Cardiovascular Disease Datasets: Research and Healthcare Insights
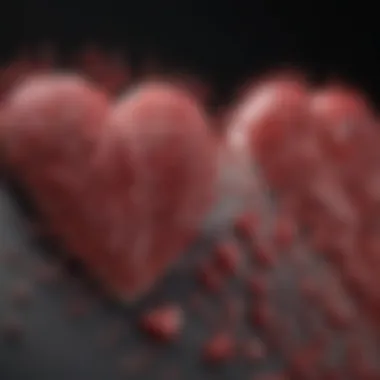
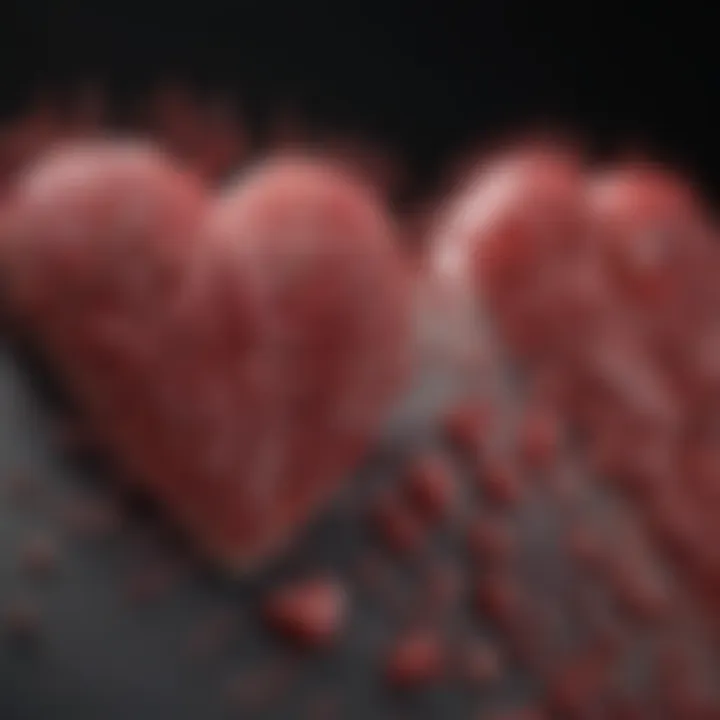
Intro
Cardiovascular diseases are among the leading causes of death globally. As researchers and healthcare professionals continue to navigate this complex landscape, the need for robust data becomes ever more critical. This article facilitates a thorough insight into how cardiovascular disease datasets can influence both research and healthcare strategies. By focusing on various aspects of these datasets—from their structure and collection to implications on treatment outcomes—we aim to unearth in-depth understanding.
Healthcare systems are increasingly reliant on data-driven approaches. Through careful analysis, we can uncover trends, assess risks, and evaluate treatment efficacy, ultimately improving patient outcomes. This article explores these nuances with the intention of fostering a deeper engagement in cardiovascular research.
Overview of Research Topic
Brief Background and Context
The cardiovascular disease dataset is not a singular entity but rather a composite of diverse data sources, including clinical trials, hospital records, and longitudinal studies. Historically, the focus was primarily on anecdotal findings and smaller studies. However, the landscape has evolved with technology enabling comprehensive data collection and analytical methods. This change in perspective reflects a shift toward more empirical evidence in medicine, laying the groundwork for efficient interventions and therapies.
Importance in Current Scientific Landscape
In today's scientific arena, knowledge is power, particularly when intertwined with data. Cardiovascular research demands not just any data, but high-quality, organized datasets that offer insights into trends and patient behaviors. Such datasets are pivotal in shaping public health policies and clinical practices.
A well-structured cardiovascular dataset can serve multiple functions:
- Risk Assessment: It allows for more precise identification of at-risk populations.
- Treatment Evaluation: Enables evaluations of the effectiveness of treatments over time.
- Public Health Strategy: Helps in formulating policies based on documented trends.
To highlight the weight of this type of data, consider the following important point:
"The richness of cardiovascular datasets can transform not just individual patient care but also influence broader societal health initiatives."
Methodology
Research Design and Approach
The foundation for research surrounding cardiovascular datasets relies heavily on meticulous design. Exploratory research is often intertwined with longitudinal studies, aiming to connect immediate findings with long-term health outcomes. Mixed-method approaches allow for qualitative insights alongside quantitative data, providing a well-rounded view of the health landscape.
Data Collection Techniques
Data collected for cardiovascular studies typically falls into a few main categories. These include:
- Clinical Trials: Information derived directly from controlled studies, ensuring a level of rigor and reliability.
