Analyzing and Presenting Data: Strategies and Techniques
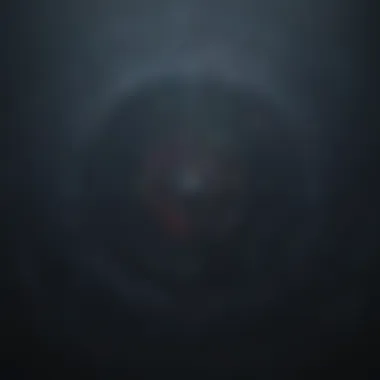
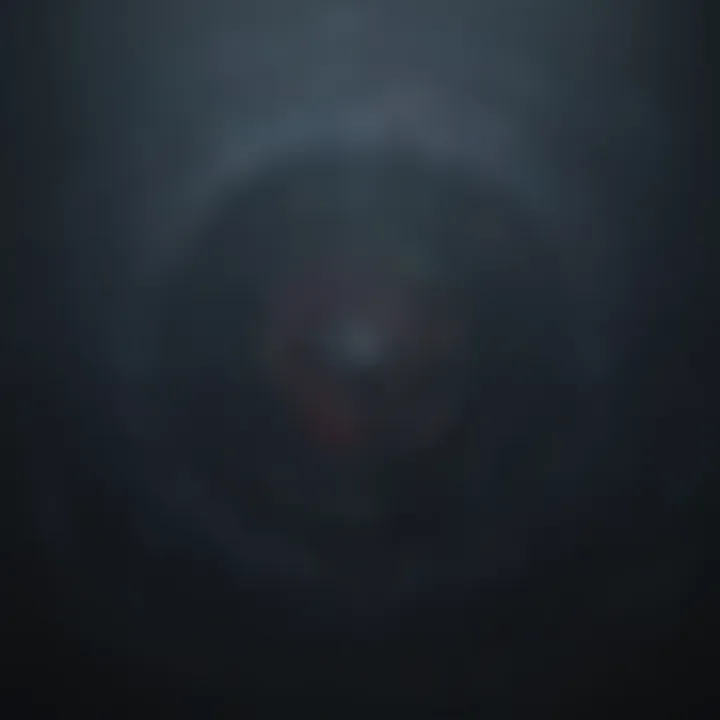
Intro
The fast-paced digital world of today is filled with data—various forms, and endless streams. As students, researchers, educators, and professionals scurry to make sense of this rich tapestry of information, the ability to effectively analyze and present data takes center stage. Understanding how to transform raw numbers into insightful narratives is not just a skill; it is an art form that can propel decisions and innovations across fields.
In this exploration of data analysis and presentation techniques, we will delve into critical elements that define a successful approach. From the initial stages of gathering relevant data to ensuring its integrity, and ultimately presenting findings in a way that resonates, each segment plays its part in painting the complete picture.
So, let’s roll up our sleeves and dive in.
Understanding the Role of Data in Research
Data serves as the lifeblood of research, acting as the foundation upon which conclusions are drawn and insights are crafted. Without data, research would be little more than a shot in the dark. Within the vast expanse of scientific inquiry, understanding the role of data goes beyond mere collection; it encompasses its significance in discovery, validation, and communication.
The Importance of Data
First off, the significance of data cannot be overstated. At its core, data can reveal patterns and truths about our world, encapsulating everything from population trends to the impact of climate change. This predictive power helps researchers, educators, and professionals alike make informed decisions. The ability to draw reliable conclusions hinges directly on the quality and integrity of the data collected. Bad data can lead to skewed results, leading researchers down the wrong path. Thus, recognizing its importance is paramount to the success of any research endeavor.
Types of Data in Scientific Inquiry
In scientific inquiry, data can be broadly categorized, impacting how we approach the analysis and interpretation. It’s crucial to understand these categories to choose the right methodology and ultimately leverage the data effectively.
Quantitative vs. Qualitative Data
Quantitative data refers to information that can be measured and expressed numerically. For example, a study measuring the average temperature increase over time uses numerical values that can be subjected to various statistical analyses. This type of data is renowned for its objectivity and ability to facilitate comparisons, which is vital in scientific research.
On the flip side, qualitative data encompasses descriptive information that cannot be easily quantified. Think of interviews or open-ended survey responses, which provide rich insights into people’s thoughts and feelings. While it lacks the numerical rigidity of quantitative data, it offers a depth of understanding that numerical data often cannot. Therefore, the significance comes from how one complements the other. Their combination enables researchers to paint a fuller picture.
Primary vs. Secondary Data
When we talk about primary data, we are discussing data collected firsthand for a specific purpose. This is often regarded as the gold standard, as it is original and directly related to the research question being addressed. For instance, conducting an experiment to measure the effectiveness of a new drug results in primary data. Its advantage lies in its specificity and reliability; however, it can be time-consuming and costly to gather.
