Structured and Unstructured Data in Healthcare
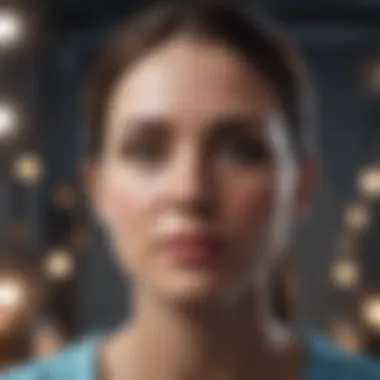
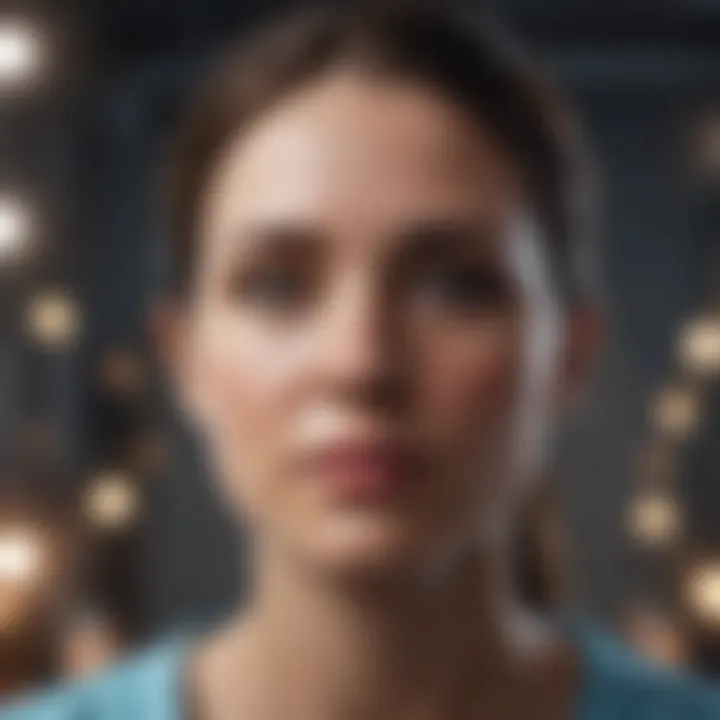
Intro
Data plays a crucial role in healthcare, influencing both administrative functions and clinical practice. In the context of healthcare, data can be classified into two primary categories: structured and unstructured data. Each type has its own characteristics, strengths, and challenges that significantly impact medical decision-making, patient outcomes, and overall healthcare efficiency.
Understanding the difference between these data types is essential for professionals working in the field. This article aims to explore the critical aspects of structured and unstructured data in healthcare, emphasizing their significance and implications for effective data management and analysis.
Moreover, recent advances in technology are paving the way for enhanced data integration and analytics. As healthcare continues to evolve, the thoughtful application of these data types will become increasingly vital to improving care outcomes and supporting clinical decisions.
Overview of Research Topic
Brief Background and Context
The healthcare industry produces vast amounts of data at an unprecedented rate. This data ranges from structured data, such as laboratory test results and electronic health records, to unstructured data, such as clinical notes, imaging, and patient-generated content.
Structured data is organized in a predefined format, making it easily searchable and analyzable. In contrast, unstructured data lacks a specific format or structure, making it more challenging to manage. Yet, both types of data are invaluable in painting a comprehensive picture of patient health and treatment options.
Importance in Current Scientific Landscape
In the current scientific and healthcare landscape, the significance of leveraging structured and unstructured data cannot be overstated.
- Decision Making: Structured data often supports clinical guidelines and protocols, while unstructured data can provide deeper insights into patient experiences and outcomes.
- Research Advancements: Integration of both data types enhances research capabilities, providing a fuller understanding of diseases, treatment responses, and patient demographics.
- Patient Outcomes: Effectively utilizing these data types contributes to improved patient care, as healthcare professionals are better equipped to personalize treatments and interventions.
Challenges and Opportunities
While both structured and unstructured data offer tremendous potential, they also pose challenges related to data management, integration, and safeguarding patient privacy. However, advancements in technologies such as artificial intelligence and machine learning are opening new avenues for transforming unstructured data into actionable insights, driving better healthcare outcomes.