- Electronic Health Records: A wealth of information that captures patient histories, treatments, and outcomes in real-time.
- Wearable Technology: Increasingly, data from personal health monitors like heart rate trackers are included, providing valuable real-time health metrics.
Prolusion to Cardiovascular Disease
Cardiovascular disease (CVD) remains a leading global cause of mortality, commanding attention from both the medical community and researchers alike. The nuances of this topic stretch far beyond basic statistics; it's a web of risk factors, biological mechanisms, and social determinants that intertwine in complex ways. Understanding these diseases is essential not only for individual health but also for public health strategies and healthcare policy. This section serves as a critical starting point, laying the groundwork for the discussion on cardiovascular disease datasets that follow.
Definition and Scope
In the simplest terms, cardiovascular disease encompasses a range of conditions affecting the heart and blood vessels. This includes coronary artery disease, heart rhythm problems (arrhythmias), and even congenital heart defects. The scope is broad: from heart attacks to strokes, the implications of CVD touch many lives across different demographics.
A key point to consider is that cardiovascular disease does not discriminate; it affects people of all ages, genders, and ethnic backgrounds. Thus, when we talk about defining and understanding this disease, we're not just looking at clinical definitions; it’s critical to include socioeconomic factors, lifestyles, and regional health disparities.
Prevalence and Impact
The prevalence of cardiovascular disease paints a stark picture. According to the World Health Organization, CVD accounts for approximately 32% of all global deaths. This staggering number emphasizes the importance of addressing cardiovascular health through various interventions.
- Risk Factors: Factors such as smoking, saturated fat intake, high blood pressure, and physical inactivity significantly increase the likelihood of developing CVD. Understanding these risks is imperative for shaping educational programs aimed at prevention.
- Economic Burden: The financial impact is no less alarming. CVD imposes a hefty burden on healthcare systems, leading to increased medical expenses and lost productivity. The societal fallout from morbidity and mortality incurs costs that can affect economies, often resulting in stagnation in regions heavily affected by heart disease.
"Addressing cardiovascular disease is not just a health issue but a unique challenge that ties into economic stability and social equity."
The pervasive nature of CVD necessitates a proactive approach in both research and healthcare. By exploring cardiovascular disease datasets, we can glean insights into this multifaceted issue, paving the way for effective treatments and preventive measures. This sets the stage for our next discussions focused on the datasets that illuminate these crucial elements.
Understanding Cardiovascular Disease Datasets
Understanding cardiovascular disease datasets is crucial in the context of advancing both research and healthcare strategies. These datasets serve as a foundation upon which various analyses and interpretations can be built, ultimately leading to better patient outcomes. As the healthcare ecosystem becomes increasingly data-driven, comprehending the structure and significance of these datasets is vital for practitioners, researchers, and policy makers alike.
Effective understanding of these datasets allows stakeholders to unveil trends, assess risks, and develop treatment protocols backed by empirical evidence. It also facilitates cross-disciplinary collaborations, thus enriching the research landscape. By delving into the specifics of what constitutes a dataset, we can appreciate the nuances that differentiate each type and their respective contributions to cardiovascular health knowledge.
What is a Dataset?
A dataset is essentially a collection of related data points, organized in a format that allows easy access, analysis, and interpretation. In the realm of cardiovascular diseases, datasets typically consist of patient information, clinical metrics, and outcomes, among other variables. They're not just simple lists, but rather intricate compilations designed to exhibit trends and outcomes across various demographics, geographies, and treatment modalities.
The strength of a dataset lies in its ability to enable researchers to draw correlations, identify patterns, and make predictions that can significantly impact patient care and clinical practices. A well-structured dataset can be an invaluable asset for generating insights that influence public health policy.
