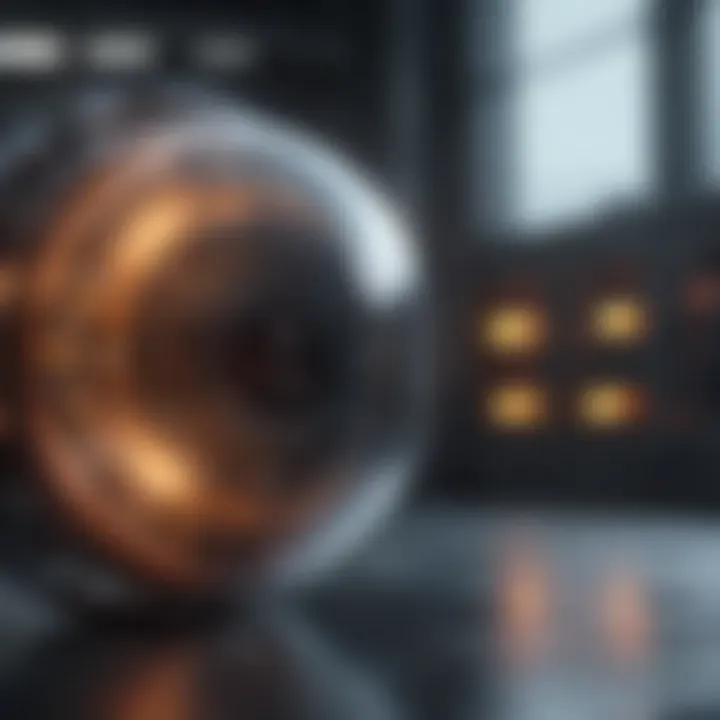
Advancements in AI and Machine Learning
Artificial intelligence and machine learning are pivotal in the evolution of digital twin technology. As algorithms become increasingly sophisticated, digital twins can analyze vast amounts of data in real time. This capability allows for the simulation of countless scenarios, which helps in decision-making. For instance, in manufacturing, AI-driven digital twins can predict failures or inefficiencies before they occur, leading to proactive measures rather than reactive fixes. Moreover, these advancements enable adaptive learning, which means the digital twin becomes more accurate over time as it learns from data patterns.
Key developments include:
- Predictive analytics: Leveraging historical data for anticipating future trends.
- More accurate modeling: AI helps refine the models that digital twins are based on, ensuring better representation of the physical asset.
- Real-time insights: Continuous learning allows for immediate adjustments in operations, further increasing efficiency.
Emerging Industries
Digital twin technology is beginning to pervade various sectors beyond its traditional applications in manufacturing and healthcare. Industries such as agriculture, retail, and energy are increasingly recognizing the benefits of this technology. For example, in agriculture, digital twins can optimize crop yields by simulating different cultivation methods and environmental factors. In retail, they can enhance customer experience by analyzing shopping patterns and inventory management.
Other relevant industries include:
- Smart grid management: Digital twins can help in monitoring and controlling energy systems.
- Logistics and supply chain: By modeling entire supply chains, companies can identify bottlenecks and optimize resource allocation.
- Urban planning: Digital twins of smart cities provide valuable data for city planners, allowing for better resource distribution and enhanced infrastructure planning.
Regulatory Developments
As digital twin technology evolves, so does the need for appropriate regulations. Governments and governing bodies are beginning to recognize the importance of establishing frameworks to ensure data security and ethical implications in the use of digital twins. Regulations will likely focus on three main areas: data privacy, interoperability, and standards for implementation.
Some important aspects to consider include:
- Data privacy: Given the large amounts of data that digital twins generate, regulations must protect user privacy and sensitive information.
- Standardization: Establishing common protocols and standards can improve compatibility across different systems and platforms.
- Ethical considerations: With AI becoming integral in decision-making processes, addressing ethical implications is essential to maintain public trust.
"Digital twin technology is not just a passing trend. Its integration into various sectors can lead to significant improvements in efficiency and understanding."