Prologue to Healthcare Data
The burgeoning field of healthcare data has emerged as a critical component in the effective delivery of medical services and improved patient outcomes. Understanding the different types of healthcare data—structured and unstructured—is crucial for professionals in the industry. This overview will elucidate key points about the nature of healthcare data and its implications.
Definition of Healthcare Data
Healthcare data refers to a collection of information generated through medical practices, which encompasses patient records, treatment histories, clinical trials, and more. This data can be broadly categorized into two distinct types: structured data, which follows a predefined format, and unstructured data, which is not easily categorized. Structured data typically includes details such as patient demographics, diagnoses, and treatment codes. In contrast, unstructured data may encompass a variety of formats like free-text notes, images, and even audio recordings.
Understanding the definitions is paramount. The evolving landscape of healthcare demands that professionals appreciate the nuances of these data types. Accurate definitions provide a foundation for healthcare administrators, researchers, and practitioners to optimize data usage.
Importance of Data in Healthcare
Data plays an influential role in modern healthcare. Its significance lies primarily in its ability to inform clinical decisions, streamline operations, and drive patient care improvements. As healthcare becomes increasingly data-driven, organizations rely on insights drawn from vast datasets to enhance decision-making processes.
The benefits of leveraging data in healthcare include:
- Enhanced Patient Care: By using data analytics, providers can tailor treatment plans based on comprehensive patient profiles.
- Operational Efficiency: Data enables health systems to identify inefficiencies and allocate resources effectively.
- Research and Development: Data plays a vital role in advancing medical research and creating new treatments.
Moreover, as technology evolves, the ability to capture, manage, and analyze data continues to grow. Healthcare professionals must recognize the pivotal role that accurate data plays in ensuring quality care and operational excellence. In today's world, the ability to turn data into actionable insights is a vital skill.
"Data is the new oil, and in healthcare, it powers decisions that can save lives."
Categorizing Healthcare Data
Understanding the different types of healthcare data is crucial for both practitioners and researchers. Categorizing healthcare data allows organizations to make informed decisions about data management, analysis, and utilization. The distinctions between various data types influence how they are stored, retrieved, and utilized, impacting overall patient care and operational effectiveness.
In this section, we explore the primary categories of healthcare data, focusing on structured and unstructured formats. Each type has its own unique characteristics, benefits, and challenges that need to be understood in order to leverage data effectively.
Overview of Data Types
Healthcare data can generally be divided into two primary categories: structured data and unstructured data. Structured data refers to information that is organized in a predefined manner, often in a database or spreadsheet format. Examples include:
- Patient demographics
- Laboratory results
- Billing information
In contrast, unstructured data encompasses information that does not adhere to a specific format. This includes:
- Clinical notes from healthcare providers
- Medical imaging files
- Patient feedback from surveys
Each type of data operates within its own operational framework. The structured data is easier to process and analyze since it fits neatly into relational models. Unstructured data, however, provides richer context and insights, despite its challenges in processing and analyzing.
Differentiating Structured and Unstructured Data
The differences between structured and unstructured data are significant in healthcare. Structured data offers clarity and uniformity, making it easier to maintain and analyze. For instance:
- Structured data is often collected via systematic processes, reducing errors and ensuring consistency.
- It allows for quick retrieval using SQL queries or similar methods, facilitating analytics and reporting.
- Examples such as Electronic Health Records (EHRs) showcase how structured data aids in clinical decision-making by providing necessary patient information at a glance.