Types of Cardiovascular Datasets
Different types of cardiovascular datasets cater to various aspects of research and clinical practice. Each type has unique features and characteristics, adding to the richness of the dataset landscape. Here, we take a closer look at three primary categories:
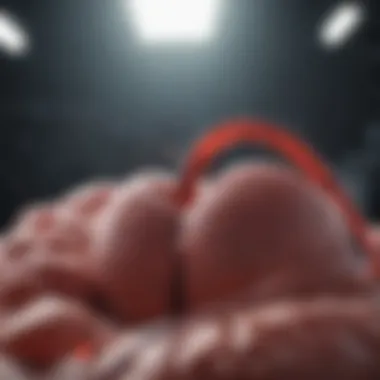
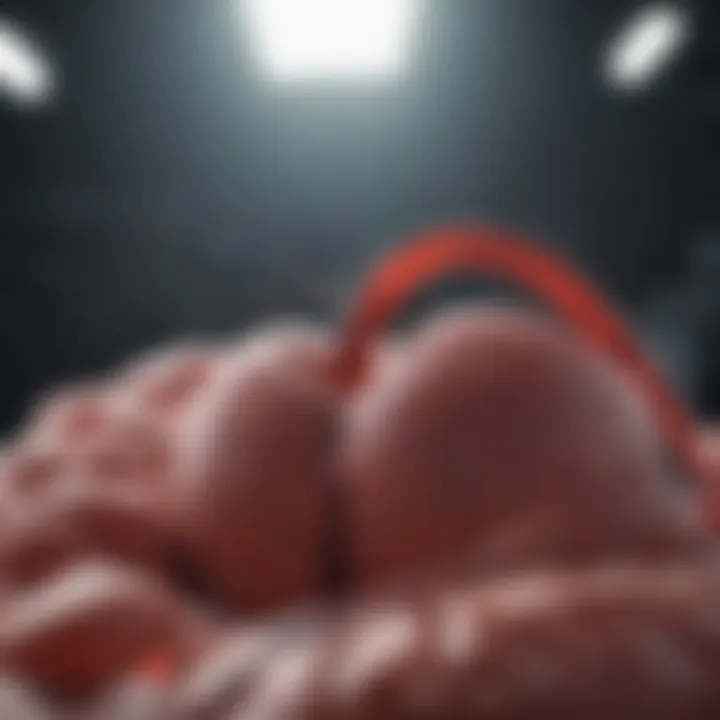
Clinical Datasets
Clinical datasets encompass comprehensive patient records that include medical history, diagnostic data, treatment plans, and outcome measures. They are vital for understanding individual patient journeys and treatment efficacy. One noteworthy characteristic of clinical datasets is the inclusion of real-world clinical measurements, which provides immediate relevance for healthcare providers.
These datasets are popular for their direct applicability to patient care and their role in clinical research. They facilitate outcomes studies and cohort research that can lead to evidence-based guidelines. However, they may sometimes be limited in terms of geographical diversity or sample size, which could influence the generalizability of findings.
Epidemiological Datasets
Epidemiological datasets focus on the study of health-related states in populations. They aim to uncover patterns and determinants of cardiovascular diseases across various demographics and geographic areas. A key characteristic is that they often integrate data from multiple sources, including surveys, public health records, and community assessments.
These datasets are beneficial for understanding the prevalence and risk factors associated with cardiovascular diseases. They can inform public health initiatives effectively. However, epidemiological datasets may face challenges such as reliance on self-reported data, which can introduce biases and inaccuracies.
Genetic Datasets
Genetic datasets delve into the inheritance patterns and genetic predispositions that may contribute to cardiovascular diseases. They often include genomic, transcriptomic, and epigenomic data collected from diverse populations. A striking feature is their potential to provide insights into personalized medicine, paving the way for tailored treatment approaches.
These datasets have garnered popularity in recent years, especially with the surge of interest in genomics. However, one drawback is the complexity involved in analyzing genetic data, which may require advanced computational skills and tools. This can sometimes hinder accessibility for researchers and practitioners not well-versed in bioinformatics.
Understanding the different types of cardiovascular datasets not only enriches our knowledge but also facilitates better decision-making in healthcare and research. Each type contributes uniquely to the overarching goal of improving cardiovascular health outcomes.
Data Collection Methods
Data collection is the backbone of any research endeavor, especially when it comes to understanding cardiovascular diseases. Without reliable and accurate data, the research can't pave the way for better treatment and preventive measures. It’s a bit like trying to build a house without a solid foundation; all it takes is one strong gust of wind to knock it down. Here, we will break down the primary sources of data and standardization practices that ensure the data collected serves its purpose effectively.
Sources of Data
Hospitals and Clinics
Hospitals and clinics are often the first stop for data collection in cardiovascular research. They serve as a major source of patient information because they gather a wealth of clinical data daily. A real window into patient conditions is what these institutions provide. The key characteristic of hospitals is their frontline interaction with patients; they observe and record numerous real-time cases where heart-related illnesses are treated. This direct access to patient care allows researchers to analyze various treatment outcomes and complications.
One unique feature of hospitals is their access to electronic health records (EHR). These systems allow quick aggregation of vast amounts of data, from lab results to patient histories. The advantage is clear: this data can provide a comprehensive view of patient outcomes over time. However, there are disadvantages too. Privacy concerns often loom over the data collected, making it essential to ensure patients’ confidentiality while collecting valuable information. The potential for incomplete data from diverse hospital units adds another layer of complexity.
