Artificial Intelligence in Healthcare: Transformations and Implications
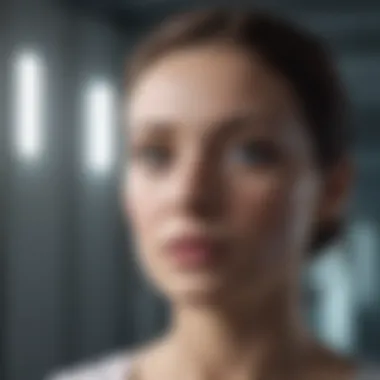
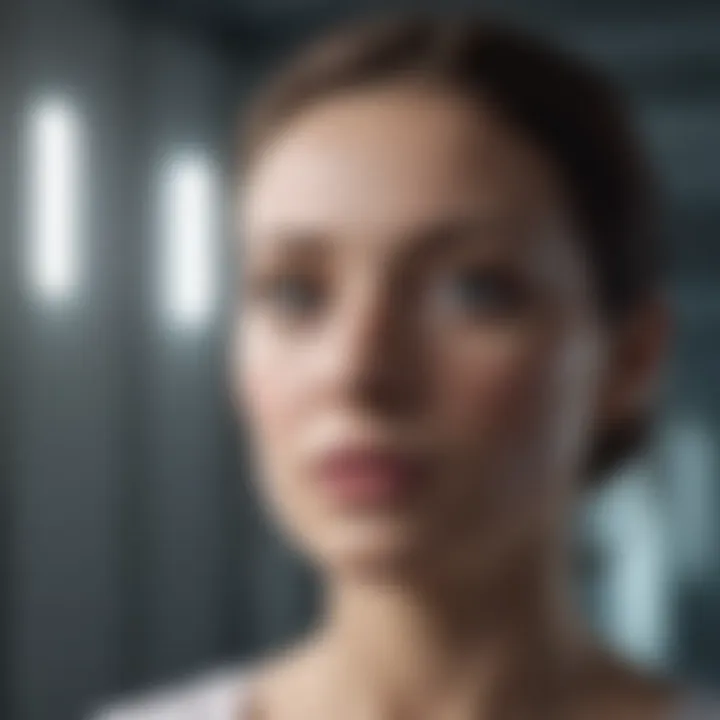
Overview of Research Topic
Brief Background and Context
Artificial intelligence (AI) has begun to influence various sectors significantly, and healthcare is no exception. From the early days of computer-assisted diagnosis to the current innovations in machine learning and natural language processing, the journey of AI in healthcare has been remarkable. The technology now aids professionals in making better-informed decisions by analyzing vast amounts of data that would be overwhelming for humans alone. These advances are not just theoretical; they have concrete applications in clinics, hospitals, and research laboratories.
Importance in Current Scientific Landscape
The relevance of AI in healthcare cannot be overstated. With rising healthcare costs, a growing patient population, and the demand for quick and accurate diagnostics, AI presents solutions that can streamline processes and enhance patient outcomes. In a field where precision is paramount, the ability of AI tools to identify patterns and predict outcomes can lead to earlier interventions and tailored treatment plans. This relevance resonates with students, researchers, educators, and professionals alike, pushing the boundaries of traditional practices and inviting further exploration of ethical considerations that accompany these technologies.
Methodology
Research Design and Approach
To analyze the transformations and implications of AI in healthcare, a mixed-methods approach can be effective. This involves both qualitative and quantitative research methodologies. Qualitative methods might include interviews with healthcare professionals who have implemented AI solutions, providing insights into real-world applications. On the other hand, quantitative analysis could involve examining data from clinical trials or healthcare databases to measure the efficacy of AI interventions.
Data Collection Techniques
Data collection for this study can be conducted through various techniques:
- Surveys targeting healthcare professionals to assess their experiences with AI tools.
- Interviews with AI developers and medical experts regarding the latest advancements.
- Case studies focusing on healthcare institutions that have successfully integrated AI systems into their workflows.
- Literature reviews of existing studies on AI applications in diagnostics and treatment decisions.
In employing these techniques, the analysis will cover both the promise and the challenges associated with implementing AI technologies in healthcare settings. By dedicating an entire research framework to this subject, the narrative formed will emphasize the impact of AI on patient care, diagnostics, medical research, and the ethical considerations that emerge from this intersection of technology and human factors.
