One Models: A Comprehensive Overview of Their Impact
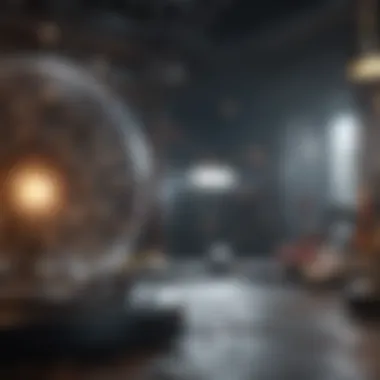
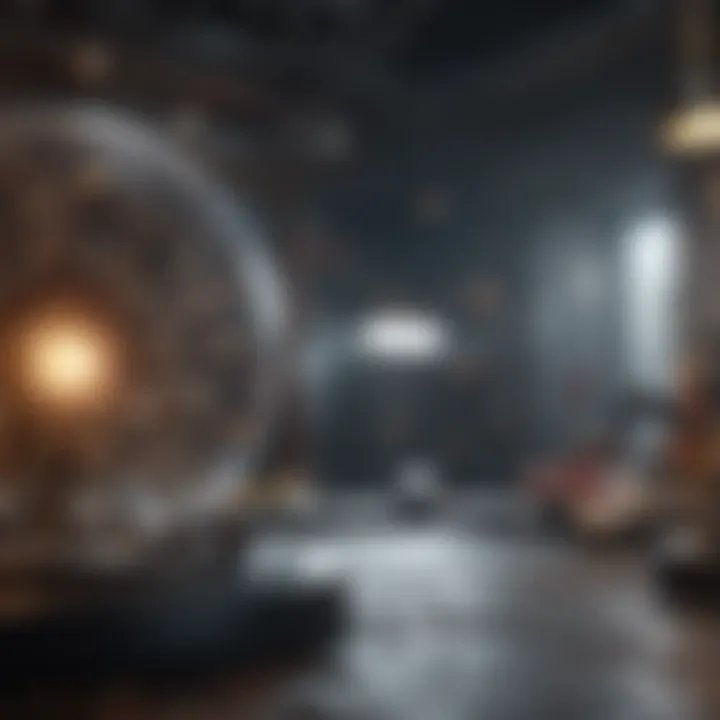
Overview of Research Topic
Understanding the concept of one models is crucial across various fields in science. This overview will highlight the principles, applications, and implications of these models.
Brief Background and Context
One models provide a simplified representation of complex phenomena. They are used to predict and analyze behaviors within diverse scientific disciplines like physics, biology, and economics. These models typically rely on established theories and frameworks, facilitating the interpretation of data and aiding in hypothesis testing. The development of one models has been influenced by advances in computational power and mathematical techniques, allowing for more precise predictions.
Importance in Current Scientific Landscape
In the present scientific landscape, one models serve as essential tools. They enhance our understanding of intricate systems and play a pivotal role in decision-making processes. For example, in climate science, one models help predict weather patterns and assess the impact of climate change. Their relevance is also notable in econometrics, where they analyze market trends and inform policy decisions. Consequently, grasping the essentials of one models is vital for students, researchers, educators, and professionals alike.
Methodology
A comprehensive exploration of one models requires an understanding of the methodologies used in their development.
Research Design and Approach
Research on one models commonly follows a systematic approach. The design often includes literature reviews, theoretical formulation, and empirical testing of the models. Researchers often start with existing theories, examining how they can be adapted to create a one model that addresses specific questions in a given field.
Data Collection Techniques
Data collection for testing one models employs various techniques. These may include:
- Surveys: Gathering information from specific populations or phenomena.
- Experiments: Conducting controlled experiments to observe model predictions.
- Observational data: Collecting existing data from reliable sources for analysis.
The choice of technique depends on the specific objectives of the study and the nature of the phenomena being modeled.
"One models are not just tools; they are frameworks allowing scientists to engage with the world more effectively."
Through this overview, readers can appreciate the significance of one models and the methodologies that underpin their application in scientific inquiry. The next sections will delve deeper into theoretical frameworks and case studies illustrating the practical utility of one models.
Foreword to One Models
The concept of "one models" plays a pivotal role in understanding complex systems across various scientific disciplines. They serve as simplified representations that focus on essential elements of a system, thus facilitating analysis and prediction. The primary benefit of employing one models lies in their capacity to distill complex phenomena into manageable frameworks, enabling researchers to derive insights without getting mired in overwhelming detail.