Public Health Organizations
Public health organizations play a crucial role by providing population-based data which is vital for epidemiological studies. They collect information from a wider net, incorporating data from various communities. This broad scope is particularly beneficial for understanding national trends in cardiovascular diseases. Public health organizations serve not just as data collectors, but also as interpreters, analyzing how socio-economic factors influence health outcomes.
The standout feature of these organizations is their ability to conduct epidemiological surveys. They can reach vast populations, leading to datasets that reflect the general public’s health rather than just a clinical subset. A significant advantage here is the power to identify risk factors, trends, and correlations across demographics. However, the downside is the potential for lack of granularity; these datasets might not delve into individual treatment details, limiting the depth of understanding for specific patient interactions.
Research Institutions
Research institutions are often at the cutting edge of cardiovascular data collection, employing both quantitative and qualitative methodologies to gather data. They contribute significantly to creating specialized datasets, often designed around specific hypotheses or research questions. These institutions can focus on long-term studies, allowing for an in-depth look at disease progression.
The hallmark of research institutions is their collaboration with various entities, combining clinical data from hospitals with epidemiological data from public health organizations. This synergy can lead to rich datasets that encompass multiple aspects of cardiovascular health. However, one major consideration is resource availability. Research institutions may have access to advanced technology and methodologies, yet funding constraints can hinder data collection efforts.
Standardization of Data Collection
Standardization in data collection is vital to ensure that the data is reliable and comparable across different studies and settings. Without standard protocols, different researchers might collect data in ways that do not align, leading to gaps and inconsistencies in findings.
Standard protocols include:
- Defining terms and measurements consistently.
- Using uniform templates for data entry to minimize errors.
- Training personnel involved in data collection to follow procedures diligently.
By sticking to standardized methods, researchers can facilitate collaboration, comparing their results more efficiently. This, in turn, fosters a more cohesive understanding of cardiovascular disease across various populations and locations, ultimately leading to better healthcare approaches.
Data collection methods shape the foundation of cardiovascular research, as they dictate the accuracy and reliability of subsequent analyses and conclusions.
Data Structure and Management
The structure and management of data play a crucial role in the efficacy of cardiovascular disease research. Proper management ensures data is not only accessible but also reliable and actionable. As we dive deeper into the vast sea of information pertaining to heart health, recognizing how data is organized can make all the difference in clinical outcomes and research validations. The way data is structured underpins the ease of analysis and can provide profound insights into various treatment methodologies and risk factors prevalent in cardiovascular diseases.
Database Design Principles
When embarking on the journey of creating a coherent data structure, one must pay heed to a few core principles that guide database design. A well-structured database serves as the backbone for accurate data collection and analysis. Here are some essential principles to consider:
- Normalization: This process involves organizing data to minimize redundancy. It helps in maintaining the integrity of the dataset, reducing anomalies that could skew research findings.
- Schema Design: A clear schema defines how data is stored, categorized, and related. Understanding the relationships among different types of data entities can illuminate important trends and patterns.
- Indexing: Implementing indices speeds up data retrieval processes, which is vital when dealing with large datasets. Quick access to pertinent information enhances the overall analytical capabilities of researchers.
Paying attention to these principles not only streamlines the data management process but can also drastically improve the potential for meaningful insights into cardiovascular health. Additionally, a well-designed database can facilitate better collaboration among researchers, allowing for shared insights and combined efforts in tackling the challenges posed by cardiovascular diseases.
Data Quality Assurance
Ensuring the quality of data collected is non-negotiable in the realm of healthcare research. Data quality assurance encompasses a set of protocols aimed at maintaining high standards throughout data collection, storage, and analysis. Here are some key components of effective data quality assurance:
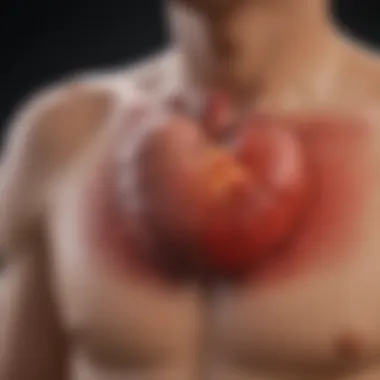
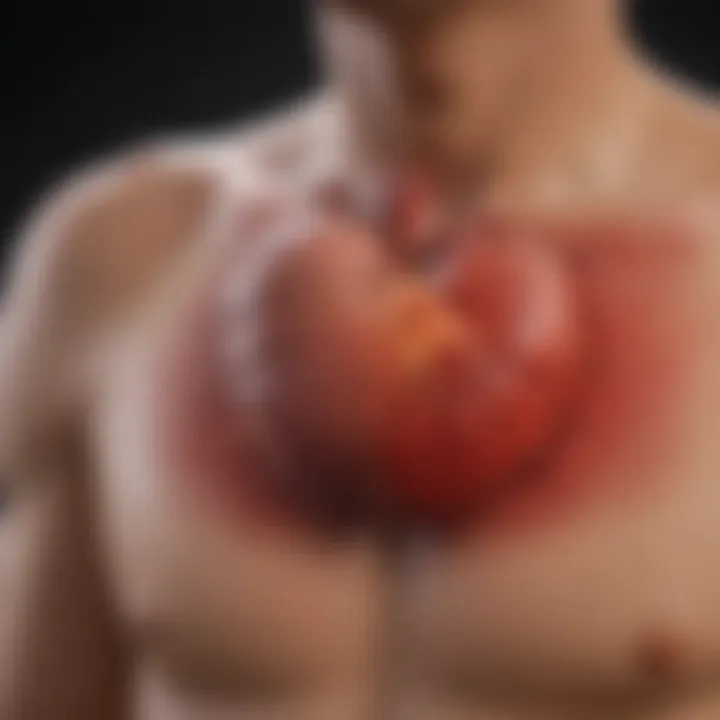
- Accuracy: Data must represent real-world situations accurately. For example, consider a dataset of patients with heart disease. If the data are misentered, it would lead to erroneous conclusions about treatment efficacy.