Conversely, secondary data is information collected by someone else for a different purpose. Examples include census data or research articles that present findings from prior studies. While secondary data can provide context and breadth in research, it may not always perfectly align with the research question. Researchers must be vigilant regarding the source, as the validity of secondary data can vary significantly. The challenge lies in reconciling the differences between primary and secondary data for a comprehensive analysis.
Effective data usage requires thoroughly understanding the types and context of data to draw sound conclusions.
Data Collection Methods
Data collection methods lay the foundation for effective data analysis and presentation. The reliability of conclusions drawn from data is highly dependent on how, where, and when that data has been gathered. In scientific research and decision-making, the choice of data collection method can significantly impact the integrity and applicability of the findings.
Each method comes with its own set of benefits and considerations. For instance, utilizing surveys can result in a rich dataset if tailored correctly, while experimental designs can yield detailed insights into causal relationships. The broader aspect of employing these collection methods involves understanding the context of the research questions and objectives. Adjusting strategies based on these factors leads to more targeted and meaningful data results.
Surveys and Questionnaires
Surveys and questionnaires stand as versatile tools in the data collection arsenal. They allow researchers to gather information from a wide audience quickly and efficiently, accommodating various topics from opinions to behaviors. One of the appealing aspects of these tools is their ability to reach a diverse audience, enabling the collection of both qualitative and quantitative data.
The advantages of using surveys are many: they can be conducted online, sent via email, or even delivered in person. This flexibility provides researchers the option to choose the method that best suits their audience, minimizing barriers to engagement. Moreover, well-crafted questions can elicit responses that reveal underlying trends and patterns.
However, crafting effective surveys requires careful attention. Questions need to be clear to avoid misunderstandings, leading to misleading data. Bias in question phrasing can also influence responses, making it crucial to test surveys before widespread distribution. A common pitfall is asking too many leading questions or failing to include neutral options. Hence, striking a balance is essential for valuable and reliable data.
Experimental Design
Experimental design refers to the structured approach employed when conducting research, particularly in determining cause-and-effect relationships. It can be pivotal in yielding robust, insightful results, serving to clarify the workings of complex phenomena.
Controlled Experiments
Controlled experiments focus on isolating the variable under study to observe the effects on a dependent variable. This structured method is often seen as a gold standard in research because it allows for high reliability and reproducibility of results. By controlling extraneous variables, researchers can draw firmer conclusions about causal relationships.
A key characteristic of controlled experiments is the manipulation of independent variables while observing changes in the dependent variables. This enables researchers to make informed decisions about the relationship and potential impact of different aspects within the study. However, controlled experiments may sometimes lack ecological validity, as they are often conducted in artificial settings that may not represent real-life situations well.
Field Studies
Field studies, on the other hand, examine phenomena in their natural context, offering a more holistic view. Investigating subjects in their usual environment can unveil nuances that might be overlooked in controlled experiments. These studies can lead to rich, qualitative insights that resonate more with real-world scenarios.
A significant advantage of field studies is their capacity to capture authentic behavior, which often translates to data that reflect genuine circumstances. However, they can be susceptible to uncontrolled variables that researchers need to account for, complicating the interpretation of results. Thus, while field studies can provide depth and contextual awareness, they require careful consideration of external influences.
Observational Techniques
Observational techniques encompass a range of methods through which researchers can gather data without direct interaction or manipulation of the subject matter. This approach is particularly valuable when studying behaviors that cannot be ethically or practically manipulated, allowing researchers to gain insights from a distance.
One common technique is participant observation, where the researcher becomes part of the setting, closely observing behaviors and interactions while recording data discreetly. This method provides a rich, detailed context often missing in surveys or controlled experiments but requires ethical considerations regarding the researcher’s role and influence.
Another approach is non-participant observation, where researchers observe subjects without directly engaging. This method is beneficial for minimizing interference with the observed behaviors. Both techniques, while powerful, come with their own sets of limitations, such as observer bias or the lack of control over external variables.
The choice of data collection methods is paramount for quality insights; adequate planning and foresight can make the difference between meaningful conclusions and ambiguous results.
Ensuring Data Integrity
Ensuring data integrity is a linchpin in the world of data analysis, and it takes center stage in this narrative. It's not just a checkbox we tick off; rather, it’s foundational to the credibility of any research or analysis we undertake. When we talk about data integrity, we're really diving into the reliability and accuracy of the data we’ve collected. This can influence the outcomes of our research and affect the already tight-knit tapestry of knowledge and decisions that rely on this data.
When we’re confident in our data integrity, it shines through in our findings. This builds trust among stakeholders, from researchers to policymakers, making them more likely to act on our conclusions. Moreover, there are several considerations we need to keep in mind:
- Data Consistency: Maintaining consistency across datasets is essential. A discrepancy can lead to questioning the entire framework of your study.
- Completeness: Incomplete data can skew results. Imagine wanting to gauge public opinion but failing to include certain demographics; the insights gained would be limited and potentially misleading.