On the other hand, unstructured data holds immense potential insights that structured data may not capture. It offers valuable, qualitative observations that can enhance patient care, but the challenges are notable:
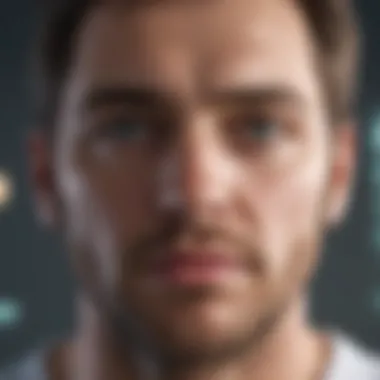
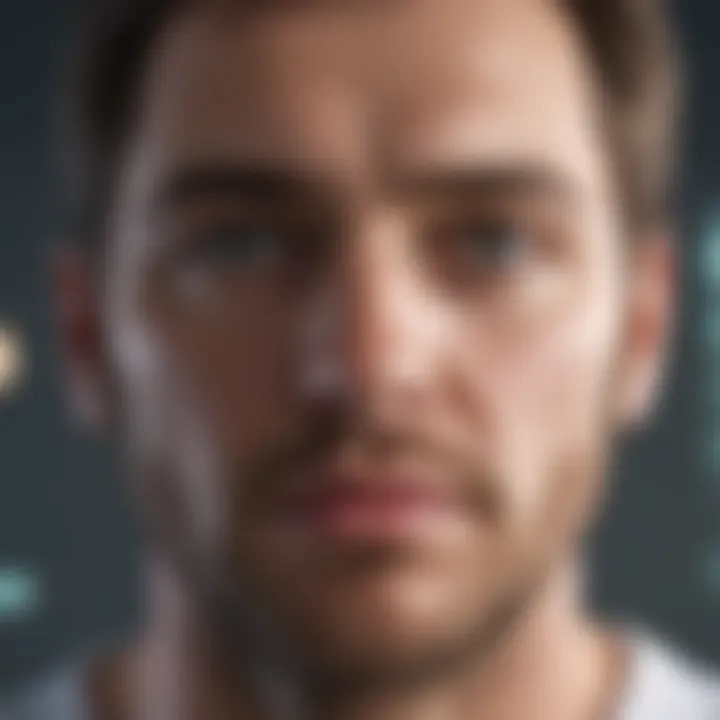
- Unstructured data is varied, fragmented, and dispersed across different systems, making it difficult to consolidate and analyze.
- Processing this data often requires advanced technologies like Natural Language Processing, as it involves understanding human language and context.
The integration of both data types is essential for a complete picture of patient health and clinical effectiveness. By recognizing how structured and unstructured data complement each other, healthcare professionals can optimize their data strategies for improved patient outcomes and operational efficiency.
Characteristics of Structured Data
Structured data presents a well-defined format that allows for easy storage, retrieval, and processing. In the healthcare sector, characteristics of structured data play a pivotal role in optimizing various medical operations. Understanding these characteristics facilitates better data management practices, leading to improved patient care and efficiency within healthcare systems.
Format and Organization
The format of structured data is systematic. Typically, it conforms to a predictable schema or model. Data points are often stored in rows and columns, resembling a table. This organization enables rapid access and effective queries, which are crucial in time-sensitive environments like hospitals and clinics.
For example, a patient’s demographic information may reside in a centralized database with fields for name, age, and medical history. This standardization allows healthcare providers to easily access necessary information without confusion or ambiguity. The clear organization minimizes errors that can occur in chaotic situations where decisions must be made swiftly.
Examples in Healthcare
Electronic Health Records (EHRs)
Electronic Health Records represent a significant advancement in healthcare data management. EHRs compile comprehensive patient information, including medical history, allergies, medications, and lab results into a single digital record. The key characteristic of EHRs is their ability to centralize critical health information.
This digital approach enhances shared access among healthcare providers, which fosters coordinated care. However, transitioning from paper-based records to digital EHRs can be resource-intensive. Implementation can involve substantial training for healthcare personnel.
Clinical Databases
Clinical databases store structured information collected during clinical trials or patient care. A key characteristic of these databases is their ability to analyze data trends over time. For instance, a database may track patient outcomes related to specific treatments, generating valuable insights.
The unique feature of clinical databases is their capacity to handle large quantities of standardized data efficiently. However, maintaining the quality and integrity of such data is vital. Inaccuracies can lead to misleading conclusions that affect healthcare practices.
Billing Codes
Billing codes categorize services and procedures performed by healthcare providers. They follow strict guidelines established by organizations like the American Medical Association. A key characteristic of billing codes is their standardization across the industry.