- Completeness: Missing data can create significant gaps in research outcomes. Regular audits should be undertaken to ensure that the dataset is comprehensive and that all necessary variables are included.
- Consistency: Data must remain consistent across various datasets. A lack of consistency can arise from different data entry methods or varied definitions of health metrics.
Maintaining high data quality ultimately aids in the reliability of research outcomes. An accurate, complete, and consistent dataset directly affects the risk assessments and treatment strategies developed for cardiovascular disease management.
"Data quality is crucial; accurate data leads to effective healthcare decisions."
In summary, understanding data structure and its management can profoundly influence the outcomes in cardiovascular research. By adhering to the principles of database design and ensuring the integrity of data through strict quality assurance protocols, one can unlock the potential that accurate data holds for advancing the field of cardiovascular health.
Data Analysis Techniques
In the realm of cardiovascular disease research, data analysis techniques hold significant importance, acting as the backbone of substantial advancements in understanding, prevention, and treatment. These techniques enable researchers to sift through abundant data in actionable ways, leading to enhanced insights that can directly influence patient outcomes and healthcare strategies.
Statistical Methods
Statistical methods form the foundation for data analysis in cardiovascular research. By employing rigorous statistical frameworks, researchers can uncover patterns and relationships that might otherwise go unnoticed. Techniques such as regression analysis help to establish the influence of different risk factors on heart health, thereby enabling clinicians to identify high-risk populations effectively.
Consider the application of baseline and longitudinal data analysis in clinical studies. This approach offers a comprehensive view of changes in patient conditions over time, further refining risk assessment strategies. Additionally, using survival analysis can shed light on the prognosis of patients with cardiovascular disease, informing decisions on treatment protocols and potential interventions.
Machine Learning Applications
In recent years, the integration of machine learning into data analysis has transformed how cardiovascular datasets are utilized. Machine learning offers advanced computational techniques that can identify intricate patterns in large datasets that traditional statistical methods might miss.
For instance, neural networks can predict patient outcomes based on a variety of inputs such as age, lifestyle factors, and clinical history. This capability not only enhances predictive accuracy but also supports personalized medicine, where treatments are tailored to individual patient profiles. Furthermore, clustering algorithms can segment populations according to risk factors, which helps in designing targeted prevention strategies.
"Machine learning is not just about algorithms; it's about deriving actionable insights from vast pools of data."
Predictive Analytics in Cardiovascular Research
Predictive analytics stands as a key component in cardiovascular disease research, facilitating the anticipation of future events and outcomes. By merging statistical methods, machine learning, and historical data, researchers can forecast risks associated with cardiovascular conditions, enabling proactive management of patient care.
Techniques like risk scoring models assess the likelihood of events such as heart attacks or strokes based on comprehensive datasets. The use of historical data patterns aids in creating robust predictive models, which can significantly enhance clinical decision-making. Commonly used tools include the Framingham Risk Score and the ASCVD Risk Estimator, which help in quantifying the risk levels and guiding early interventions effectively.
In summary, data analysis techniques are not merely academic exercises; they have profound real-world implications that can shape clinical practices and healthcare outcomes. As the field evolves, embracing novel computational approaches alongside established methods will be crucial in addressing the ongoing challenges associated with cardiovascular diseases.