Prelims to Artificial Intelligence in Healthcare
Artificial Intelligence (AI) has rapidly emerged as a pivotal force in healthcare, revolutionizing various facets of patient care, diagnostics, and research. It offers significant advantages, such as improving diagnostic accuracy, personalizing treatments, and enhancing operational efficiencies. Understanding AI's role within this context is essential for students, researchers, educators, and professionals aiming to grasp the intricacies of modern healthcare systems.
AI encompasses a wide range of technologies designed to simulate human intelligence. These include machine learning, natural language processing, and robotics. Such technologies help in processing vast datasets, leading to more informed medical decisions. This efficiency enables healthcare providers to achieve better outcomes, improve patient experiences, and reduce operational costs. Additionally, AI assists in addressing the challenges faced by the healthcare sector, such as physician shortages and increasing patient loads.
However, integrating AI into healthcare also presents challenges. Issues related to data privacy, security, and algorithmic bias raise critical questions about the implementation of these technologies. Understanding the holistic impact of AI in healthcare necessitates a deep dive into its definitions, historical context, and current applications. It also involves examining its benefits, ethical implications, and future prospects.
Through this exploration, we will unpack how AI is not just reshaping healthcare but is also an integral part of the evolution of medical practices and patient services. The implications are profound and will influence how future advancements are guided by both technological progress and ethical considerations.
Defining Artificial Intelligence
Artificial Intelligence refers to the capability of a machine to imitate intelligent human behavior. It involves the development of algorithms that enable machines to perform tasks typically requiring human intelligence, such as decision-making, problem-solving, and pattern recognition. In healthcare, AI can analyze complex data sets, recognize patterns in medical images, and support clinical decision-making.
The most common forms of AI in healthcare include machine learning and deep learning. Machine learning allows systems to improve their performance over time through experience, while deep learning mimics the functioning of the human brain to uncover intricate data patterns. Together, these technologies offer substantial potential for enhancing medical processes.
Historical Context of AI in Medicine
The journey of AI in medicine spans several decades, tracing back to its early conceptualization in the mid-20th century. Initial efforts largely focused on developing algorithms to process medical information. Early AI systems such as MYCIN, created in the 1970s, aimed to diagnose bacterial infections and recommend antibiotics based on patient data.
Fast forward to the 21st century; AI has matured significantly, driven by advancements in computing power and data availability. The introduction of electronic health records (EHR) has provided a rich foundation of data, making it possible for AI algorithms to learn and evolve. Today, AI applications range from enhancing imaging analysis to predictive analytics in patient outcomes, marking a transformational shift in how healthcare is delivered.
Current Applications of AI in Healthcare
Artificial intelligence (AI) is becoming critical in healthcare today. Its applications are transforming how medical professionals diagnose, treat, and manage patient care. Understanding current applications of AI allows stakeholders to see the potential benefits, such as improved patient outcomes and operational efficiencies. In this section, we will explore specific areas where AI is currently being utilized in the healthcare field, highlighting diagnostics, treatment personalization, and operational efficiency.
AI in Diagnostics
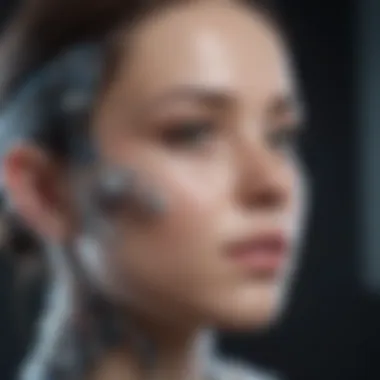
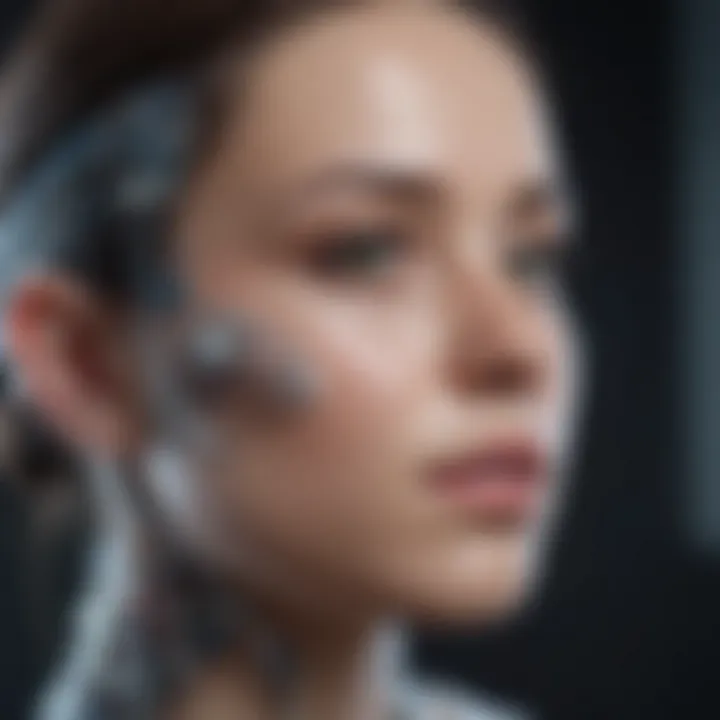
AI in diagnostics has revolutionized how diseases are detected and managed. By using advanced algorithms, AI systems can analyze large data sets much faster than human clinicians. This capability leads to early detection of various medical conditions, increasing the chances of successful treatments.