- Accuracy: If you’re off by even a small percentage, it can lead to significant misinterpretations in broader findings. Accuracy is the lifeblood of any analysis.
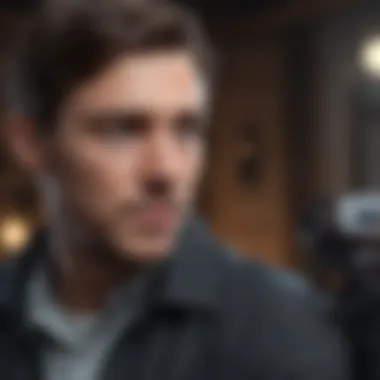
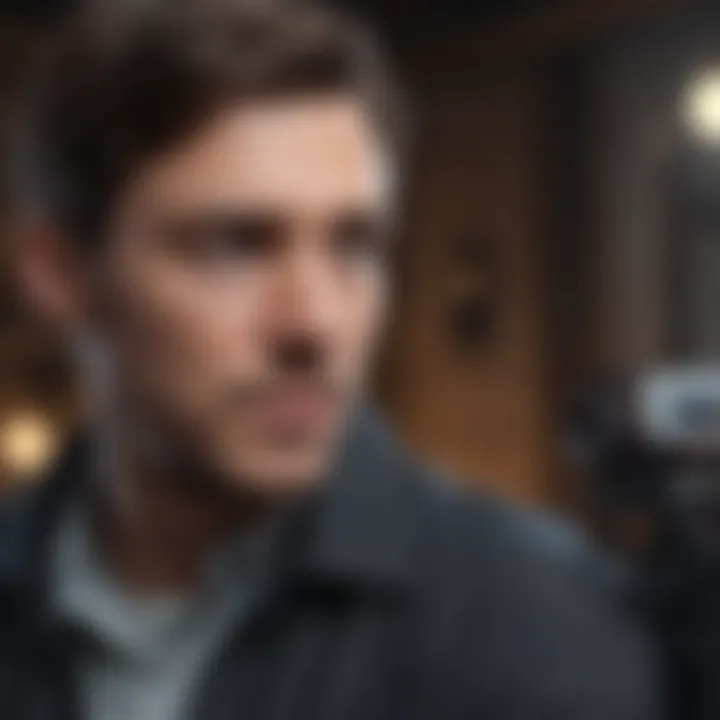
By addressing these facets of data integrity, we can better prepare ourselves to face the more complex hurdles of analysis. After all, if the data isn’t rock-solid, then what are we really standing on?
Data Validation Processes
In the realm of data analysis, data validation is a critical process that helps confirm the authenticity and quality of the data we use. It's like double-checking your math homework before turning it in. By employing systematic validation processes, we can catch errors early, saving time and resources down the line.
Common techniques for ensuring data validation include:
- Range Checks: Ensuring values fall within a specified range can prevent outliers that may not make sense contextually.
- Type Checks: Verifying that data types are correct, e.g., that numbers are indeed numbers, not strings masquerading as integers, can eliminate many headaches.
- Cross-Verification: This might involve comparing data collected from different sources or timeframes to spot inconsistencies.
The benefits of robust data validation are manifold. Firstly, it fosters confidence in conclusions drawn from the analysis. Secondly, it enhances the integrity of the analysis process itself, allowing for a smoother workflow and effective decision-making processes.
Identifying and Mitigating Bias
Bias can be a sneaky adversary in data analysis, lurking in the shadows and waiting for an opportune moment to distort findings. Identifying and addressing bias isn't just a recommended good practice; it’s crucial in ensuring that the results we report are truly reflective of reality.
Consider the following potential sources of bias:
- Selection Bias: When the sample isn’t representative of the population, it can lead to skewed results. A classic example is polling only individuals who have smartphones, ignoring those without.
- Confirmation Bias: This occurs when analysts favor information that confirms their preconceived notions while discounting contradictory evidence. It’s akin to playing favorites in a sports team.
- Measurement Bias: Flaws in how data is collected can happen too. If a survey unintentionally steers participants toward a particular answer, the result is a skewed representation of opinion.
To tackle bias, researchers can adopt several strategies. Using stratified sampling methods ensures diverse representation across demographics. Implementing blind studies can also reduce the influence of researchers' expectations on outcomes. The goal is clarity; when bias is tackled head-on, we pave the way for more reliable and authentic insights.
"The mischief of bias isn’t always obvious, but its impact can ripple through findings and decisions like a stone cast into still waters."
In sum, maintaining data integrity requires vigilance and deliberate strategies, from validation processes to countering biases. With these elements firmly in place, one can navigate the intricate landscape of data analysis with greater assurance.
Data Analysis Techniques
Understanding data analysis techniques is vital in the realm of research, as they provide structure to the otherwise chaotic nature of raw data. The techniques help researchers sift through the noise, extracting valuable insights that contribute to informed decision-making. By applying these methods, one can draw conclusions that are not just speculations but based on systematic numeric evidence. As such, it plays a cornerstone role in the process of transforming data into knowledge.