Implications for Healthcare and Research
The intersection of cardiovascular disease datasets and their implications for healthcare and research is both critical and complex. Understanding these implications can significantly enhance how medical professionals assess risks, manage treatments, and ultimately improve patient outcomes. The foundation of such advancements rests on meticulous data collection, quality management, and robust analytical approaches.
Risk Assessment and Management
Risk assessment is a key aspect of cardiovascular healthcare. It involves identifying individuals at high risk for cardiovascular events, such as heart attacks or strokes. Data derived from various cardiovascular disease datasets allow healthcare professionals to uncover patterns and trends that inform risk factors. For example, studies may link specific demographic details or lifestyle choices to increased risk, helping clinicians to tailor prevention strategies accordingly.
When it comes to managing these risks, datasets provide a wealth of statistical data that improve predictive modeling. By applying these models, health practitioners can predict which patients may experience adverse outcomes based on their specific health profiles. Moreover, integrating genetics and biomarkers into risk assessments helps in refining these predictions, making them more precise and actionable.
"Data-driven decision-making in cardiovascular care shifts focus from reactive to proactive strategies, reducing incidence rates and enhancing life quality."
In practice, the management of cardiovascular risk hinges on regular assessment of patients using tools developed from dataset analysis. Healthcare systems that use predictive analytics are often able to prioritize interventions for high-risk patients, ensuring timely medical attention when necessary.
Guiding Treatment Strategies
Further downstream, the implications of cardiovascular disease datasets profoundly influence treatment strategies. When healthcare providers have access to comprehensive datasets, they can analyze previous treatment outcomes, leading to evidence-based recommendations. This is especially relevant in understanding how certain medications work across various demographics and comorbidities.
Tailoring treatment plans based on data allows for a more personalized approach. For instance, patients with certain genetic markers may respond better to specific drugs, and having that data can steer doctors away from ineffective treatments and towards more suitable options.
In addition, researchers can leverage datasets to evaluate new therapeutic approaches against established ones, identifying trends that indicate effectiveness. The continuous feedback loop created by employing such data enhances the overall efficacy of cardiovascular care.
Lastly, it is important to note the ethical dimensions surrounding these practices. While datasets provide valuable insights, they must be handled with care, ensuring patient privacy and informed consent are prioritized throughout the research and treatment processes.
Integrating the implications of these datasets into actual healthcare practices not only streamlines processes but also builds a framework for more effective cardiovascular disease management. Academic research, clinical trials, and real-world applications thus coalesce around a shared goal — enhancing patient care through informed, data-driven insights.
Ethical Considerations in Data Usage
In the field of cardiovascular disease research, the importance of ethical considerations cannot be overstated. The handling of data is governed by a set of principles that aim to protect individuals while fostering beneficial research. These considerations are pivotal when we dive into sensitive medical information, touching the lives of numerous patients and their families. Practitioners and researchers alike must grapple with the responsibility of maintaining the integrity of their findings while upholding the respect and dignity of those whose data fuels their studies.
The relevance of ethical measures extends beyond just compliance with laws and regulations; they foster trust between patients and healthcare systems, an essential component in gathering reliable data for cardiovascular research. The success of research hinges on participants feeling safe and secure in sharing their information. Such trust can lead to comprehensive participation which, in turn, enriches datasets, enhancing the accuracy and applicability of research findings.
Privacy and Confidentiality
Privacy and confidentiality are keystones in the realm of ethical data usage. With the rise of digital record-keeping and the digitization of medical information, personal data can easily be accessed. This digital age brings forth serious concerns. Protecting patient identities and their medical history is paramount. Notably, a breach can lead to not just legal ramifications but a significant erosion of trust between healthcare providers and patients.
"When individuals feel their data is handled with care, they are more likely to participate in studies that can ultimately help save lives."