This standardized approach simplifies the process of coding and billing, leading to fewer claims denials. However, the complexity of billing codes can present challenges. For instance, coding errors can result in delayed payments or legal issues, making it imperative for staff to be adequately trained.
Advantages of Structured Data
Structured data offers several distinct advantages that benefit healthcare operations.
Easy Retrieval
One major advantage of structured data is its easy retrieval. Because of the organized format, healthcare providers can quickly pull relevant patient information. This rapid access can be crucial in emergency situations.
Unique features like database query languages allow for efficient data searches. In situations where time is a factor, quick retrieval can impact patient outcomes positively.
Data Consistency
Another significant advantage is data consistency. Structured data follows predefined formats, which minimizes discrepancies in information entry and retrieval. This consistency is vital when managing patient data across various departments.
However, inconsistency can still occur when data is entered manually. Regular audits and validation checks can mitigate risks associated with data inconsistencies.
Facilitation of Analytics
Structured data simplifies analytics, as it is easier to analyze large datasets meaningfully. Tools designed for data analysis can handle structured data efficiently, providing insights to improve patient care and operational efficiency.
The unique feature here is the ability to produce reports effortlessly, which are crucial for decision-making processes. However, reliance solely on structured data may limit understanding if unstructured data holds critical insights not captured in this format.
Characteristics of Unstructured Data
Unstructured data constitutes a significant part of the information generated and used in healthcare. Understanding its characteristics is crucial, as it can impact patient care, clinical outcomes, and operational efficiency. Unlike structured data, which is organized in a predefined format, unstructured data lacks a specific model. This flexibility offers both advantages and challenges, making it an important component of the healthcare data landscape.
Nature and Format
Unstructured data can be seen as raw, which does not fit neatly into tables or databases. It includes sources like text, audio, video and images. This type of data often contains valuable insights that are not immediately evident. The nature of unstructured data means that it can be more complex to analyze. However, its versatility can also provide wider context for patient care and treatment pathways. The challenge lies in organizing and interpreting this data, which is paramount for effective decision-making in healthcare.
Examples in Healthcare
Clinical Notes
Clinical notes are an essential part of patient records. They are written by healthcare providers during patient visits. Clinical notes enable the documentation of patient's history, symptoms, and treatment plans. One key characteristic of clinical notes is their contextual richness. They provide nuanced details that structured data may overlook. This makes clinical notes a valuable source of insights for both patient management and research.
However, due to their free-form nature, extracting specific information from clinical notes can be challenging. Tools such as Natural Language Processing can assist in interpreting these narratives, transforming them into more readable formats, but they still may require human oversight for accuracy. Thus, the value of clinical notes lies in their content, but the challenges are in processing them effectively.
Medical Images
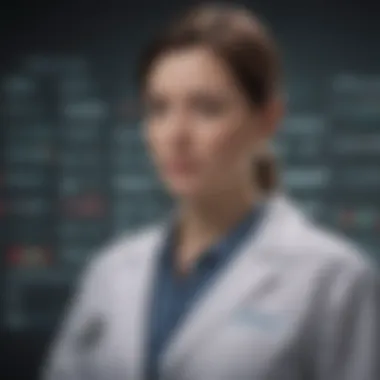
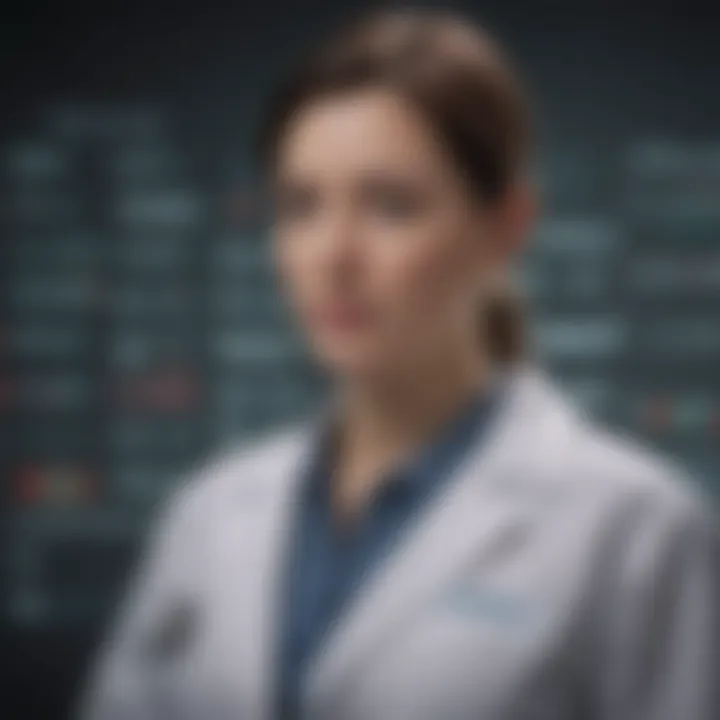
Medical images, including X-rays and MRIs, are crucial for diagnosis and treatment. They provide a visual representation of a patient’s condition and are often unstructured data. One key characteristic of medical images is their ability to convey complex information quickly. This allows for immediate analysis by specialists.