Image Recognition
Image recognition is a powerful tool in medical diagnostics. It allows AI systems to analyze medical images, such as X-rays and MRIs, with high precision. This technology excels in identifying patterns that may be missed by the human eye. One key characteristic of image recognition is its accuracy and speed, providing quicker results at a reduced cost for healthcare systems. A unique feature is its ability to improve over time through machine learning, which means it can become even more effective as it processes more images. However, reliance on technology can raise concerns over validation and the potential for misdiagnosis if the system is not properly trained.
Genomics
In genomics, AI analyses vast amounts of genetic data to offer insights into diseases at a molecular level. It helps identify gene mutations that may lead to specific health issues. The ability of AI to process complex genomic data makes it invaluable in personalized medicine and targeted therapies. Genomics is crucial for precision in understanding individual patient needs, making it a hot topic for research. One potential downside is that genomic data handling requires strict privacy protocols due to sensitive personal information. Maintaining security is a top consideration in this field.
Predictive Analytics
Predictive analytics uses historical data to forecast future health trends. This application is vital in anticipating disease outbreaks and patient needs. It makes healthcare more proactive rather than reactive. The key characteristic of predictive analytics is its ability to use existing data to make informed predictions. It provides healthcare professionals with valuable information regarding patient care pathways. A unique feature is its focus on population health, allowing institutions to allocate resources strategically. However, the reliance on historical data may not always accurately represent future risks.
AI in Treatment Personalization
Treatment personalization becomes increasingly vital in the healthcare landscape. With AI, treatments can be tailored to individual patients, maximizing effectiveness while minimizing side effects. This approach leads to better health outcomes and improved patient satisfaction.
Tailored Therapeutics
Tailored therapeutics involves customizing healthcare treatments based on individual genetic, environmental, and lifestyle factors. AI algorithms can analyze a myriad of data points to recommend the best treatment plans for patients. A critical aspect of tailored therapeutics is its impact on patient response and adherence to treatment. A unique feature is that it can lead to more efficient use of medications, potentially reducing waste and costs. Nevertheless, the complexity of these treatments may pose challenges, such as ensuring that healthcare providers are adequately trained to implement them.
Remote Monitoring
Remote monitoring systems leverage AI to track patients' health outside traditional clinical settings. This application plays a significant role in managing chronic diseases. Continuous monitoring allows for timely interventions, boosting patient safety. Key characteristics of remote monitoring include real-time data analysis and improved accessibility for patients. A unique feature is its ability to empower patients, encouraging them to take control of their health. However, some patients may lack the necessary devices or technology literacy required to utilize remote monitoring effectively.
AI in Operational Efficiency
Operational efficiency is a critical component for healthcare organizations. AI can streamline various processes, leading to reduced costs and improved resource allocation. The capabilities of AI to handle repetitive tasks allow healthcare professionals to focus on patient care rather than administrative duties.
Resource Allocation
Resource allocation is essential for optimizing healthcare delivery. AI systems can analyze data on patient flow and resource availability to make informed decisions on staff scheduling and equipment usage. A key characteristic of resource allocation platforms is their use of predictive modeling to forecast needs. This capability is particularly beneficial for hospitals facing fluctuating patient volumes. A unique feature is the potential for enhanced patient care through timely and appropriate resource distribution. On the downside, reliance on AI data may lead to difficulties if the input data is flawed or outdated.