Defining One Models
One models generally refer to a class of theoretical models that aim to encapsulate the behavior of a system through a unified perspective. These models can take many forms, such as mathematical equations, algorithmic representations, or graphical illustrations. At their core, one models strive for simplicity while retaining essential characteristics of the phenomena they represent. The ease of interpretation becomes a critical factor, allowing practitioners from diverse fields to grasp underlying principles quickly. This accessibility is especially significant for students and researchers who seek a foundational understanding of complex systems.
Historical Context
The evolution of one models can be traced back to early scientific inquiry. Before the advent of modern computational tools, scientists relied on rudimentary forms of representation to understand their observations. Classical mechanics is one of the earliest disciplines where simplified models, such as Newton's laws of motion, were utilized to describe physical phenomena. As scientific understanding has expanded, so too have the methodologies used to create one models. The integration of statistical methods and computational approaches in the 20th century marked a significant advancement in model-building. This evolution illustrates not only the increasing complexity of models but also their ability to adapt to new discoveries and technological innovations. In todayโs context, one models are integral to disciplines as varied as biology, chemistry, and environmental science, proving their relevance and flexibility.
"Models are not just tools but frameworks for thinking critically about reality."
The historical perspective on one models highlights their necessity in shaping our comprehension of the world around us. This foundational understanding sets the stage for deeper exploration of their theoretical frameworks and applications across multiple scientific domains.
Theoretical Foundations
The theoretical foundations of one models are critical to understanding their relevance and application in scientific disciplines. This section outlines how theoretical frameworks shape the formation and usage of one models, as well as their implications for research integrity and advancement. By establishing a solid theoretical base, researchers improve the reliability and applicability of their models across various fields.
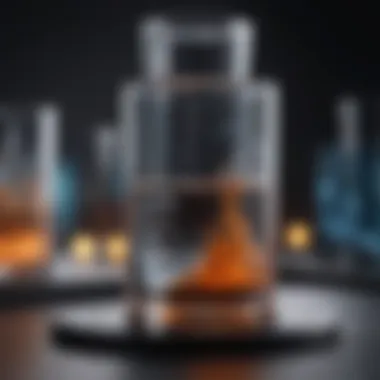
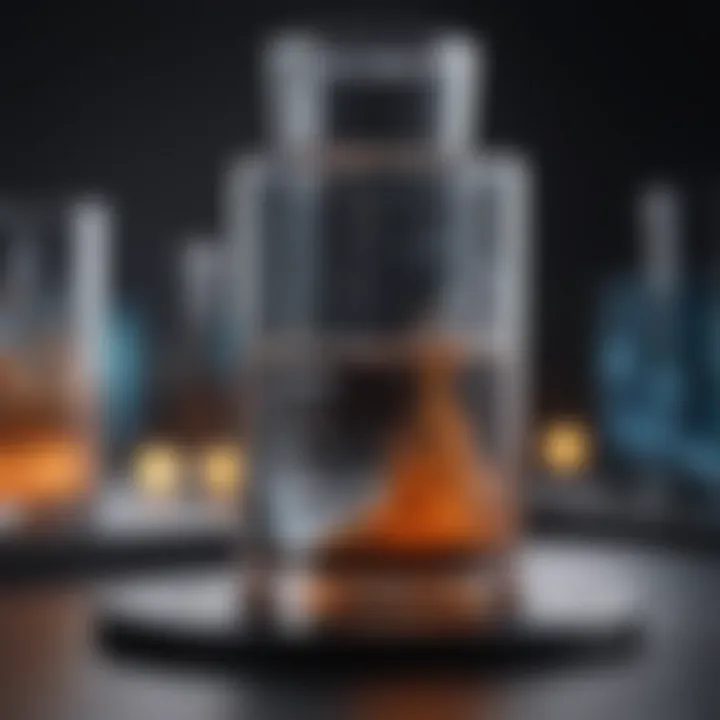
Mathematical Frameworks
Mathematical frameworks provide the backbone for developing one models. These frameworks encompass a range of mathematical tools and concepts that enable scientists to describe complex phenomena simply. Understanding these frameworks is vital for scientists who aim to analyze data and make predictions based on one models.
Key aspects of mathematical frameworks include:
- Formulation of equations: Mathematical equations offer a precise language for describing relationships within data.
- Computation techniques: Numerical methods and algorithms are employed to solve mathematical models when analytic solutions are infeasible.