The challenge with medical images is the need for advanced technology and trained personnel to analyze them accurately. Additionally, storing high-resolution images can require significant storage capacity. Despite these challenges, medical images remain irreplaceable due to their diagnostic capabilities in healthcare.
Patient Feedback
Patient feedback, collected through surveys or informal reviews, helps healthcare facilities understand patient experiences. This unstructured data provides insights into the quality of care, areas needing improvement, and patient satisfaction. One key characteristic of patient feedback is its diversity, as it captures various perspectives.
However, interpreting patient feedback can be difficult. Negative feedback can often have more emotional weight and may not accurately represent general experiences. Tools that aggregate feedback can help identify trends, but direct interpretation may lack nuance. Patient feedback remains central to improving care quality in a patient-centered approach.
Challenges of Unstructured Data
Unstructured data offers significant advantages but also presents several challenges. These include difficulties in data interpretation, storage complexity, and analysis hurdles.
Data Interpretation
Interpreting unstructured data requires skilled analysts who can discern relevant information. This task is often complex due to variations in language, context, and meaning. It necessitates significant time and resources.
Storage Complexity
Storing unstructured data requires robust systems to handle its variety and volume. It can lead to challenges in management and accessibility, often requiring advanced storage solutions.
Analysis Difficulties
Unstructured data presents unique analysis challenges. Traditional analytical tools may not work effectively with this type of data. Advanced techniques like machine learning are needed to extract meaningful insights, but they can be resource-intensive.
Understanding the characteristics of unstructured data is critical. It affects how healthcare data is managed, interpreted, and utilized. By recognizing both the potential and challenges of unstructured data, healthcare professionals can better leverage this vital information.
Combining Structured and Unstructured Data
In the modern healthcare landscape, combining structured and unstructured data emerges as a critical approach to improve patient care and streamline processes. While structured data provides well-defined, easily accessible information, unstructured data carries a wealth of contextual and nuanced details that are often overlooked. This integration retains the advantages of both data types, enabling healthcare practitioners to make more holistic decisions. By leveraging both forms of data, the medical community can gain insights that neither could provide alone. Furthermore, the combined use of structured and unstructured data fosters innovative solutions in medical research, clinical practices, and operational management.
Benefits of Integration
One of the main benefits of integrating these data types is the enriched knowledge base it creates. Structured data, like coded clinical outcomes, can be combined with unstructured data from sources such as clinical notes, generating a more comprehensive understanding of patient health. This synergy can lead to improved clinical outcomes, as it allows healthcare professionals to analyze a wider array of information. Moreover, integrating these data types can enhance operational efficiency by streamlining workflows and automating tasks traditionally done manually.
Use Cases in Healthcare
Personalized Medicine
Personalized medicine stands out significantly in tailoring treatments based on individual patient profiles. It relies on data analytics that benefits from both structured and unstructured data. The structured elements, like genetic information, are crucial, but unstructured data, such as previous treatment responses noted by physicians, are equally essential. This integration allows the healthcare provider to create highly individualized care plans, drastically improving patient outcomes. Its unique feature is the focus on the patient as a whole rather than only their disease, opening avenues for more targeted therapies.