Descriptive Statistics
Descriptive statistics serve as the first step in statistical analysis. They summarize the key features of a dataset, providing a simple but effective overview. This simplicity is their strength, as they allow researchers to grasp the essence of their data without being overwhelmed by complex computations.
Measures of Central Tendency
Measures of Central Tendency encompass common statistical measures such as mean, median, and mode. They give insight into the average or typical values within a dataset. This has significant importance in understanding general trends without diving deep into individual data points.
The key characteristic of these measures is their ability to condense vast amounts of data into a single value that represents a central point. When you think about it, having a reliable central figure makes it easy to compare different datasets and pin down variations or patterns.
However, they come with their own quirks. For instance, the mean, while popular, can be highly swayed by outliers. A single unusually high or low number can distort the overall picture. In contrast, the median offers a more robust alternative, especially when dealing with skewed distributions. So, it's essential to choose wisely which measure to leverage depending on the dataset at hand.
Measures of Dispersion
Measures of Dispersion, including range, variance, and standard deviation, provide insight into the spread and variability of data points. Understanding how data points deviate from the central tendency is crucial for getting the full picture. While one might grasp the average, knowing how closely packed or widespread the data is adds depth to that comprehension.
The peak characteristic of measures of dispersion lies in their ability to reveal the variability in data. High dispersion signals inconsistency, while low dispersion suggests uniformity. This is particularly beneficial in fields where variability can influence decision-making processes significantly.
One unique feature of these measures is how they complement the measures of central tendency. For example, a dataset can have the same mean while exhibiting vastly different variances. Failing to consider dispersion while analyzing data may lead to misleading conclusions.
Inferential Statistics
Inferential statistics take researchers a step further. They allow one to make predictions or inferences about a population based on a sample. It's all about making educated guesses while quantifying the uncertainty attached to them.
Hypothesis Testing
Hypothesis Testing is at the heart of inferential statistics. It involves formulating a null hypothesis and an alternative hypothesis, allowing researchers to test assumptions using sample data. By determining whether to reject the null hypothesis, one can draw conclusions that extend beyond the sample.
The core characteristic here is its rigorous methodology. This gives hypothesis testing an edge as a systematic approach to testing claims and validating theories. The beauty lies in its reliance on probability, meaning one can quantify the likelihood of a hypothesis being true.
That said, hypothesis testing comes with some limitations. A common criticism is that it doesn't indicate the degree of truth but rather if the sample data supports the hypothesis. Thus, while it’s useful for validation, it may oversimplify complex scenarios.
Confidence Intervals
Confidence Intervals provide a range of values that likely include the true population parameter. They represent the degree of certainty around estimates derived from sample data, showcasing the reliability of statistical findings.
The defining trait of confidence intervals is their ability to encompass uncertainty. They enable researchers to communicate findings that acknowledge variability and risk, enhancing the transparency of research conclusions.
However, just like with hypothesis testing, confidence intervals have their nuances. The width of a confidence interval can indicate the level of precision. A wide interval suggests uncertainty, while a narrow one conveys strong confidence in the estimate. It's paramount to balance sample size and variability to produce meaningful intervals.
Multivariate Analysis
Moving deeper into the tapestry of data, Multivariate Analysis equips researchers with tools to understand relationships between multiple variables. Instead of zeroing in on just one factor, this analysis allows for a broader perspective.
Regression Analysis
Regression Analysis is a powerful multivariate technique that examines the relationships between a dependent variable and one or more independent variables. This method allows researchers to ascertain how changes in predictor variables influence outcomes.
Its most significant characteristic is its predictive capability. By establishing a mathematical relationship between variables, regression analysis aids in forecasting future trends based on historical data. This is invaluable across industries from finance to healthcare.
On the flip side, if the model isn't specified correctly, the conclusions drawn can be misleading. Also, it’s important to avoid assumptions about linearity unless justified, as real-world concerns can often tweak those relationships.