Workflow Optimization
Workflow optimization involves using AI to improve the efficiency of healthcare processes. By automating routine tasks, AI can help reduce wait times and enhance patient interactions with healthcare systems. The key characteristic of workflow optimization tools is their ability to integrate disparate systems for seamless operation. This integration can lead to better communication among healthcare teams. A unique feature is that it can adapt and learn from operational data, optimizing workflows over time. However, one challenge is ensuring staff buy-in and training, as any implementation hurdles can impact patient care negatively.
Integration of AI in these applications signifies a turning point for the healthcare industry. By embracing these technologies, the potential for enhancing healthcare delivery and patient outcomes becomes increasingly tangible.
Benefits of AI Integration in Healthcare
The integration of artificial intelligence (AI) in healthcare brings a multitude of advantages. These benefits extend beyond mere automation, as they offer meaningful improvements in patient care, operational efficiency, and cost management. Understanding these aspects is crucial for stakeholders seeking to harness the full potential of AI technologies. This section delves into the key benefits of AI integration, focusing on enhancing patient outcomes, reducing costs, and increasing accessibility.
Enhancing Patient Outcomes
AI provides several tools that significantly enhance patient outcomes. One of the most impactful areas is in diagnostics. With the capabilities of machine learning algorithms, hospitals can analyze vast datasets quickly and accurately. For instance, image recognition technology can detect anomalies in X-rays and MRIs more efficiently than human radiologists. This leads to quicker diagnoses, allowing for timely interventions and improved patient prognoses.
Moreover, AI facilitates personalized treatment strategies. By analyzing data from genomic sequencing and patient histories, AI can help healthcare professionals design tailored treatment plans that are more effective for individual patients. This level of customization increases the chances of successful outcomes and reduces the risk of adverse reactions. Thus, the integration of AI not only enhances the quality of care but also optimizes the entire patient journey.
Reducing Costs
Implementing AI in healthcare can lead to significant cost reductions. One of the ways this happens is through increased operational efficiency. AI systems can predict patient admissions, ensuring that hospitals staff appropriately to meet demand without overextending resources. This predictive analytics capability helps in effective resource allocation, potentially reducing waste and lowering operational costs.
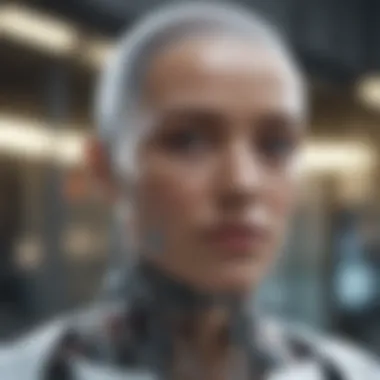
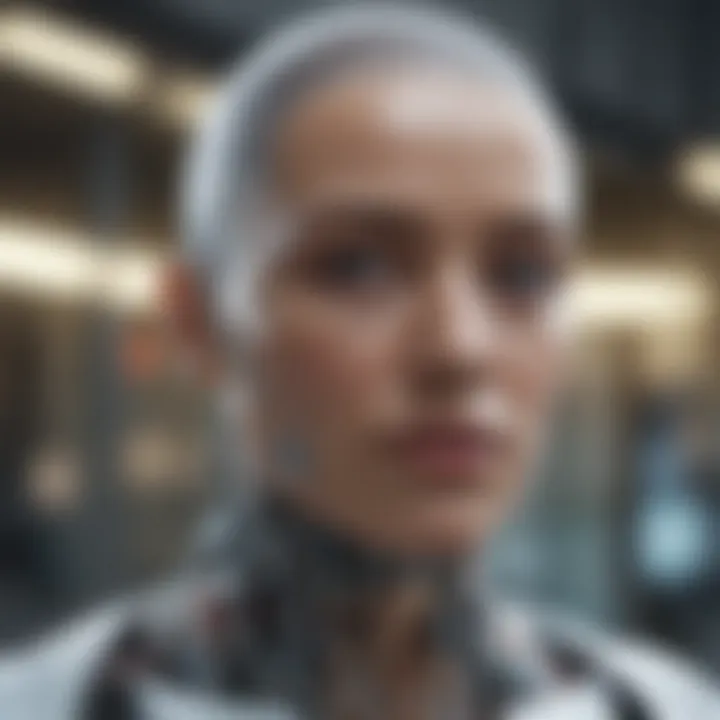
Furthermore, AI reduces the number of unnecessary tests and procedures. By providing accurate diagnostics, AI can ensure that only necessary treatments are pursued. For example, algorithms can analyze patient data to determine when further testing is essential, thus diminishing the costs associated with redundant medical procedures. Over time, these cost savings can be substantial, allowing healthcare providers to reallocate budgets toward services that directly benefit patients.