Predictive Analytics
Predictive analytics represents another core aspect where combining data types proves invaluable. Integrating historical structured data with unstructured patient experiences can predict health trends and outcomes. For example, structured data from electronic health records can signal risks of chronic conditions, while unstructured data may give insights into lifestyle factors through patient feedback. The key characteristic of predictive analytics is its ability to forecast potential issues before they arise. This preemptive approach leads to proactive healthcare interventions and reduced long-term costs.
Comprehensive Patient Profiles
Comprehensive patient profiles are vital in providing a full scope of patient care. By merging structured data, such as demographics and lab results, with unstructured data like personal health histories and preferences, healthcare providers can create profiles that offer deeper insights into patient needs. This comprehensive overview enhances decision-making processes and can significantly improve patient satisfaction. The unique feature of comprehensive patient profiles is their adaptability, allowing for ongoing updates and adjustments as new information becomes available. Such profiles not only aid individual patient care but also support population health management strategies.
"The combination of structured and unstructured data acts like a bridge, connecting fragmented health information to form a cohesive picture of patient care."
Thus, the integration of structured and unstructured data in healthcare leads to innovative solutions and enhanced patient experiences, paving the way for a more effective healthcare system.
Technology and Data Management
Technology plays a crucial role in the management of both structured and unstructured data in healthcare. The sheer volume and variety of data generated in this sector necessitate sophisticated tools and methods for effective handling. Proper data management ensures that healthcare providers can make informed decisions, enhance patient outcomes, and streamline operations. Implementing technology for data management leads to improved data accuracy, improved retrieval processes, and reduced costs for healthcare organizations.
Tools for Managing Structured Data
In healthcare, structured data tools are essential for organizing and maintaining accurate patient information. Electronic Health Records (EHR) systems like Epic and Cerner facilitate the storage and access of structured data. These systems allow healthcare professionals to quickly locate relevant patient information. Furthermore, they support interoperability by enabling data sharing between different healthcare providers.
Advantages of structured data management tools include:
- Improved data accuracy
- Enhanced reporting capabilities
- Streamlined billing processes
Tools for Managing Unstructured Data
Managing unstructured data is particularly challenging due to its unpredictable nature. Tools such as IBM Watson and Nuance's Dragon Medical One utilize advanced algorithms to process and analyze unstructured data effectively. These solutions help in extracting meaningful information from clinical notes and medical imaging.
Key benefits include:
- Enhanced efficiency in review processes
- Better insights from patient feedback
- Improved diagnostic accuracy
Emerging Technologies in Data Integration
Emerging technologies bring forth transformative capabilities for integrating structured and unstructured data.
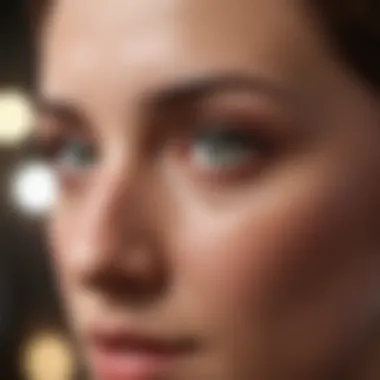
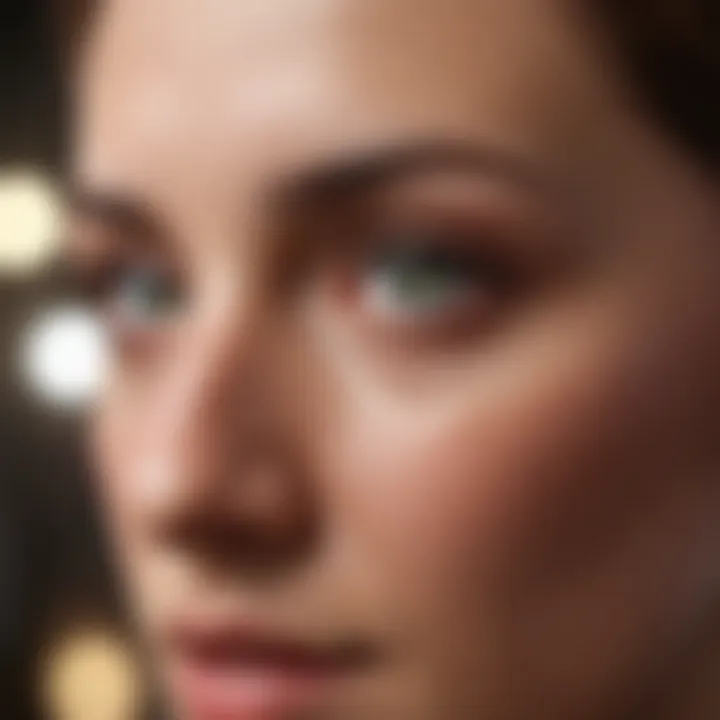
Artificial Intelligence
Artificial Intelligence (AI) contributes significantly to data integration by automating repetitive tasks and providing analytical insights. A key characteristic of AI is its ability to learn from data patterns. This adaptability makes it a popular choice for tackling complex healthcare data challenges. Unique features include predictive analytics and natural language processing, providing healthcare professionals with actionable insights. Advantages of AI include increased efficiency and accuracy, while potential downsides may involve high implementation costs and reliance on data quality.