- Simulations: Models are often assessed through simulations, allowing researchers to explore scenarios that are challenging to test in reality.
These components ensure that one models retain scientific rigor, allowing for effective communication of results and implications.
Statistical Considerations
Statistical considerations are another essential aspect of the theoretical foundations of one models. They support the validation and refinement of these models by applying statistical methods to assess their performance. In essence, statistics allows scientists to quantify uncertainty, which is crucial when interpreting model outputs.
The role of statistics in one models involves different facets, such as:
- Data analysis: Statistical techniques help distill large sets of data into meaningful insights that inform model development.
- Parameter estimation: Understanding how various parameters influence model behavior is critical, and statistical methods provide the tools to estimate these parameters effectively.
- Hypothesis testing: Researchers can utilize statistical tests to evaluate the goodness of fit of the model against observed data.
Ultimately, a strong statistical foundation enhances the credibility of one models, establishing them as legitimate tools within scientific inquiry.
"Mathematics is the language with which God has written the universe."
- Galileo Galilei
In summary, robust theoretical foundations encompassing mathematical frameworks and statistical methods are crucial for the development and application of one models. Understanding these foundations allows researchers to navigate the complexities of their disciplines with confidence.
Applications in Various Disciplines
Within the realm of scientific inquiry, the concept of one models plays a crucial role in various disciplines. Their ability to distill complex systems into understandable representations enhances both research and practical applications. Understanding how these models operate in different scientific fields not only contributes to theoretical advancements but also facilitates real-world impact. Therefore, this section focuses on specific applications of one models across biological sciences, chemical processes, physical sciences, and earth sciences.
Biological Sciences
One models have profound relevance in biological sciences, where they assist in understanding intricate biological systems. For instance, these models can simulate population dynamics, enabling researchers to predict how species interact within their ecosystems. They play an essential role in epidemiology, where models help track disease spread and the effects of intervention strategies.
Important Point: One models can provide insights that direct public health initiatives, improve resource allocation, and enhance understanding of disease patterns.
Additionally, one models are pivotal in genetic research, aiding in the study of gene interactions and their implications on phenotypic traits. The precision provided by these models supports advancements in personalized medicine, ensuring that therapies can be tailored to individual genetics.
Chemical Processes
In the field of chemistry, one models are instrumental in representing chemical reactions and processes. These models facilitate the understanding of reaction kinetics, helping chemists predict the speed of reactions under various conditions. Furthermore, one models are employed in designing catalysts, where they can simulate different configurations and their effectiveness in accelerating reactions.
The potential of one models in chemical engineering is significant. They allow for better optimization of processes, ensuring efficiency and sustainability. Computational chemistry, leveraging one models, aids in predicting molecular behavior, opening avenues for novel materials and compounds development.
Physical Sciences
The physical sciences greatly benefit from one models, especially in physics and engineering. In physics, these models can describe phenomena such as fluid dynamics, thermodynamics, and electromagnetism. They provide essential insights that lead to technological advancements, such as improved energy systems and materials with novel properties.
For engineers, one models can simulate structural integrity, predicting how materials will react under different loads and conditions. This application is vital for civil engineering, where safety and durability of structures are paramount.
Earth Sciences
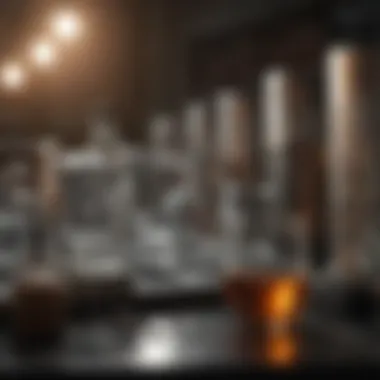
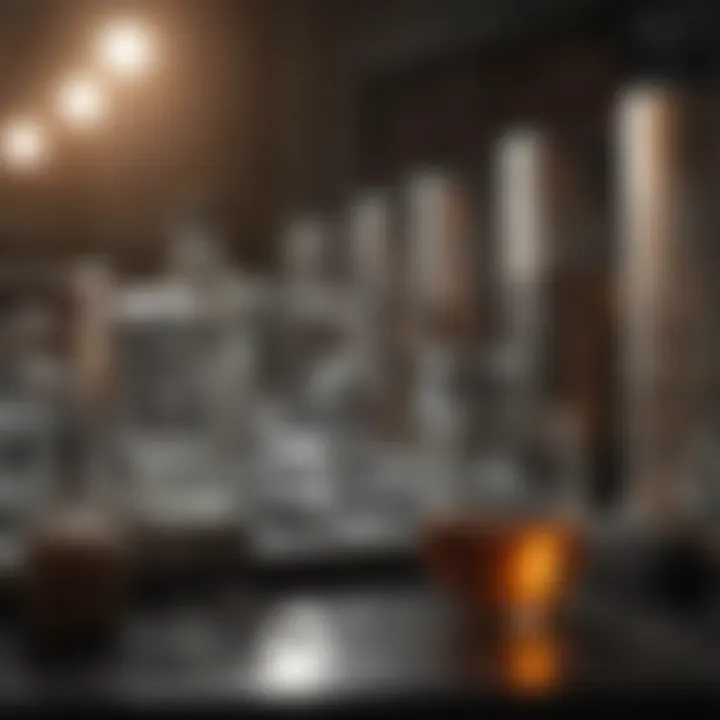
In the earth sciences, one models are crucial for understanding complex environmental systems and phenomena. They help in predicting weather patterns, climate change impacts, and geological events such as earthquakes. These models can analyze vast datasets to establish correlations and make informed predictions about future conditions.