Increasing Accessibility
AI technology has the potential to increase healthcare accessibility significantly. Telemedicine, powered by AI, allows patients in rural or underserved areas to receive specialized medical care without traveling long distances. By leveraging virtual platforms, healthcare providers can extend their reach, offering consultations and follow-ups through video calls or chatbots.
Moreover, chatbots are enhancing patient engagement by providing 24/7 access to health information. Patients can obtain answers to their queries without waiting for business hours, thus improving patient satisfaction. These technologies also empower patients to manage their health conditions proactively, likely leading to better health outcomes.
In summary, AI integration into healthcare provides profound benefits that cannot be ignored. It enhances patient outcomes through improved diagnostics and personalized treatment, reduces costs via operational efficiency, and increases accessibility through telemedicine and digital resources. This unique convergence of technology and patient care holds the promise of transforming how healthcare is delivered.
Challenges in Implementing AI in Healthcare
The integration of artificial intelligence in healthcare presents significant opportunities and transformative potential. However, the path to effective implementation is fraught with various challenges. Understanding these challenges is crucial for stakeholders who aim to capitalize on AI technologies. Issues surrounding data privacy, system integration, and algorithmic bias come to the forefront in discussions about AI's adoption in healthcare. Addressing these challenges is essential not only for the functionality of AI applications but also for the ethical and equitable delivery of care.
Data Privacy and Security
Data privacy and security represent critical concerns in the adoption of AI in healthcare. The deployment of artificial intelligence systems often necessitates the collection, storage, and analysis of vast amounts of personal health information. This creates vulnerabilities, which malicious entities can exploit.
Healthcare organizations must comply with regulatory frameworks such as the Health Insurance Portability and Accountability Act (HIPAA) in the United States. These regulations govern how patient information is handled and shared. Breaches can lead to severe consequences, including legal penalties and loss of public trust, further complicating AI deployment.
Ensuring robust data protection measures is paramount. Organizations must invest in cybersecurity technologies such as encryption, firewalls, and intrusion detection systems. Employee training on data handling practices can also safeguard against internal risks. As healthcare providers adopt AI, balancing innovation with stringent data privacy standards will be vital.
Integration with Existing Systems
Integrating AI solutions into existing healthcare systems poses a unique set of challenges. Many healthcare institutions still rely on legacy systems that are not designed to accommodate the demands of modern AI technologies. This disconnect can hinder the seamless flow of information and could reduce the efficiency of healthcare delivery.
To achieve successful integration, it is necessary to assess the compatibility of new AI applications with current systems. This may involve upgrading existing IT infrastructures. Healthcare providers might also need to allocate resources for staff training. Change management is also essential to facilitate adoption among healthcare professionals who may be resistant to new technologies. By ensuring compatibility and effective training, organizations can improve the chances of successful AI implementation.
Bias and Fairness in Algorithms
Another pressing challenge in AI adoption in healthcare is bias and fairness in algorithms. The datasets utilized to train AI models may not be representative of the diverse patient populations. If the data lacks diversity, it can lead to biased outcomes that may adversely affect certain groups.
For instance, if an AI diagnostic tool is trained predominantly on data from a specific demographic, it may not perform adequately for individuals outside that demographic. This can exacerbate existing health disparities, compromising the very goal of enhanced patient care.
To mitigate bias, developers must use diverse datasets in training AI models. Continuous monitoring and updating of these models can help to evaluate performance across various patient profiles. Stakeholder engagement for feedback can lead to a fair and effective implementation.
If the underlying data is flawed or biased, the AI system's decisions and recommendations will also be flawed.
Addressing these challenges is crucial for the sustainable and ethical integration of AI in healthcare. Stakeholders, including policymakers, developers, and healthcare organizations, must collaborate to overcome these hurdles. Only then can the true potential of AI be realized in transforming healthcare delivery.