Natural Language Processing
Natural Language Processing (NLP) enhances the integration of unstructured data by converting free text into a structured format. One of its key characteristics is the capability to understand context in clinical notes, making it beneficial for extracting crucial patient information. Its unique feature lies in its ability to automate documentation processes. Advantages of NLP include time savings and improved data accuracy; however, challenges may arise from variations in language usage.
Machine Learning
Machine Learning (ML) aids in recognizing patterns and making predictions based on historical data. A key characteristic of ML is its predictive power, which allows it to identify trends over time. This establishes it as a beneficial element in enhancing patient care through personalized medicine. Its unique feature includes the ability to adapt as new data becomes available. The advantages of ML are found in increased predictive accuracy, while potential disadvantages may pertain to data privacy concerns.
"The integration of technology in data management not only optimizes healthcare operations but also helps to secure better clinical outcomes for patients."
By leveraging these advanced technologies, healthcare organizations can overcome the complexities of data management, leading to a more efficient and effective healthcare system.
Data Security and Privacy Concerns
Data security and privacy are critical components of healthcare data management. As healthcare continues to be digitized, the susceptibility of sensitive patient information rises. Recognizing the importance of securing structured and unstructured data ensures not only compliance with regulations but also maintains patient trust. This section will address key regulatory frameworks governing data security and highlight challenges in achieving optimal data protection.
Regulatory Frameworks
Healthcare data is governed by an array of legal frameworks aimed at protecting patient privacy and confidentiality. In the United States, the Health Insurance Portability and Accountability Act (HIPAA) sets standards for sensitive patient information. Under HIPAA, healthcare providers, insurers, and their business associates must safeguard Protected Health Information (PHI).
Compliance with these regulations mandates the implementation of both administrative and technical controls, safeguarding against unauthorized access to data. Moreover, various states have additional regulations that further specify data protection requirements. In the European Union, the General Data Protection Regulation (GDPR) also plays a pivotal role in shaping data handling practices. Organizations dealing with EU citizens' data must adhere to strict obligations, including obtaining explicit consent for data processing.
Fair Use policies in these frameworks provide guidelines on how data can be utilized within the healthcare context while ensuring the privacy of individuals.
Challenges in Data Protection
Despite existing regulations, numerous challenges remain in effectively protecting healthcare data. One major issue is the increasing sophistication of cyber attacks. Hackers target healthcare systems to exploit vulnerabilities, making data breaches more common. Healthcare organizations must therefore continually assess and enhance their security measures to protect sensitive data.
Additionally, the variety of data formats—whether structured or unstructured—complicates data protection efforts. Unstructured data, like clinical notes or imaging files, often lacks a uniform protection schema, making it difficult to enforce consistent security practices.
Human error is another significant challenge. Employees may inadvertently expose data through negligence, such as improper sharing of login credentials or failure to follow access controls.