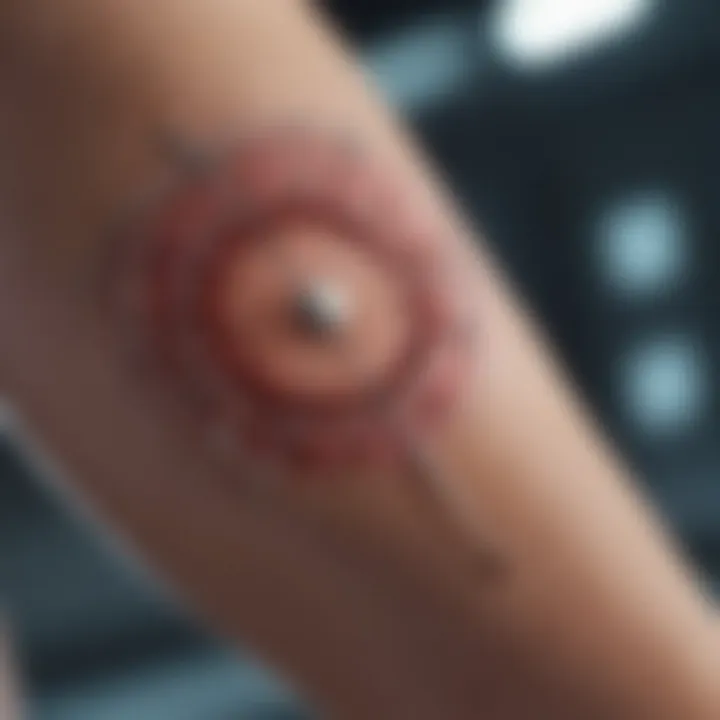
To bolster privacy efforts, data anonymization techniques are often employed. Such methods include:
- Removing identifiable information from datasets
- Aggregating data to present trends without exposing individual identities
- Using encryption to safeguard sensitive information during transmission
These measures ensure that while researchers have the data they need to perform meaningful analysis, the anonymity of patients remains intact.
Informed Consent
Informed consent stands as another foundational pillar in ethical data utilization. This process involves ensuring that participants are fully aware of how their data will be used, and they have freely agreed to participate in research projects. For cardiovascular research, this is particularly vital. Patients need to understand the potential benefits and risks tied to their participation.
Obtaining informed consent involves several critical aspects:
- Clear Communication: Researchers must present information in a way that is easily understandable, eliminating jargon and technical terms that could confuse participants.
- Voluntary Participation: Individuals should have the freedom to decline or withdraw from a study at any stage without any repercussions to their healthcare.
- Understanding of Usage: Participants should be made aware of the possible implications of their data being used in studies, ensuring they grasp how their contributions can lead to medical advancements in cardiovascular health.
By cultivating an environment of transparency, the research community not only respects participants' rights but also enhances the reliability of their findings. Engaging ethically with data might appear straightforward, yet the complexity of human emotions and privacy must not be underestimated.
Challenges in Cardiovascular Disease Data Management
In today's world, where data reigns supreme, managing the intricacies of cardiovascular disease datasets emerges as a vital yet daunting task. The crux of this challenge lies in the disparate nature of data sources and the vast volume of information generated daily. With research continuously evolving, understanding the hurdles in data management can profoundly impact both clinical and research outcomes. Each obstacle not only complicates data handling, but can also skew results, leading to misguided assumptions that may negatively affect patient care.
Data Integration Issues
One of the most significant challenges in managing cardiovascular datasets is integrating data from different sources. Often, data arises from various hospitals, clinics, and external research institutions. Each entity may use different data formats, categorization methods, and reporting standards, resulting in data silos. When these silos are not harmoniously combined, it can lead to a lack of cohesion in research findings.
- Inconsistent data formats can impede the ease of analysis. For instance, if one dataset records the age in years while another provides it in months, researchers might end up making incorrect assumptions during comparative studies.
- Missing data is another concern. Whether due to a patient not disclosing medical history or a data entry oversight, gaps in information can disrupt the big picture. This often leads to the exclusion of individuals from analyses, skewing the understanding of disease prevalence and treatment effects.
These integration issues can also create unnecessary frustrations for researchers who seek to produce actionable insights. Ultimately, without a streamlined approach to data management, the potential of cardiovascular datasets remains underutilized.
Addressing Bias in Datasets
Bias in data can rear its head in various forms, impacting the legitimacy of conclusions drawn from cardiovascular research. A primary concern is the selection bias that arises when the data collected is not representative of the broader population. For instance, if a study primarily gathers data from urban populations, rural health disparities may be overlooked, leading to erroneous conclusions about risk factors or treatment effectiveness.
To mitigate this bias, researchers can adopt several strategies:
- Diverse Sampling: Efforts should be made to include participants from various backgrounds, including different geographical locations, socio-economic statuses, and ethnicities. This diversity can provide a more rounded view of cardiovascular disease trends.
- Regular Audits: Researchers should periodically review their datasets for potential biases. This can include scrutinizing the demographics of participants and adjusting the samples if necessary to ensure broader representation.
- Transparency: Communicating the potential limitations in datasets is crucial. It allows other researchers and practitioners to understand the context of findings, promoting informed decisions in clinical settings.
"A data set is only as good as the understanding of its limitations. Embracing transparency encourages more meaningful interpretations in cardiovascular research."
Addressing bias is not just about improving scientific accuracy; it's vital for patient safety. When biases persist unchecked, the strategies derived from research may not only mislead practitioners but also endanger patients. Ultimately, successfully managing cardiovascular disease data demands vigilance in tackling both integration issues and potential biases—ensuring that research is both precise and representative, paving the way for better healthcare outcomes.