Ethical Considerations in AI Healthcare Applications
In the context of healthcare, the integration of artificial intelligence raises significant ethical questions. These considerations are essential for ensuring that AI technologies enhance, rather than complicate, the experience of patients and healthcare providers. It is crucial to explore how various ethical components, such as informed consent, accountability, and the impact on patient-provider relationships, shape the conversation about AI applications in this field.
Informed Consent
Informed consent is a cornerstone of ethical medical practice, and it becomes even more complex with the implementation of AI. Patients generally expect transparency and understanding when they engage with healthcare systems. With AI's involvement, ensuring that patients are aware of how their data will be used poses challenges. The complexity of algorithms and the potential for automated decisions can obscure this understanding.
Patients need clear communication about AI's role during diagnosis and treatment. Health professionals must articulate how AI assists in decision-making and the possible implications of that assistance. Without effective informed consent, patients risk feeling powerless, which could lead to mistrust in the healthcare system. Therefore, organizations must prioritize educational initiatives to support a deeper understanding of AI.
Accountability in AI Decisions
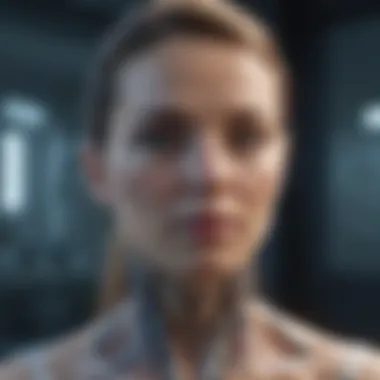
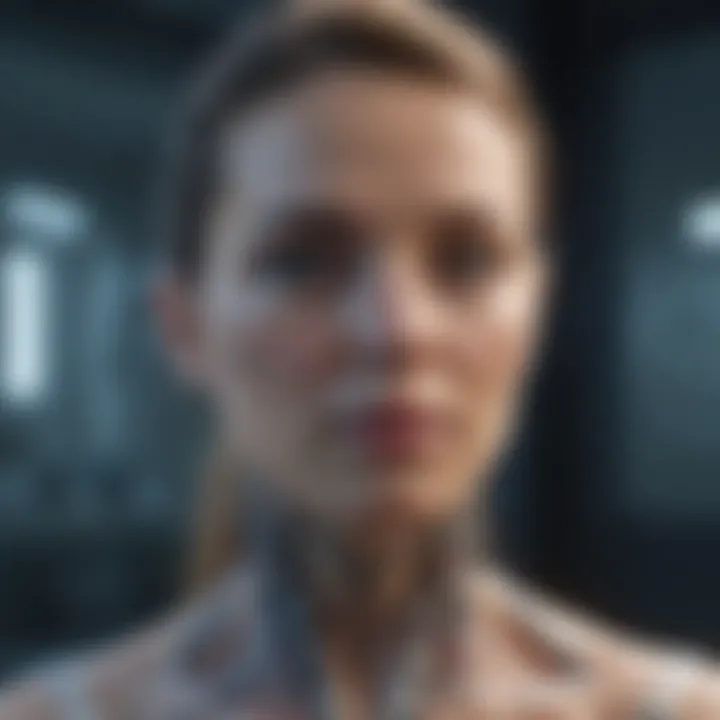
Accountability is another pressing issue. AI systems are designed to analyze vast amounts of data, but the challenge lies in determining who is responsible for the decisions made by these systems. If an AI makes a diagnostic error, does liability fall on the algorithm developers, healthcare providers, or the institution that employed them?
This ambiguity can deter healthcare professionals from fully embracing AI technologies. Clear guidelines on accountability must be established, requiring collaboration between developers, healthcare entities, and regulatory bodies. As AI continues to evolve, ongoing discussions about accountability will be necessary to maintain trust among all stakeholders involved in patient care.
Impact on Patient-Provider Relationships
AI applications have a profound effect on the dynamics of patient-provider relationships. Traditionally, these interactions are built on trust, empathy, and communication. However, the introduction of AI can make patients feel less connected to their caregivers. Automated systems may handle routine tasks, yet they lack the human touch that is vital in healthcare.
Patients may perceive AI as a substitute for their healthcare provider, which can lead to dissatisfaction in care. To mitigate this, it is important for practitioners to strike a balance between using AI tools and maintaining the personal element of patient care. Maintaining effective communication and ensuring that patients feel heard and valued is vital as AI systems become more integrated.