Models in this field also support resource management, enabling better decision-making regarding water resources, agriculture, and land use. The integration of one models into earth sciences is imperative for addressing sustainability challenges and informing policy decisions.
Methodological Approaches
Methodological approaches form the backbone of research involving one models. They encompass the systematic methods researchers use to gather, analyze, and validate data. Effective methodologies ensure that findings are robust and applicable across various scientific domains. For one models, specific elements play a crucial role. By focusing on data collection and model validation processes, these approaches enhance the understanding and applicability of the models being utilized.
Data Collection Techniques
Data collection is fundamental in constructing any model. It not only informs the framework but also impacts its accuracy significantly. In the context of one models, various data collection techniques can be employed. Qualitative and quantitative methods are widely used. Quantitative data can be collected through surveys, experiments, and sensor readings. On the other hand, qualitative data might be gathered through interviews and observations.
Additionally, leveraging technology has revolutionized data collection. For instance, tools like satellite imaging and data monitoring systems provide real-time data, enhancing the model's relevance.
"The technique of data collection can greatly influence the outcomes and implications of one models."
Choosing the appropriate method depends on the research question and the nature of the data required. Careful consideration must be given to sample size and selection. Properly designed protocols enhance reliability and validity.
Model Validation Processes
Validation is essential for ensuring that the one models function correctly and produce results that can be trusted. Model validation processes verify that the model accurately represents the real-world phenomena it intends to simulate. It involves comparing predicted outcomes with observed data.
Different validation methods exist, such as:
- Cross-validation: This technique tests the model against different subsets of data to check for reliability.
- Sensitivity analysis: This process assesses how changes in input variables affect the output, helping to understand the model's robustness.
- Calibration: Adjusting model parameters based on observed data ensures that the model operates accurately within specified limits.
Researchers must rigorously document the validation process. This not only ensures transparency but aids fellow scientists in scrutinizing and replicating the research. Ultimately, robust validation lays the groundwork for confidence in model applicability, especially as new challenges and questions emerge in scientific inquiry.
Advancements driven by One Models
The evolution of one models significantly shapes the landscape of scientific inquiry. These models are instrumental in providing simplified representations of complex systems, which facilitates a deeper understanding of various phenomena. In this section, we will articulate how innovations in research and real-world applications arise from one models. Each element elaborated upon unveils the transformative potential that these models hold within scientific fields.
Innovations in Research
One models foster innovations across numerous research domains. They provide the foundational structure for hypotheses, calculations, and experimental designs. For instance, in biology, one models can help simulate ecological interactions, leading to novel experiments and insights into biodiversity. Furthermore, they allow researchers to test various scenarios quickly without the need for extensive empirical data collecting initially. This significantly reduces the time and resources spent, as researchers can narrow down promising avenues for further investigation.
Moreover, the iterative process of model refinement is crucial. As data become available, models can be adjusted to enhance their predictive accuracy. This aspect of one models is paramount, allowing for continuous improvement and innovation in research methodologies. The integration of technology has also played a pivotal role. With advancements in computational power and programming, researchers can now employ intricate simulations yielding important data that inform future studies.
Real-World Applications
Real-world applications of one models span across various fields, providing quantifiable solutions to pressing issues. In environmental science, for example, one models are utilized to predict climate change impacts, enabling policymakers to strategize effectively. They enhance decision-making processes, emphasizing the need for data-driven approaches.