In summary, the future of digital twin technology looks promising. Continued advancements in AI and its application across emerging industries will fuel its growth. However, regulatory developments must keep pace to address the challenges presented by this innovative technology.
Case Studies of Digital Twin Applications
The case studies of digital twin applications are essential for understanding the practical implications of this technology. By examining real-world examples across various industries, we can glean insights into how digital twins are being utilized to solve complex problems, enhance efficiency, and drive innovation. Each case study provides a unique perspective on how digital twin technology is not just a theoretical concept but a tangible asset that organizations can leverage. This section will highlight key implementations within the automotive industry, urban infrastructure, and healthcare monitoring.
Automotive Industry Innovations
In the automotive sector, digital twin technology is reshaping manufacturing and vehicle design processes. Companies like BMW and Ford are investing heavily in creating digital replicas of their vehicles. These replicas allow for extensive testing and simulation, resulting in reduced development times and improved performance.
Through creating a digital twin of a vehicle, manufacturers can analyze how different parts interact under various conditions. This capability facilitates not only the optimization of performance but also predictive maintenance. For example, by using real-time data collected from sensors in vehicles, manufacturers can predict potential failures and schedule maintenance before issues arise, ultimately enhancing customer satisfaction and safety.
Smart Urban Infrastructure
Cities around the world are adopting digital twin technology to create smart urban environments. Digital twins help in visualizing city data, managing public services, and improving urban planning. Cities like Singapore and Barcelona have implemented these technologies to manage traffic flows and optimize resource distribution.
For instance, Singapore's digital twin integrates data from various sources, including traffic sensors, weather patterns, and public transportation schedules. This integration allows city planners to simulate different scenarios and make informed decisions to enhance traffic management and minimize congestion. The benefits extend to improving public safety and enhancing the overall quality of life for residents.
Healthcare Monitoring Solutions
In the healthcare sector, digital twins are proving transformative in patient monitoring and treatment planning. Organizations like Philips and Siemens are utilizing digital twins for personalized medicine approaches. By creating a digital representation of a patient, healthcare providers can simulate how different treatments will affect individual health outcomes.
For example, a digital twin of a patient's heart can allow doctors to visualize and analyze the specific rhythms and functions of that heart. This technology aids in identifying potential risks and optimal treatment plans tailored to the patient’s unique physiological characteristics. Such advancements result in improved patient outcomes and more efficient use of healthcare resources.
"Digital twin technology is truly revolutionizing how industries operate, preparing them for future challenges and opportunities."
Closure and Summary
In this article, we have delved into the multifaceted world of digital twin technology. Understanding this technology is vital as it serves as a conduit between the physical and digital realms. It offers a framework for organizations aiming to optimize processes and enable better decision-making. Digital twins allow for real-time monitoring and simulation, thereby accelerating innovation across various sectors.
Recap of Key Concepts
Digital twin technology integrates several key components. The relationship between the physical entity and its virtual counterpart is foundational. Data integration and the use of Internet of Things (IoT) devices play critical roles in ensuring accuracy and real-time relevance. This article also highlighted the technological frameworks supporting digital twins, particularly focusing on artificial intelligence and cloud computing.
We explored applications across multiple industries, including manufacturing, healthcare, and smart cities. Each use case demonstrates the capability of digital twins to enhance efficiencies and optimize performance. The benefits are twofold: improving diagnostics and extending the life of physical assets through predictive maintenance.
Moreover, the challenges of implementing digital twins were also discussed. Issues around data security, scalability, and system integration require careful attention. These challenges present considerations for organizations looking to adopt this innovative technology.
Final Thoughts on Digital Twins
As we look to the future of digital twin technology, its potential appears increasingly promising. Advancements in AI and machine learning will likely enhance the capabilities of digital twins. Emerging industries also stand to benefit as they integrate these systems into their operations. Regulatory developments will further shape their implementation and evolution.
Ultimately, digital twins are poised to revolutionize how industries operate, making processes more efficient and data-driven. By understanding and implementing this technology, organizations can lead their sectors into a new era of innovation and efficiency.