Future Directions in Cardiovascular Data Research
The landscape of cardiovascular disease research is constantly evolving. Future directions in this field hinge on not only technological advancements but also on creative interdisciplinary collaboration. Shifting the focus to emerging technologies and their integration is imperative for enhancing data utilization in predicting outcomes and tailoring patient-specific interventions. Implementing forward-thinking strategies in research can open new avenues to improve cardiovascular healthcare.
Emerging Technologies
In the realm of cardiovascular data, new technologies are at the forefront, revolutionizing how researchers collect, manage, and analyze data. For instance:
- Wearable devices: Tools such as smartwatches and fitness trackers provide real-time data on heart rates and activity levels. The data gathered can help in monitoring patients more effectively, allowing for timely interventions based on activity trends.
- Artificial Intelligence (AI): Through machine learning algorithms, AI enables researchers to identify patterns and correlations within extensive datasets far more efficiently than traditional methods. This can aid in predicting potential cardiovascular events and personalize treatment plans.
- Telemedicine: With the rise of remote health consultations, telemedicine is facilitating the collection of patient data from the comfort of home. This not only includes essential health metrics but also provides insights into patients’ lifestyles and socio-economic factors.
Implementing such technology actively contributes to the precision medicine movement, fostering tailored individual healthcare approaches that will greatly improve patient outcomes.
Interdisciplinary Approaches
It's critical to understand that addressing cardiovascular disease is not solely a medical endeavor; it requires a holistic approach. Interdisciplinary collaboration not only brings diverse sets of expertise together but also enhances the richness of the datasets and their analyses. Here are some key elements:
- Collaboration between health professionals and data scientists: Engaging data experts in clinical discussions can lead to more pertinent and effective analytical frameworks, ensuring data collection is relevant and actionable.
- Involving social scientists: Understanding the social determinants of health—such as income, education, and access to care—can provide deeper insights into patient behaviors and cardiovascular risk factors.
- Cross-sector partnerships: Collaboration between academic institutions, hospitals, and public health organizations can facilitate a better flow of information and resources, ultimately leading to greater innovations in research.
"By merging various fields of expertise, we can uncover critical relationships that are often missed in siloed approaches."
The End
The exploration of cardiovascular disease datasets is vital to both research and clinical practice. As recapped in this article, these datasets do not just serve as numbers and statistics; they represent real human experiences and outcomes. The key insights gleaned from this analysis include the significance of effective data collection and management strategies as activities that ensure rigorous studies are conducted, ultimately leading to improved patient therapies and healthcare solutions.
Summarizing Key Insights
Before we wrap things up, let’s highlight some of the key points discussed:
- Diverse Types of Datasets: A variety of datasets exist, each tailored to different aspects of cardiovascular health, from clinical datasets focusing on patient outcomes to epidemiological datasets that provide insight into population health trends.
- Data Analysis Importance: Employing statistical and machine learning methods has been highlighted. These techniques can unveil patterns and potential risk factors, guiding clinicians in better identifying high-risk patients and improving treatment protocols.
- Ethical Responsibilities: As the volume of data grows, so does the need for rigorous ethical considerations. Protecting patient privacy and ensuring informed consent is not just a requirement; it is fundamental to gaining public trust in research.
- Integration and Bias Challenges: The problems with integrating various datasets and tackling inherent biases in the data are real hurdles that need careful attention. The reliability of conclusions drawn from data-heavy studies hinges on addressing these issues.
Call to Action for Researchers
For researchers, the findings present a clarion call. There is a pressing need to continuously push the envelope on how data can be utilized in cardiovascular research.
- Collaboration Across Disciplines: Interdisciplinary collaborations will likely prove fruitful. By merging insights from different fields—be it genetics, public health, or computational biology—researchers can design holistic approaches that enhance the understanding of cardiovascular diseases.
- Advocate for Transparency: Researchers should also advocate for greater transparency in data usage. Sharing datasets responsibly can lead to new breakthroughs and reduce duplicative efforts across the research community.
- Engage with Ethical Frameworks: Lastly, as the landscape of data in healthcare evolves, so must the ethical frameworks guiding research. Getting involved in discussions surrounding ethical standards and best practices is essential for fostering trust and integrity in research outcomes.
In summary, cardiovascular disease datasets are more than mere repositories of information; they are the keys to unlocking future improvements in healthcare. The insights gleaned from them can pave the way for innovative therapies and more informed public health policies. Researchers play a crucial role in this ongoing narrative, and their engagement is essential as we strive for progress in understanding and combating cardiovascular diseases.