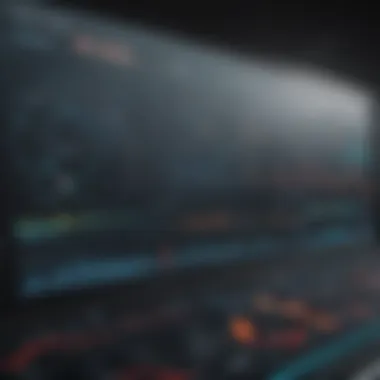
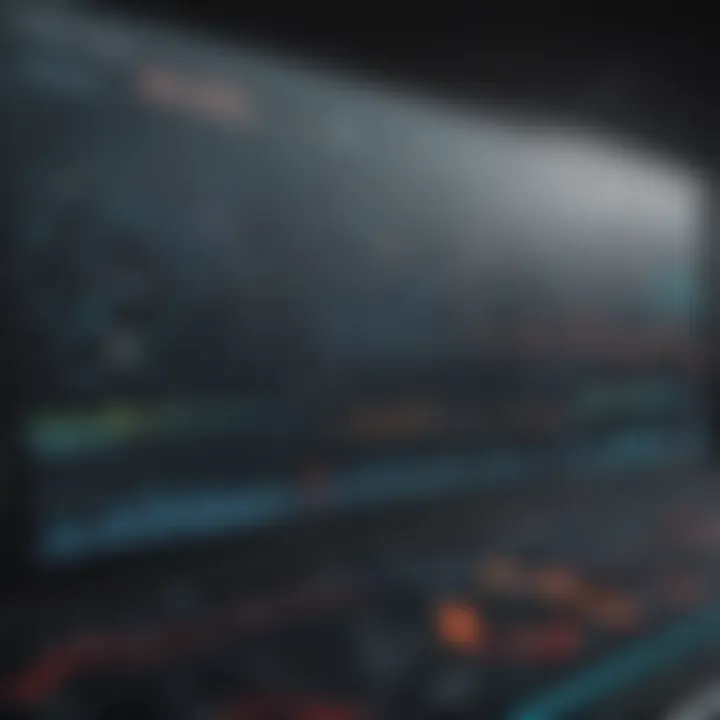
Factor Analysis
Factor Analysis simplifies complex data by identifying underlying relationships among variables. Through this method, researchers can cluster correlated variables, revealing the latent structures that might not be immediately obvious.
Its key feature is the ability to reduce data dimensionality, allowing teams to focus on significant factors while screening out noise. In cases with many variables, this becomes essential for efficient analysis.
However, the challenge lies in interpreting the factors correctly. Often, the clusters identified may not have clear real-world meaning or application without a thorough understanding of the data context, making interpretation a potentially tricky task.
Data analysis techniques enrich the process of understanding data, transforming raw numbers into narratives that inform decisions. From descriptive statistics showcasing basic tendencies to inferential statistics predicting implications and multivariate analyses revealing intricate relationships, each technique provides critical insights that are fundamental to robust research.
Visualizing Data
Visualizing data is akin to painting a vivid landscape with numbers; it takes the raw material of data and transforms it into meaningful insights. In today's age, where information overload is the norm, effective data visualization becomes crucial. It provides clarity, helping audiences to grasp complex concepts quickly. Moreover, it reveals patterns and trends that numbers alone might obscure. For instance, a well-designed chart has the power to convey information more instantly than pages of statistics. This underscores why mastering this skill is integral to conveying research findings understandably and impactfully.
Choosing the Right Visualization Tool
Graphs and Charts
Graphs and charts are a keystone in the realm of data visualization. They serve not only to present data in a more digestible format but also encourage engagement from viewers. The key characteristic of graphs and charts is their ability to summarize large volumes of data and allow for rapid comparison. They are a popular choice because they simplify complex information, making it immediately accessible to diverse audiences.
- Unique Feature: Graphs can depict changes over time, while charts can represent part-to-whole relationships effectively.
- Advantages: They help identify outliers and trends that might not be apparent in a table of numbers.
- Disadvantages: However, over-simplification can lead to misinterpretation, so careful selection of the right type of graph or chart is vital.
Infographics
Infographics take data visualization a step further by integrating graphics into storytelling. The specific aspect of infographics is their capacity to combine data, text, and visuals into an engaging narrative. The key characteristic that makes infographics a beneficial choice is their inherent ability to draw the viewer's eye, keeping them engaged while imparting information.
- Unique Feature: Infographics often blend statistics with design, making them not only informative but also aesthetically pleasing.
- Advantages: They cater to different learning styles, ensuring that both visual learners and those who prefer narratives can glean valuable insights.
- Disadvantages: On the flip side, if designed poorly, infographics can overwhelm rather than inform, so balance is crucial.
Best Practices for Data Visualization
To ensure that the visualization effectively communicates information, here are some best practices to keep in mind:
- Keep it Simple: Overly complex visualizations can confuse rather than clarify.
- Use Consistent Scales: In graphs, ensure that scales are uniform to avoid misleading interpretations.
- Color Wisely: Leverage color to highlight key aspects but avoid using too many hues that can distract.
- Test Your Visuals: Seek feedback from potential users to see if your visualization effectively communicates the intended message.
"Data is like a river; if you don’t manage it properly, it can flood and obscur the very truths it aims to show."
Communicating Data Effectively
Effective communication of data is crucial in ensuring that insights gleaned from research are not only understood but also actionable. In the current era, where information bombards us from all sides, presenting data in a clear and engaging manner can significantly influence decision-making processes in various fields, including education, healthcare, and business. Spotting trends, making sense of complex datasets, and telling a compelling story with numbers allows stakeholders to grasp essential findings quickly.