"The ethical landscape of AI in healthcare is not just a matter of technology, but fundamentally about preserving the humanity in care."
The Future of AI in Healthcare
The future of artificial intelligence in healthcare is a critical focus as it captures the transformative potential of technology in medicine. Understanding this evolution is essential for various stakeholders, including healthcare professionals, patients, and policymakers. AI is not only enhancing current practices but also paving the way for groundbreaking advancements that could significantly improve health outcomes in the years to come.
Innovations on the Horizon
Innovations in AI are set to revolutionize healthcare delivery. Technologies like machine learning and natural language processing are at the forefront, enabling faster and more accurate diagnoses. For instance, AI algorithms are now capable of analyzing vast amounts of clinical data that would take humans a much longer time to process. These innovations promise to lead to developments like:
- AI-driven virtual health assistants that provide real-time advice and symptom-checking for patients.
- Wearable health devices integrated with AI for continuous monitoring and feedback, improving patient engagement.
- Advanced predictive analytics that assess individual risk factors and suggest preventative measures tailored to personal health.
The implication of such innovations cannot be understated. They are not simply enhancements; they could become indispensable tools in everyday medical practice.
Potential for Global Health Improvements
The global implications of AI integration in healthcare are profound. AI can help bridge gaps in healthcare accessibility, particularly in underserved regions. By deploying AI solutions, organizations can:
- Expand telemedicine capabilities in remote areas, allowing patients to receive care without traveling substantial distances.
- Facilitate training and support for local health workers using AI systems that provide real-time consultations.
- Enhance epidemic response through predictive models that forecast disease spread, enabling proactive measures.
These factors suggest that AI has the potential to democratize healthcare access, improving overall health outcomes across diverse populations.
Regulatory and Policy Developments
As the influence of AI expands in healthcare, regulatory frameworks need to keep pace. Effective governance is essential to ensure that innovations are safe and ethical. Important considerations include:
- Establishing standards for AI algorithms to ensure their effectiveness and fairness in patient care.
- Creating transparent guidelines on how patient data is utilized, safeguarding privacy without hindering technological progress.
- Collaborating internationally to align regulations across borders, particularly since health issues often transcend geographical boundaries.
Regulatory environments that nurture innovation while protecting patient rights will be crucial. AI solutions must not only be effective but also trustworthy and ethically sound.
Epilogue
The conclusion serves as a critical component in synthesizing the insights gathered throughout the article. In exploring the role of artificial intelligence in healthcare, one must recognize its transformative potential in multiple areas including patient care, diagnostics, and operational efficiency. The implications of AI technologies resonate deeply within the healthcare landscape, highlighting both the opportunities and challenges that arise with adoption.
Summary of Key Insights
AI has demonstrated a capacity to enhance patient outcomes through improved diagnostics and tailored treatment options. For instance, image recognition systems can analyze medical images with high accuracy, potentially surpassing human capabilities in certain scenarios. Furthermore, AI facilitates the personalization of therapeutics, allowing healthcare providers to map treatment plans more effectively to individual patient needs. This approach not only improves the efficacy of interventions but may also streamline resource allocation in healthcare facilities.
Data privacy and security remain paramount concerns, as healthcare data is sensitive and requires stringent regulatory oversight. Similarly, the integration of AI into existing healthcare systems poses challenges that need strategic solutions. The potential for algorithmic bias continues to be a pressing issue, making the case for meticulous approaches to AI design and deployment.
Overall, the insights gathered suggest that while the future of AI in healthcare is promising, it must be approached with careful consideration of ethical principles, equity, and consent.
Call to Action for Stakeholders
Stakeholders play an essential role in the evolving landscape of AI in healthcare. The medical community, technologists, and policymakers need to collaborate closely to harness the full potential of AI while overcoming inherent challenges. There is a need for continued investment in research, education, and training to ensure that all parties understand AIβs capabilities and limitations.
Healthcare professionals should advocate for best practices in the use of AI tools to improve outcomes while safeguarding patient trust. Policymakers must also develop frameworks that encourage innovation yet prioritize patient safety and data privacy.
Only through a combined effort can we create an environment where AI serves as a powerful ally in the mission of advancing healthcare, ultimately leading to enhanced equity and improved health outcomes for all.