Similarly, in public health, one models are vital for understanding the spread of diseases, like the COVID-19 pandemic. Researchers can input varying parameters to observe potential outcomes, thus guiding response strategies for healthcare systems.
In addition, the financial sector extensively employs one models to analyze economic trends. Data derived from these models assist in formulating policies that can stabilize markets and encourage growth. The benefits of applying one models in real life extend to industries like agriculture, logistics, and urban planning, showcasing their versatility and necessity in addressing real-world challenges.
Advancements driven by one models illustrate the synergy between theoretical concepts and practical applications, showcasing their influence on scientific advancements and societal progress.
Through these contributions, one models play an essential role not only in advancing research but also in providing actionable insights relevant to contemporary issues. Their importance cannot be overstated, as they bridge the gap between abstract theory and tangible outcomes.
Case Studies
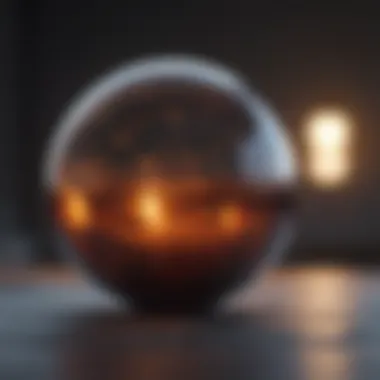
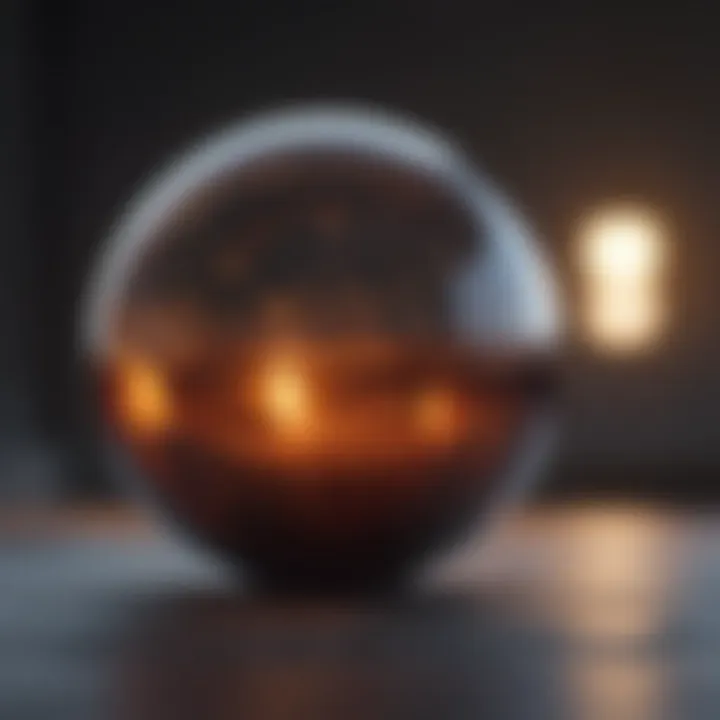
Case studies play a pivotal role in demonstrating the practical implications of one models across various scientific fields. They serve as concrete examples that highlight how theoretical principles translate into real-world applications. Through the analysis of specific instances, researchers can observe the effectiveness and limitations of one models, gaining valuable insights that inform future research and practice. In addition, case studies can illustrate innovative methodologies developed in the process of implementing one models, serving as a guide for others in the field.
The importance of this section lies in its capacity to bridge the gap between theory and practice, reinforcing concepts previously discussed. By providing evidence through actual implementations, case studies affirm the relevance of one models in addressing complex scientific challenges. They also reflect the adaptability of these models to diverse scenarios, making them integral to understanding both successes and failures within scientific inquiry.
Successful Implementations
Successful implementations of one models are found across various disciplines, illustrating the model's versatility. For instance, in ecological research, the Application of one models has enabled scientists to predict species survival under changing environmental conditions. Such studies not only confirm the predictive power of these models but also foster conservation strategies and policies.
Another example can be seen in the area of material science, where one models assist in developing new materials with desired properties. The work done by researchers at MIT in synthesizing durable polymer composites is one such successful implementation. Their approach meticulously utilized one models to analyze molecular interactions and optimize the material's performance, yielding innovative products that have wide-ranging applications.