"It’s not just about compliance, it’s about building a culture of security within healthcare organizations"
Finally, the interoperability of different healthcare systems presents hurdles. Integrating data from various sources enriches analysis but can lead to gaps in security. Ensuring that all systems comply with privacy standards is essential for cohesive data protection efforts.
Addressing these challenges requires continuous education and training for healthcare professionals, along with investment in advanced security technologies. Moreover, fostering a culture of accountability within healthcare organizations will significantly enhance data security and compliance with regulatory frameworks.
Future Trends in Healthcare Data
Understanding future trends in healthcare data is essential for various stakeholders operating in this complex landscape. The interaction between structured and unstructured data continues to evolve. These changes are not just technological but also procedural. Organizations must adapt to emerging methods for data utilization which can significantly impact patient care and operational efficiency.
Next Steps in Data Utilization
As data utilization progresses, several steps need to be taken. Healthcare providers are actively exploring the following:
- Integration of Advanced Analytics: Tools such as predictive analytics and real-time data monitoring are gaining traction. These techniques allow for the extraction of actionable insights that can improve patient outcomes.
- Enhanced Data Interoperability: Seamless data exchange between systems is crucial. Institutions are focusing on building infrastructures that support interoperability, which enhances patient care coordination.
- Utilization of Patient-Generated Health Data: Engaging patients in their health journeys can yield valuable data. Healthcare providers must invest resources to integrate this information effectively.
Developing these capabilities will require thoughtful planning and investment. Organizations should focus on training personnel in data management and ensuring compliance with data privacy regulations.
Vision for Data-Driven Healthcare
The vision for a data-driven healthcare system is dynamic and transformative. Potential outcomes of this vision include:
- Personalized Treatment Plans: Tailoring healthcare solutions based on individual patient data may become commonplace. This approach can result in more effective treatments and increased patient satisfaction.
- Operational Efficiency Improvements: Data can streamline administrative operations. This might include scheduling, billing, and resource allocation processes, leading to cost savings.
- Proactive Patient Care Models: With more sophisticated data analysis, healthcare providers can move towards anticipatory care. This should reduce hospital readmissions and create better health outcomes for patients.
By embracing a data-driven framework, healthcare systems can become more proactive and patient-centered. Organizations must remain flexible and adapt to changes in data technologies and practices, fostering continuous improvement in care delivery.
"The convergence of data technology and healthcare is poised to redefine how patients are treated, leading to improved outcomes and efficiencies."
Everyone involved in healthcare must stay updated on trends in data utilization. This includes knowing what advancements to anticipate and how to prepare for their impacts.
Closure
In wrapping up the discussion on structured and unstructured data within the healthcare sector, it becomes clear that these data types play crucial roles in shaping modern medical practices. Understanding their characteristics and implications is vital for optimizing healthcare operations and improving patient care.
Summarizing Key Points
- Definition and Categories: The article illustrates the distinction between structured and unstructured data. Structured data is highly organized and easily searchable, while unstructured data includes information that is not easily categorized, such as free text and images.
- Practical Examples: We provided specific examples from the healthcare realm, including electronic health records for structured data and clinical notes for unstructured data. Both types of data contribute uniquely to patient data management.
- Integration Benefits: The integration of both data types is essential for comprehensive patient profiles. It allows healthcare professionals to leverage insights from varied sources, enhancing decision-making.
- Technological Implications: Advanced technologies such as artificial intelligence and machine learning are transforming how structured and unstructured data are managed. These tools facilitate the analysis and integration of vast amounts of data, leading to better healthcare outcomes.
Implications for Healthcare Professionals
Healthcare professionals must acknowledge the growing significance of data in their field. The effective use of both structured and unstructured data can lead to improved patient outcomes through informed decision-making.
- Data Literacy: Professionals should develop their skills in data analysis. Understanding the nuances of data can help in interpreting complex patient information effectively.
- Policy and Governance: It’s important to stay updated on the policies surrounding data security and privacy. The protection of patient data is paramount and adherence to regulations helps maintain trust.
- Adaptation to Technology: Embracing new technologies that enhance data integration and analysis will be crucial. This will equip professionals to harness the full potential of healthcare data in achieving high-quality care.