The Art of Storytelling with Data
Good data storytelling combines analysis with visual techniques and narrative elements. It’s about creating a connection with your audience that invites them to explore the underlying causes or implications of the data. This art is crucial because people remember stories much more than they remember plain statistics.
Contextualization
Contextualization stands out as a key element in data storytelling. It involves placing data within a relevant framework, which can help audiences grasp the significance of the findings. For example, if you are analyzing a city's pollution levels over time, comparing them to health statistics provides a real-world implication that numbers alone cannot.
The hallmark of contextualization is its ability to transform mere figures into relatable narratives. By providing background or situational context, one can evoke responses or connection from the audience.
However, one must tread carefully. An overly complicated context can muddy the waters, leading to potential misinterpretations. Striking a balance is essential. If successful, contextualization can enhance appreciation and understanding of the data presented, making it a favorable choice for any presentation.
Engagement Techniques
Engagement techniques are another pillar supporting the effective communication of data. Techniques such as interactive visuals, animations, or simply engaging layouts can capture audience attention better than static presentations. Audiences are more likely to absorb information presented in a dynamic manner.
A key characteristic of these techniques is their capacity to transform dry data into a memorable experience. For instance, using interactive graphs allows users to explore various aspects of the data at their own pace, leading to deeper understanding. This approach is especially popular in digital formats and has shown to increase retention rates significantly.
Yet, there can be drawbacks. Over-relying on gimmicks might distract from the core message of the data. The challenge lies in ensuring that while the method is engaging, it doesn't overshadow the substance of what the data is trying to convey.
Tailoring Your Message for Diverse Audiences
Adapting your message for different audiences is crucial for effective data communication. Not all stakeholders will have the same level of familiarity with the subject matter. Researchers might understand intricate statistical methods, while a business executive might need a straightforward summary.
The importance of considering the audience when presenting data cannot be overstated. Tailoring your message ensures that your findings resonate, making them more impactful. For instance, when speaking to a community group about health trends, using simple language and relatable examples will drive engagement and comprehension.
Challenges in Data Analysis
Data analysis, while an essential part of research, is not without its fair share of difficulties. The inherent complexity involved in analyzing large datasets—particularly when it involves incomplete or flawed data—presents significant challenges that must be navigated carefully. Understanding these challenges is crucial for anyone involved in data handling, be it students, researchers, or professionals, as they can have major implications on the validity of conclusions drawn from the data.
Dealing with Incomplete Data
Incomplete data is one of the primary obstacles analysts face. This can occur for a variety of reasons, such as missed responses in surveys or issues with data collection methods. The first step in handling incomplete data is identifying the extent of the chaos. Analysts often encounter two popular strategies: imputation and deletion.
- Imputation involves estimating missing values based on the available information. This can be done using models like mean substitution or more sophisticated methods like multiple imputation, which provide a bit more accuracy.
- Deletion, on the other hand, involves removing any incomplete data entries. While this may seem like a straightforward solution, it often leads to biased results or reduced statistical power.
Selecting the right approach largely depends on the dataset and the specific analysis being undertaken. The goal is always to retain as much valuable information as possible without sacrificing the integrity of the analysis itself.
Addressing Misinterpretations
Misinterpretations of data can lead to misguided conclusions. Sometimes it's not just about having the right tools but ensuring that the interpretations match the context of the data. Data visualizations, while useful, can sometimes be misleading if the data isn't represented appropriately. Here are crucial points to consider:
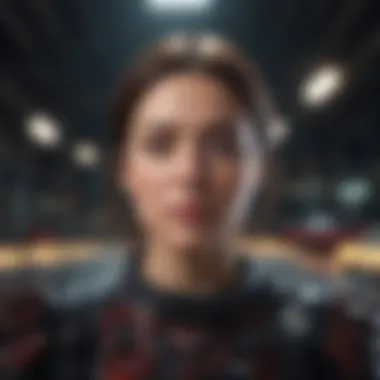
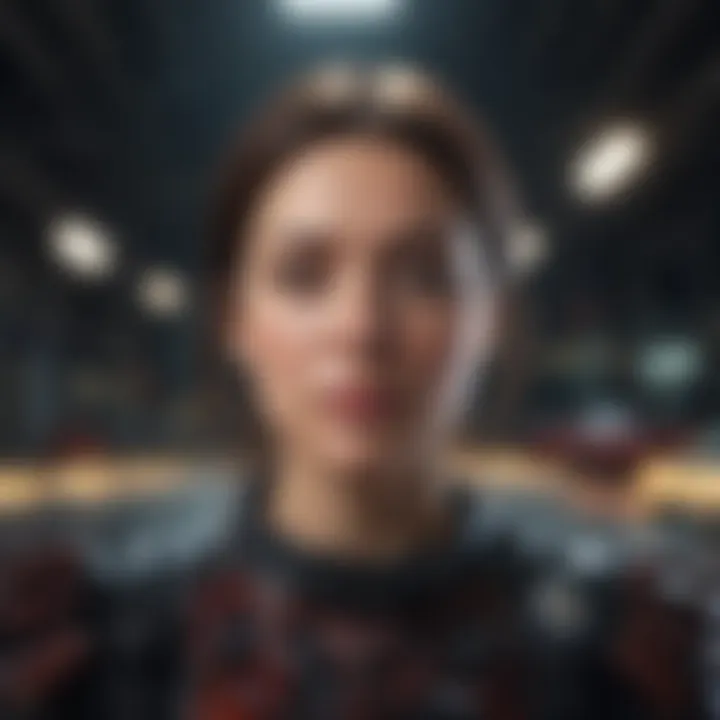
- Know Your Audience: Tailoring your message helps bridge the gap between technical details and comprehensibility. If a presentation is aimed at a non-technical audience, avoiding jargon and overly complicated visual representations can prevent misunderstandings.