"Successful examples show how adaptable and powerful one models can be across various scientific disciplines, ensuring broad relevance and utility."
Lessons Learned
From the successful implementations of one models, several lessons emerge that inform ongoing research and application. First and foremost, the importance of validation is clear. Models must be rigorously tested against empirical data to ensure their predictions align with reality. This process helps prevent overfitting and reinforces the modelโs credibility.
Moreover, collaboration is a recurring theme in several case studies. Interdisciplinary approaches often lead to enhanced insights, combining different fields of expertise to tackle complex problems. This is evident in climate modeling projects where meteorologists, oceanographers, and ecologists come together to create comprehensive models of climate change impacts.
Additionally, adaptability proves to be a key lesson. The ability to refine and modify a model based on new data or unforeseen variables is crucial to its success. This iterative process of modeling allows researchers to stay relevant and effective in their predictions amid changing circumstances.
In essence, case studies not only exemplify the practical applications of one models but also provide a reflective lens through which scientists can evaluate their methodologies and strategies. This feedback loop is essential to the evolution of models in any given discipline.
Challenges in One Models
In the exploration of one models, it is crucial to address the challenges that researchers and practitioners face. These challenges can often impede the effectiveness and reliability of the models. A thorough understanding of these obstacles aids in refining existing models and informs future developments. Common difficulties include the inherent limitations of current models and the potential for misapplication within different disciplines.
Limitations of Current Models
Current models are built on historical data and certain assumptions that may not hold true in every scenario. For instance:
- Data Dependence: The quality and availability of data can limit the accuracy of predictions. Often, models rely on data that may be incomplete or biased. This skews results and impacts decision-making.
- Assumptions: Many one models operate under simplifying assumptions. While these assumptions make models easier to work with, they can overlook complexities in real-world situations. It leads to results that might not be applicable to different contexts.
- Scalability: Current models may not scale well when applied to larger datasets or systems. They can break down, producing unreliable outcomes when pushed beyond their intended use.
"Understanding the limitations of current models is vital for improving their application in scientific research."
Future Prospects
Examining future prospects for one models encompasses opportunities for improvement and adaptation. As technology and data collection methods evolve, new methods will emerge.
- Advanced Computational Techniques: Machine learning and artificial intelligence are poised to enhance the predictive capabilities of one models. These techniques allow for more complex data patterns to be analyzed and understood.
- Interdisciplinary Approaches: Encouraging cross-disciplinary collaborations can lead to innovative solutions. This can help build robust models that incorporate insights from varied fields.
- Dynamic Modelling: Instead of static models, the design of dynamic and adaptive models will become increasingly important. They can adjust as new data is received, allowing for more accurate representations of real-time scenarios.
The End
The conclusion section is an essential element of this article as it synthesizes the insights gained about one models throughout the various sections. It encapsulates the significance of these models in advancing scientific thought and utility across diverse disciplines. Understanding the conclusions drawn is pivotal for interpreting the practical implications of one models in research and real-world applications.
Summarizing Key Findings
Throughout the article, several key findings emerge regarding the role and effectiveness of one models. These findings include:
- Interdisciplinary Relevance: One models have proven to be versatile tools across different scientific fields, demonstrating their ability to integrate various types of data and methods. This has allowed for richer insights compared to more isolated approaches.
- Methodological Rigor: The methodologies employed in the formulation and validation of one models have underscored the importance of robust data collection techniques and validation processes. This ensures that the models remain reliable and useful in practical situations.
- Innovative Applications: Case studies discussed highlight the successful application of one models in solving complex scientific problems. These case studies elucidate how one models can lead to tangible advancements in biological, chemical, and earth sciences.
In general, one models serve as a bridge between theoretical concepts and practical applications, ultimately enriching both academic and applied research.
Implications for Future Research
The exploration of one models opens various avenues for future research. Some implications for further study include:
- Enhancement of Model Accuracy: Future research can focus on refining the mathematical frameworks to enhance model accuracy. This may involve integrating new data sources or developing more sophisticated algorithms that better represent complexities found in real-world scenarios.
- Broader Application Scenarios: Researchers should consider extending the application of one models to encompass new fields or issues, particularly in emerging areas like climate change, data science, or health sciences. This can lead to novel insights and solutions.
- Collaboration Across Disciplines: There is a growing need for interdisciplinary collaboration which can lead to innovative methodologies that combine strengths from various fields. This can also enhance the robustness of findings derived from one models.