- Context Matters: What might seem like a direct correlation could easily be a misconception without a clear understanding of underlying factors. Always question the narrative that the data supports.
- Use Robust Statistical Techniques: Applying the correct statistical tests is paramount. Misapplication of methods could invalidate conclusions and affect results adversely.
"Data may be the new oil, but it sure isn't the new gold without the right analytical process to refine it."
In short, being vigilant about misinterpretations can save countless hours of future work and erroneous direction. This vigilance enhances the overall conversation about analytics and opens doors to richer insights, providing a more precise foothold for strategic decision-making.
By addressing these challenges, analysts not only improve their immediate outcomes but also contribute to the larger conversation around data analysis practices. In the world of data, every detail counts, and a proactive approach is essential.
Ethics in Data Handling
In the world of data analysis and presentation, ethics plays a pivotal role in shaping how information is gathered, interpreted, and shared. The importance of ethical considerations in data handling cannot be overstated, especially as our environment becomes increasingly data-centric. It’s not just about numbers; it’s about the lives and experiences those numbers represent. When researchers, students, and professionals understand and apply ethical principles, they bolster the credibility of their work while protecting the individuals and communities involved.
Ethical data handling encompasses several critical facets:
- Respect for Participants: In any research involving human subjects, ensuring informed consent is paramount. Participants should be made fully aware of how their data will be used, with permission obtained without coercion. This promotes trust and enhances the integrity of the research process.
- Aligning with Legal Standards: Depending on the geographic location, various laws govern data usage. For instance, GDPR in Europe enforces strict regulations on personal data utilization. Adherence to these laws not only avoids legal ramifications but also cultivates ethical responsibility.
- Equality in Representation: When collecting data, it’s crucial to ensure diverse samples that accurately represent the entire population. This guards against biases that can skew results and lead to harmful misinterpretations.
By embedding ethical practices in data handling, one can protect the privacy of individuals and foster transparent data reporting.
Privacy Concerns and Data Protection
Privacy has become a buzzword in our digital age. As data breaches and identity theft become more common, the protection of personal information is more important than ever. Researchers must be particularly vigilant about safeguarding participants’ data to uphold their privacy rights.
- Data Anonymization: This technique is vital. By stripping personally identifiable information from datasets, researchers can still extract valuable insights without compromising individual identities. Techniques such as data masking or aggregating responses help maintain confidentiality.
- Secure Data Storage: Storing data in secure environments, whether on the cloud or hard drives, reduces the risk of unauthorized access. Using encryption protocols adds an additional layer of security.
- Regular Audits: Conducting periodic reviews and audits of data management practices helps identify any potential vulnerabilities. It’s better to be proactive than reactive when it comes to privacy.
"In a world where data is currency, protecting information is paramount to maintaining trust and integrity."
Emphasizing privacy concerns while handling data not only builds a reliable foundation for research but also ensures that public trust is sustained.
Transparency in Data Reporting
Transparency is an ethical cornerstone in data reporting that fosters confidence and encourages informed decision-making. When researchers share their methods and findings openly, they invite scrutiny and validation from peers and the wider community.
- Detailed Methodologies: Providing a clear outline of how data was collected and analyzed enables others to replicate your research. This builds a stronger case for the conclusions drawn and enhances the study's reliability.
- Sharing Limitations and Biases: No research is perfect. Recognizing and communicating the limitations of the study, including potential biases in data collection or analysis, adds credibility. It also helps stakeholders interpret findings more accurately.
- Open Data Initiatives: Encouraging open access to datasets and findings not only promotes collaboration but also allows others to build upon your work. Platforms like figshare or GitHub enable researchers to share their data widely.
When researchers prioritize transparency, they establish themselves as trustworthy voices in their field, promoting a culture of integrity and responsibility.
Future Trends in Data Analysis
As we forge further into the digital age, the landscape of data analysis is transforming rapidly. The emergence of new technologies and methodologies holds substantial promise for enhancing our understanding of complex information. The ability to sift through vast amounts of data efficiently is becoming increasingly pivotal for research, strategy formulation, and decision-making processes. Recognizing future trends isn’t just a passing thought; it’s a necessity to remain relevant in a data-driven world. This section examines two primary components set to shape the future of data analysis: Artificial Intelligence and Machine Learning, alongside the monumental role of Big Data.
Artificial Intelligence and Machine Learning
Artificial Intelligence (AI) and Machine Learning (ML) are undoubtedly at the forefront of modern data analysis transformations. These technologies process data at an astounding speed, allowing for deeper insights than traditional methods ever could. AI algorithms are capable of recognizing patterns, anomalies, and correlations within datasets that would otherwise go unnoticed by human analysts.
For instance, in healthcare, AI-driven diagnostics systems analyze patient data in real-time, significantly improving diagnostic accuracy and patient outcomes. Machine Learning, a subset of AI, improves its predictive abilities over time as it learns from new data. This ongoing refinement enables organizations to respond swiftly to trends and changes in customer behavior or market dynamics.
Here, we can summarize key advantages of integrating AI and ML in data analysis:
- Enhanced Efficiency: Processes that took hours can now be completed in minutes.
- Error Reduction: Automated systems reduce human error, leading to more reliable results.
- Predictive Insights: Companies can anticipate future trends based on data-driven predictions.
Incorporating AI and ML into data strategies offers organizations a competitive edge, making it essential for those looking to thrive in a data-centric environment.
The Role of Big Data
As we navigate through the sheer volume of data available today, Big Data becomes an essential player in the analysis arena. The term refers not merely to the size of the data sets, but also to the complexity and the speed at which they are generated. This vast supply of information can be overwhelming, but when harnessed properly, it can yield unparalleled insights.
Big Data is especially influential in personalization strategies across sectors. For example, e-commerce companies utilize vast troves of consumer data to tailor user experiences and enhance customer engagement. This hyper-personalization relies heavily on sophisticated data analytics tools that can process and interpret large datasets quickly.
Moreover, Big Data analysis provides the following benefits:
- Comprehensive Views: Analyzing extensive datasets offers a holistic picture of trends and patterns.
- Improved Decision Making: Organizations can make better-informed decisions based on insights drawn from the data.
- Innovation Opportunities: New patterns and trends can spark innovative approaches to products and services.
"The capacity to analyze Big Data will unlock opportunities that have remained otherwise hidden in the past."
By understanding and embracing these developments, students, researchers, and professionals can enhance their analytical skills and pave the way for a more informed future.
Closure
In the ever-evolving landscape of research, context, and decision-making, concluding and summing up the findings holds paramount importance. The conclusion serves as the spine of a comprehensive narrative, drawing connections between the data collected and the implications of that data for future action or understanding. By summarizing key insights effectively, researchers can ensure that their findings resonate and remain relevant.
Summarizing Key Insights
Differentiating between insights derived from data analysis is essential in presenting a coherent picture. Summarization acts as the thread that elucidates the intricate tapestry made from raw data. The main characteristics of this summarization phase include the ability to distill complex findings into straightforward, digestible conclusions. Such clarity is particularly beneficial for those who may not be intimately familiar with the intricacies of the data analysis but have a stake in the outcomes.
Final Thoughts on Data Analysis
The final thoughts encapsulate the essence of data analysis, steering focus towards its critical role in research and decision-making. This aspect of wrapping up analysis emphasizes the habit of reflection. By engaging in deep consideration of findings and implications, specialists can bolster the understanding about the data presented. This practice not only reinforces the data's significance but highlights patterns that may otherwise be overlooked.
Key characteristics of these final reflections include the acknowledgment of uncertainties and variables that might distort analysis. Embracing such sources of potential error, rather than avoiding them, can lead to a more robust understanding of the data landscape. Unique features of this discussion might involve recognizing evolving trends or patterns in larger contexts, which has the advantage of preparing researchers for adapting to future challenges in data representation and utilization.
Future Considerations in Data Presentation
As we look forward, the aspect of considering how data is presented becomes increasingly vital. This discussion is crucial in informing how audiences perceive and utilize data. Harnessing emerging technology, such as interactive dashboards or VR simulations, can revolutionize engagement. It pushes the boundaries of traditional methodologies, emphasizing accessibility for wider audiences.
The key characteristic of future considerations involves incorporating vast advancements in data visualization tools and techniques. These innovations foster a richer comprehension of data by transforming tedious statistics into lively narratives. A significant thread in these considerations is the pressing need for continuous adaptation and learning, as technological advancements create new horizons for data representation.
A consideration of unique features, such as personalization in data storytelling, allows for impactful connections between data and real-world scenarios. This certainly has its drawbacks: the risk of oversimplifying complex themes could undermine the data's integrity. Tailoring data presentations without diluting the information is a fine line to walk. Thus, while advancements in data presentation promise tremendous benefits, thoughtful application is essential to maintain credibility and depth in narrative-driven presentations.